Closed GGGGxxxxxxxxr closed 1 year ago
Hi @morgolock , could you please check this issue? I just want to make sure there is nothing wrong with my settings and the benchmarking I have conducted.
Hi @GGGGxxxxxxxxr
I'd suggest you try ArmNN's ExecuteNetwork
to assess the performance of this model.
See below the inference time of ACL
root@acl_hikey_9:~/tmp/user/armnn/2302# LD_LIBRARY_PATH=.:$LD_LIBRARY_PATH ./ExecuteNetwork -c CpuAcc -m ../../tflite_models/wdsr_960.tflite -N --iterations=5 |grep Inference
Info: Inference time: 400.57 ms
Info: Inference time: 315.57 ms
Info: Inference time: 318.07 ms
Info: Inference time: 318.24 ms
Info: Inference time: 319.84 ms
Versus the time using tflite inference time
root@acl_hikey_9:~/tmp/user/armnn/2302# LD_LIBRARY_PATH=.:$LD_LIBRARY_PATH ./ExecuteNetwork -c CpuAcc -m ../../tflite_models/wdsr_960.tflite -N --iterations=5 -T tflite |grep Inference
Info: Inference time: 525.38 ms
Info: Inference time: 516.27 ms
Info: Inference time: 526.44 ms
Info: Inference time: 525.00 ms
Info: Inference time: 552.10 ms
In general, I would recommend using ExecuteNetwork
when you have a .tflite
model rather than implementing the model by hand using ACL operators.
Hope this helps.
Hi @morgolock,
Thanks for your advice!
I have tried the pre-built binary of ArmNN on my android devices, here is the command I used for benchmark: ./ExecuteNetwork -c CpuAcc -m /data/local/tmp/wdsr_960.tflite -N --iterations=10 --number-of-threads=1 This is my result:
With 1-thread, it takes about 240ms for this wads_960.tflite model execution.
I have tried the command with "-T" (enable TFLITE runtime), the result is worse than ArmNN. Here is the benchmark with "-T":
But that TFLITE Inferencing speed seems like quite unmatched with the result generated by the official benchmark tool from TFLITE.
Here is the link for TFLITE official benchmark: https://www.tensorflow.org/lite/performance/measurement
Thanks again!
Hi @GGGGxxxxxxxxr
But that TFLITE Inferencing speed seems like quite unmatched with the result generated by the official benchmark tool from TFLITE.
The difference you see may be because we are using different tflite versions. I used tflite v2.10, which version of tflite did you use? How big is the difference ?
Hi @morgolock
I have found out the cause of this issue.
In TensorflowLite, if XNNPACK has been enabled, the performance on CPU would be dramatically increased. If I disable XNNPACK in Tensorflowlite, the performance would be worse than ArmNN.
Seems like XNNPACK provides a super-optimized backend implementation for operators.
It would generate a speedup over 50%. From 220ms to 90ms with one-thread on the same model.
You could try TensorflowLite Benchmark with the TFLITE model, with _use_xnnpack= true / false.
You could tell the difference.
Thanks!
Hi @GGGGxxxxxxxxr
I tried with tflite benchmark tool and compared XNNPACK vs ArmNN Delegate
XNNPACK avg=67570.9 ArmNN avg=33329.7
The ArmNN delegate is two times faster than XNNPACK for this specific use case.
Please see below
$ LD_LIBRARY_PATH=./armnn/main/:$LD_LIBRARY_PATH ./linux_aarch64_benchmark_model --graph=./wdsr_960.tflite --num_threads=4 --num_runs=120 --warmup_runs=1
STARTING!
Log parameter values verbosely: [0]
Min num runs: [120]
Num threads: [4]
Min warmup runs: [1]
Graph: [./wdsr_960.tflite]
#threads used for CPU inference: [4]
Loaded model ./wdsr_960.tflite
INFO: Created TensorFlow Lite XNNPACK delegate for CPU.
The input model file size (MB): 0.011828
Initialized session in 7.739ms.
Running benchmark for at least 1 iterations and at least 0.5 seconds but terminate if exceeding 150 seconds.
count=13 first=89785 curr=35260 min=33983 max=89785 avg=39316.2 std=14580
Running benchmark for at least 120 iterations and at least 1 seconds but terminate if exceeding 150 seconds.
count=120 first=35154 curr=94375 min=34827 max=137872 avg=67570.9 std=28514
Inference timings in us: Init: 7739, First inference: 89785, Warmup (avg): 39316.2, Inference (avg): 67570.9
Note: as the benchmark tool itself affects memory footprint, the following is only APPROXIMATE to the actual memory footprint of the model at runtime. Take the information at your discretion.
Memory footprint delta from the start of the tool (MB): init=3.125 overall=387.75
And below ArmNN
$ LD_LIBRARY_PATH=./armnn/main/:$LD_LIBRARY_PATH ./linux_aarch64_benchmark_model --graph=./wdsr_960.tflite --num_threads=4 --num_runs=120 --warmup_runs=1 --external_delegate_path="armnn/main/libarmnnDelegate.so" --external_delegate_options="backends:CpuAcc"
STARTING!
Log parameter values verbosely: [0]
Min num runs: [120]
Num threads: [4]
Min warmup runs: [1]
Graph: [./wdsr_960.tflite]
#threads used for CPU inference: [4]
External delegate path: [armnn/main/libarmnnDelegate.so]
External delegate options: [backends:CpuAcc]
Loaded model ./wdsr_960.tflite
Can't load libOpenCL.so: libOpenCL.so: cannot open shared object file: No such file or directory
Can't load libGLES_mali.so: libGLES_mali.so: cannot open shared object file: No such file or directory
Can't load libmali.so: libmali.so: cannot open shared object file: No such file or directory
Couldn't find any OpenCL library.
INFO: TfLiteArmnnDelegate: Created TfLite ArmNN delegate.
EXTERNAL delegate created.
Explicitly applied EXTERNAL delegate, and the model graph will be completely executed by the delegate.
The input model file size (MB): 0.011828
Initialized session in 31.93ms.
Running benchmark for at least 1 iterations and at least 0.5 seconds but terminate if exceeding 150 seconds.
count=23 first=40037 curr=26812 min=18118 max=40037 avg=22013.8 std=4449
Running benchmark for at least 120 iterations and at least 1 seconds but terminate if exceeding 150 seconds.
count=120 first=25867 curr=37586 min=24979 max=46407 avg=33329.7 std=2946
Inference timings in us: Init: 31930, First inference: 40037, Warmup (avg): 22013.8, Inference (avg): 33329.7
Note: as the benchmark tool itself affects memory footprint, the following is only APPROXIMATE to the actual memory footprint of the model at runtime. Take the information at your discretion.
Memory footprint delta from the start of the tool (MB): init=74.5625 overall=404.5
Hope this helps
Hi,
I have just configured 540P WDSR Model (one block) with Neon-based Arm Compute Library with NHWC layout with F32 datatype.
I also benchmarked the same model structure based on TensorflowLite on the same Android device which I have used. The total execution time on ArmComputeLibrary (both one-thread) is 150ms Verus 91ms with TensorflowLite.
I have conducted the operator benchmarking in detail.
For example, the Convolution operation is (3, 960, 540) x (3,5,5,12) -> (12, 960, 540).
The average time cost for this operator on ACL is 71.45ms compared to 33.860 ms on TensorflowLite.
Here is my benchmark graph with --instruments=SCHEDULER_TIMER_MS
Here is TensorflowLite Benchmark for the exact same operator: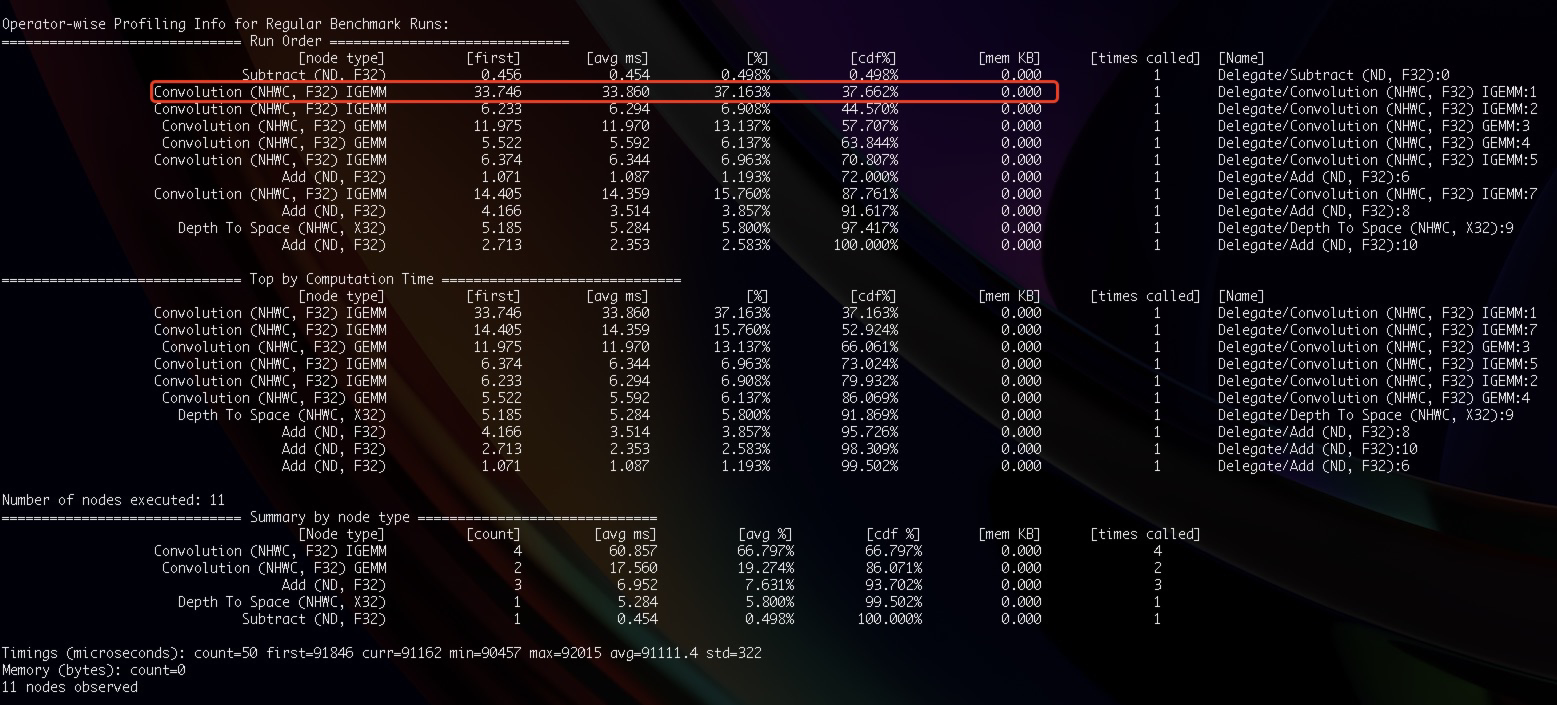
Here with the benchmark_model_code on ArmComputeLibrary for your reference:
The wdsr_540p_fp32.tflite file has been attached also if you want to benchmark the performance for TFLITE:
https://drive.google.com/file/d/1aL9sq8oDUKPu-lZqq5we7s7Vnf0enPnt/view?usp=sharing
Thanks for the patience!