Closed goktug97 closed 6 years ago
At 3036, didn't change.
3036: nan, nan avg loss, 0.001000 rate, 5.615964 seconds, 194304 images
Loaded: 0.000040 seconds
Region 82 Avg IOU: -nan, Class: -nan, Obj: -nan, No Obj: 0.000004, .5R: -nan, .75R: -nan, count: 0
Region 94 Avg IOU: -nan, Class: -nan, Obj: -nan, No Obj: 0.000001, .5R: -nan, .75R: -nan, count: 0
Region 106 Avg IOU: nan, Class: 0.000000, Obj: 0.000000, No Obj: 0.000000, .5R: 0.000000, .75R: 0.000000, count: 4
Region 82 Avg IOU: -nan, Class: -nan, Obj: -nan, No Obj: 0.000004, .5R: -nan, .75R: -nan, count: 0
Region 94 Avg IOU: -nan, Class: -nan, Obj: -nan, No Obj: 0.000001, .5R: -nan, .75R: -nan, count: 0
Region 106 Avg IOU: nan, Class: 0.000000, Obj: 0.000000, No Obj: 0.000000, .5R: 0.000000, .75R: 0.000000, count: 4
Region 82 Avg IOU: -nan, Class: -nan, Obj: -nan, No Obj: 0.000012, .5R: -nan, .75R: -nan, count: 0
Region 94 Avg IOU: -nan, Class: -nan, Obj: -nan, No Obj: 0.000006, .5R: -nan, .75R: -nan, count: 0
Region 106 Avg IOU: nan, Class: 0.000000, Obj: 0.000000, No Obj: 0.000000, .5R: 0.000000, .75R: 0.000000, count: 3
Region 82 Avg IOU: -nan, Class: -nan, Obj: -nan, No Obj: 0.000006, .5R: -nan, .75R: -nan, count: 0
Region 94 Avg IOU: -nan, Class: -nan, Obj: -nan, No Obj: 0.000003, .5R: -nan, .75R: -nan, count: 0
Region 106 Avg IOU: nan, Class: 0.000000, Obj: 0.000000, No Obj: 0.000000, .5R: 0.000000, .75R: 0.000000, count: 3
Region 82 Avg IOU: -nan, Class: -nan, Obj: -nan, No Obj: 0.000001, .5R: -nan, .75R: -nan, count: 0
Region 94 Avg IOU: -nan, Class: -nan, Obj: -nan, No Obj: 0.000002, .5R: -nan, .75R: -nan, count: 0
Region 106 Avg IOU: nan, Class: 0.000000, Obj: 0.000000, No Obj: 0.000000, .5R: 0.000000, .75R: 0.000000, count: 4
Region 82 Avg IOU: -nan, Class: -nan, Obj: -nan, No Obj: 0.000010, .5R: -nan, .75R: -nan, count: 0
Region 94 Avg IOU: -nan, Class: -nan, Obj: -nan, No Obj: 0.000000, .5R: -nan, .75R: -nan, count: 0
Region 106 Avg IOU: nan, Class: 0.000000, Obj: 0.000000, No Obj: 0.000000, .5R: 0.000000, .75R: 0.000000, count: 4
Region 82 Avg IOU: -nan, Class: -nan, Obj: -nan, No Obj: 0.000001, .5R: -nan, .75R: -nan, count: 0
Region 94 Avg IOU: -nan, Class: -nan, Obj: -nan, No Obj: 0.000001, .5R: -nan, .75R: -nan, count: 0
Region 106 Avg IOU: nan, Class: 0.000000, Obj: 0.000000, No Obj: 0.000000, .5R: 0.000000, .75R: 0.000000, count: 4
Region 82 Avg IOU: 0.746903, Class: 0.853777, Obj: 0.002779, No Obj: 0.000176, .5R: 1.000000, .75R: 0.500000, count: 2
Region 94 Avg IOU: -nan, Class: -nan, Obj: -nan, No Obj: 0.000011, .5R: -nan, .75R: -nan, count: 0
Region 106 Avg IOU: nan, Class: 0.000000, Obj: 0.000000, No Obj: 0.000000, .5R: 0.000000, .75R: 0.000000, count: 2
Region 82 Avg IOU: 0.902194, Class: 0.842486, Obj: 0.011181, No Obj: 0.000146, .5R: 1.000000, .75R: 1.000000, count: 1
Region 94 Avg IOU: -nan, Class: -nan, Obj: -nan, No Obj: 0.000018, .5R: -nan, .75R: -nan, count: 0
Region 106 Avg IOU: nan, Class: 0.000000, Obj: 0.000000, No Obj: 0.000000, .5R: 0.000000, .75R: 0.000000, count: 2
Region 82 Avg IOU: -nan, Class: -nan, Obj: -nan, No Obj: 0.000017, .5R: -nan, .75R: -nan, count: 0
Region 94 Avg IOU: -nan, Class: -nan, Obj: -nan, No Obj: 0.000002, .5R: -nan, .75R: -nan, count: 0
Region 106 Avg IOU: nan, Class: 0.000000, Obj: 0.000000, No Obj: 0.000000, .5R: 0.000000, .75R: 0.000000, count: 4
Region 82 Avg IOU: 0.832507, Class: 0.876532, Obj: 0.017637, No Obj: 0.000262, .5R: 1.000000, .75R: 1.000000, count: 1
Region 94 Avg IOU: -nan, Class: -nan, Obj: -nan, No Obj: 0.000028, .5R: -nan, .75R: -nan, count: 0
Region 106 Avg IOU: nan, Class: 0.000000, Obj: 0.000000, No Obj: 0.000000, .5R: 0.000000, .75R: 0.000000, count: 3
Region 82 Avg IOU: -nan, Class: -nan, Obj: -nan, No Obj: 0.000079, .5R: -nan, .75R: -nan, count: 0
Region 94 Avg IOU: 0.688873, Class: 0.905555, Obj: 0.000875, No Obj: 0.000013, .5R: 1.000000, .75R: 0.000000, count: 1
Region 106 Avg IOU: nan, Class: 0.000000, Obj: 0.000000, No Obj: 0.000000, .5R: 0.000000, .75R: 0.000000, count: 2
Region 82 Avg IOU: -nan, Class: -nan, Obj: -nan, No Obj: 0.000046, .5R: -nan, .75R: -nan, count: 0
Region 94 Avg IOU: 0.402090, Class: 0.934046, Obj: 0.018876, No Obj: 0.000043, .5R: 0.000000, .75R: 0.000000, count: 1
Region 106 Avg IOU: nan, Class: 0.000000, Obj: 0.000000, No Obj: 0.000000, .5R: 0.000000, .75R: 0.000000, count: 3
Region 82 Avg IOU: -nan, Class: -nan, Obj: -nan, No Obj: 0.000050, .5R: -nan, .75R: -nan, count: 0
Region 94 Avg IOU: 0.883075, Class: 0.884594, Obj: 0.002198, No Obj: 0.000034, .5R: 1.000000, .75R: 1.000000, count: 1
Region 106 Avg IOU: nan, Class: 0.000000, Obj: 0.000000, No Obj: 0.000000, .5R: 0.000000, .75R: 0.000000, count: 3
Region 82 Avg IOU: -nan, Class: -nan, Obj: -nan, No Obj: 0.000005, .5R: -nan, .75R: -nan, count: 0
Region 94 Avg IOU: -nan, Class: -nan, Obj: -nan, No Obj: 0.000003, .5R: -nan, .75R: -nan, count: 0
Region 106 Avg IOU: nan, Class: 0.000000, Obj: 0.000000, No Obj: 0.000000, .5R: 0.000000, .75R: 0.000000, count: 3
Region 82 Avg IOU: -nan, Class: -nan, Obj: -nan, No Obj: 0.000029, .5R: -nan, .75R: -nan, count: 0
Region 94 Avg IOU: -nan, Class: -nan, Obj: -nan, No Obj: 0.000035, .5R: -nan, .75R: -nan, count: 0
Region 106 Avg IOU: nan, Class: 0.000000, Obj: 0.000000, No Obj: 0.000000, .5R: 0.000000, .75R: 0.000000, count: 3
./darknet detector calc_anchors data/obj.data -num_of_clusters 9 -width 608 -height 608 -show
I didn't want to train the model on my GPU again because it is too slow so I used google cloud VM to train the model but it didn't work as expected.
If you use CUDNN_HALF=1
- then for the best speed/accuracy you should train first 1000 - 3000 iterations with CUDNN_HALF=0
and then continue training with CUDNN_HALF=1 OPENCV=1
and flag-dont_show
at the end of training command
If you want to detect very small object, then try to do this: https://github.com/AlexeyAB/darknet#how-to-improve-object-detection
for training for small objects - set
layers = -1, 11
instead of https://github.com/AlexeyAB/darknet/blob/6390a5a2ab61a0bdf6f1a9a6b4a739c16b36e0d7/cfg/yolov3.cfg#L720 and setstride=4
instead of https://github.com/AlexeyAB/darknet/blob/6390a5a2ab61a0bdf6f1a9a6b4a739c16b36e0d7/cfg/yolov3.cfg#L717
About such image. When this image will be resized to the network size 608x608 - dron will be more smaller:
And dron will be visible as:
That I'm not sure that it is possible to see a Drone in this image even theoretically. Even if a human or super-AI will look at this image.
I didn't build OpenCV for VM and use my windows machine for anchors.
Do I need to build OpenCV because I've got an error while building so I didn't want to waste time to find fix. I only need to train and get weights.
These are results for the dataset I've showed you.
That I'm not sure that it is possible to see a Drone in this image even theoretically. Even if a human or super-AI will look at this image.
Yes, It looks bad, I've created a new dataset with much bigger drones.
These are the results.
If you use CUDNN_HALF=1 - then for the best speed/accuracy you should train first 1000 - 3000 iterations with CUDNN_HALF=0 and then continue training with CUDNN_HALF=1 OPENCV=1 and flag-dont_show at the end of training command
I wil try this with the new dataset. Do I need opencv?
Is it bad to have images like these.
Logos
If you want to detect very small object, then try to do this: https://github.com/AlexeyAB/darknet#how-to-improve-object-detection
I've already done this.
Thank you for the help.
I started training with much bigger drones, like I said normally it would became non at this point but it is going well.
I've rebuilt it with CUDNN_HALF = 0
and it works. The one thing that wasn't common with my windows version and ubuntu version was CUDDN_HALF. Should I continue with it or change it to 1 after 3000 like you said. I can't see any changes in speed.
6: 5899.664551, 5898.643555 avg loss, 0.000000 rate, 5.732894 seconds, 384 images
Loaded: 0.000060 seconds
Region 82 Avg IOU: -nan, Class: -nan, Obj: -nan, No Obj: 0.557182, .5R: -nan, .75R: -nan, count: 0
Region 94 Avg IOU: 0.130260, Class: 0.383568, Obj: 0.482870, No Obj: 0.471329, .5R: 0.000000, .75R: 0.000000, c
ount: 1
Region 106 Avg IOU: 0.390566, Class: 0.562950, Obj: 0.359051, No Obj: 0.467074, .5R: 0.333333, .75R: 0.000000,
count: 3
Region 82 Avg IOU: -nan, Class: -nan, Obj: -nan, No Obj: 0.556578, .5R: -nan, .75R: -nan, count: 0
Region 94 Avg IOU: 0.111792, Class: 0.498207, Obj: 0.711638, No Obj: 0.471154, .5R: 0.000000, .75R: 0.000000, c
ount: 1
Region 106 Avg IOU: 0.382998, Class: 0.569001, Obj: 0.485595, No Obj: 0.467052, .5R: 0.333333, .75R: 0.333333,
count: 3
Region 82 Avg IOU: -nan, Class: -nan, Obj: -nan, No Obj: 0.556900, .5R: -nan, .75R: -nan, count: 0
Region 94 Avg IOU: 0.086824, Class: 0.564675, Obj: 0.338456, No Obj: 0.471358, .5R: 0.000000, .75R: 0.000000, c
ount: 1
Region 106 Avg IOU: 0.154292, Class: 0.577180, Obj: 0.446211, No Obj: 0.467008, .5R: 0.000000, .75R: 0.000000,
count: 2
Region 82 Avg IOU: -nan, Class: -nan, Obj: -nan, No Obj: 0.557081, .5R: -nan, .75R: -nan, count: 0
Region 94 Avg IOU: 0.202661, Class: 0.324839, Obj: 0.486635, No Obj: 0.471716, .5R: 0.000000, .75R: 0.000000, c
ount: 2
Region 106 Avg IOU: 0.335819, Class: 0.359475, Obj: 0.642483, No Obj: 0.467151, .5R: 0.250000, .75R: 0.000000,
count: 4
Region 82 Avg IOU: -nan, Class: -nan, Obj: -nan, No Obj: 0.556892, .5R: -nan, .75R: -nan, count: 0
Region 94 Avg IOU: 0.211860, Class: 0.626750, Obj: 0.617791, No Obj: 0.471828, .5R: 0.000000, .75R: 0.000000, c
ount: 3
Region 106 Avg IOU: 0.563700, Class: 0.719501, Obj: 0.178580, No Obj: 0.467003, .5R: 1.000000, .75R: 0.000000,
count: 1
Region 82 Avg IOU: -nan, Class: -nan, Obj: -nan, No Obj: 0.557168, .5R: -nan, .75R: -nan, count: 0
Region 94 Avg IOU: -nan, Class: -nan, Obj: -nan, No Obj: 0.471210, .5R: -nan, .75R: -nan, count: 0
Region 106 Avg IOU: 0.265112, Class: 0.469433, Obj: 0.445617, No Obj: 0.467072, .5R: 0.000000, .75R: 0.000000,
count: 4
Region 82 Avg IOU: 0.176443, Class: 0.312570, Obj: 0.602239, No Obj: 0.556627, .5R: 0.000000, .75R: 0.000000, count: 1
Region 94 Avg IOU: -nan, Class: -nan, Obj: -nan, No Obj: 0.471511, .5R: -nan, .75R: -nan, count: 0
Region 106 Avg IOU: 0.181706, Class: 0.396029, Obj: 0.502014, No Obj: 0.466205, .5R: 0.000000, .75R: 0.000000, count: 3
Region 82 Avg IOU: -nan, Class: -nan, Obj: -nan, No Obj: 0.557170, .5R: -nan, .75R: -nan, count: 0
Region 94 Avg IOU: 0.344896, Class: 0.419448, Obj: 0.719096, No Obj: 0.471212, .5R: 0.000000, .75R: 0.000000, count: 1
Region 106 Avg IOU: 0.244341, Class: 0.524644, Obj: 0.485079, No Obj: 0.466318, .5R: 0.000000, .75R: 0.000000, count: 3
Region 82 Avg IOU: -nan, Class: -nan, Obj: -nan, No Obj: 0.556918, .5R: -nan, .75R: -nan, count: 0
Region 94 Avg IOU: -nan, Class: -nan, Obj: -nan, No Obj: 0.471648, .5R: -nan, .75R: -nan, count: 0
Region 106 Avg IOU: 0.203127, Class: 0.534341, Obj: 0.547834, No Obj: 0.467389, .5R: 0.000000, .75R: 0.000000, count: 4
Region 82 Avg IOU: -nan, Class: -nan, Obj: -nan, No Obj: 0.557419, .5R: -nan, .75R: -nan, count: 0
Region 94 Avg IOU: 0.569745, Class: 0.491684, Obj: 0.482270, No Obj: 0.471179, .5R: 0.500000, .75R: 0.500000, count: 2
Region 106 Avg IOU: 0.346134, Class: 0.401266, Obj: 0.520670, No Obj: 0.465822, .5R: 0.500000, .75R: 0.000000, count: 2
Region 82 Avg IOU: -nan, Class: -nan, Obj: -nan, No Obj: 0.557475, .5R: -nan, .75R: -nan, count: 0
Region 94 Avg IOU: 0.083109, Class: 0.320227, Obj: 0.354584, No Obj: 0.471512, .5R: 0.000000, .75R: 0.000000, count: 1
Region 106 Avg IOU: 0.277230, Class: 0.381261, Obj: 0.303037, No Obj: 0.466422, .5R: 0.000000, .75R: 0.000000, count: 2
Region 82 Avg IOU: -nan, Class: -nan, Obj: -nan, No Obj: 0.556725, .5R: -nan, .75R: -nan, count: 0
Region 94 Avg IOU: 0.404005, Class: 0.530693, Obj: 0.618832, No Obj: 0.470947, .5R: 0.500000, .75R: 0.000000, count: 2
Region 106 Avg IOU: 0.331175, Class: 0.531811, Obj: 0.606449, No Obj: 0.466144, .5R: 0.000000, .75R: 0.000000, count: 2
Region 82 Avg IOU: -nan, Class: -nan, Obj: -nan, No Obj: 0.556775, .5R: -nan, .75R: -nan, count: 0
Region 94 Avg IOU: 0.537542, Class: 0.767057, Obj: 0.276498, No Obj: 0.471460, .5R: 1.000000, .75R: 0.000000, count: 1
Region 106 Avg IOU: 0.404649, Class: 0.645795, Obj: 0.473926, No Obj: 0.467668, .5R: 0.333333, .75R: 0.000000, count: 3
Region 82 Avg IOU: 0.532005, Class: 0.540673, Obj: 0.643533, No Obj: 0.556684, .5R: 1.000000, .75R: 0.000000, count: 1
Region 94 Avg IOU: 0.505834, Class: 0.664497, Obj: 0.518416, No Obj: 0.471344, .5R: 1.000000, .75R: 0.000000, count: 1
Region 106 Avg IOU: 0.306487, Class: 0.437105, Obj: 0.532901, No Obj: 0.466744, .5R: 0.000000, .75R: 0.000000, count: 2
Region 82 Avg IOU: -nan, Class: -nan, Obj: -nan, No Obj: 0.557432, .5R: -nan, .75R: -nan, count: 0
Region 94 Avg IOU: 0.377603, Class: 0.608601, Obj: 0.480991, No Obj: 0.471223, .5R: 0.000000, .75R: 0.000000, count: 2
Region 106 Avg IOU: 0.144395, Class: 0.550716, Obj: 0.386943, No Obj: 0.466214, .5R: 0.000000, .75R: 0.000000, count: 2
Region 82 Avg IOU: -nan, Class: -nan, Obj: -nan, No Obj: 0.557108, .5R: -nan, .75R: -nan, count: 0
Region 94 Avg IOU: 0.219363, Class: 0.282838, Obj: 0.347349, No Obj: 0.471247, .5R: 0.000000, .75R: 0.000000, count: 1
Region 106 Avg IOU: 0.227574, Class: 0.390735, Obj: 0.400786, No Obj: 0.467213, .5R: 0.000000, .75R: 0.000000, count: 3
I've rebuilt it with CUDNN_HALF = 0 and it works. The one thing that wasn't common with my windows version and ubuntu version was CUDDN_HALF. Should I continue with it or change it to 1 after 3000 like you said. I can't see any changes in speed.
In the most cases, you will see speedup with CUDNN_HALF=1 only if you use OPENCV=1 too. So if you don't see any acceleration, then use CUDNN_HALF=0.
I've used your repo to train custom object detection. The dataset contains 360 labeled drone images. I've successfully trained the model on my GTX960 It took a while but I got nice results. The results on different background didn't satisfy me, so I gather more images by blending, transparent background drones and 1280x720 images. Before blending I crop drones to the right size so I can use their width and height to create bounding boxes. Rotated each drone so I can get more data. Populated background images by changing their brightness and hue so that drone number and background number is the same. Blended the drone to a background, created yolo file, flipped the image, changed the brightness and once again created yolo file. I did this for every drone (2 images per drone). I checked the results with yolo_mark and they were correct. I didn't want to train the model on my GPU again because it is too slow so I used google cloud VM to train the model but it didn't work as expected. To make sure it isn't about the dataset, I've tried with 360 sized version but It didn't work either. I asked my friend to try the big dataset on windows ( GTX 1050) and it worked normally.
I've also tested the dataset with python ( anchor calc didn't show any problem though)
Old dataset has different sized images New dataset only contains 1280x720 images Dataset = Old dataset + new dataset
Dataset size: 2100 Train dataset size = 1890
Example images from the new dataset ( merged image ), mark the area so you can see the drone it is kinda hard.
I can't send ss of the yolo_mark result because I am not on my computer right now.
0 0.834375 0.5930555555555556 0.078125 0.09444444444444444
This is the text file for the last one.From old dataset ( old dataset is very augmented)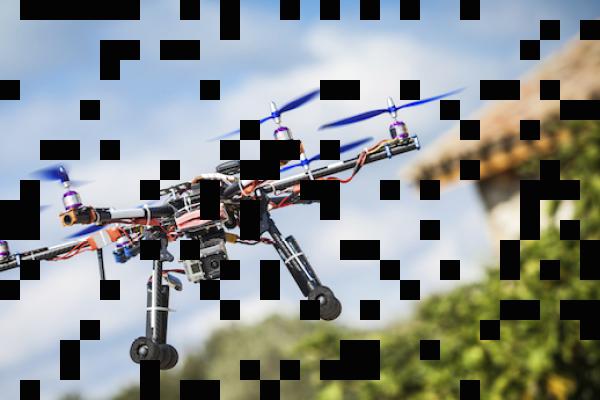
OS: Ubuntu 16.04 GPU: Tesla V100 CUDA Version: 9.1 (usr/local/cuda) Cudnn Version:7.0 (usr/local/cudnn) No OpenCV
Tried both default and calculated anchors.
~/darknet/data/obj : This is where I put my images and txts. ~/darknet/data : train.txt, test.txt, obj.data, and obj.names ~/darknet/ : yolo-obj.cfg
./darknet detector train data/obj.data yolo-obj.cfg darknet53.conv.74 First two epoch is normal At third epoch, Avg IOU becomes 0.00000 and results with inf loss After fourth epoch Avg IOU becomes nan most of the time and results with nan loss My results on Google Cloud Ubuntu
My friends results on windows 10
I couldn't find the problem everything seems normal.