Open MKiremitci opened 3 years ago
kiremitci@kiremitci:~/repos/yolov4_theaiguy/darknet$ nvcc --version ... What should i do?
If you use someone else's repo, then you should probably ask them.
If you want to use AlexeyAB's repo, then state exactly where you obtained the .cfg and .weights file you are attempting to use, and which version of darknet.
Lastly, drop by the Darknet/YOLO discord: https://discord.gg/zSq8rtW
I am really happy to take a help. Sorry about my question I am new on github, actually you are right. I will drop by discord, thanks for help.
My problem: Hi, I am new in this area and I want to use darknet and yolo on my linux. When i was enter command that below to terminal which is really beginner part of the controlling that darknet is installed or not. I am fallowing the process in this colab from my linux -> https://colab.research.google.com/drive/1_GdoqCJWXsChrOiY8sZMr_zbr_fH-0Fg?usp=sharing#scrollTo=jESyRhd4Nd38
Entered command: ./darknet detector test cfg/coco.data cfg/yolov4.cfg yolov4.weights data/person.jpg
Output of the command: CUDA-version: 9000 (10010), cuDNN: 7.4.1, CUDNN_HALF=1, GPU count: 1
CUDNN_HALF=1 OpenCV version: 3.4.4 0 : compute_capability = 500, cudnn_half = 0, GPU: GeForce GTX 950M net.optimized_memory = 0 mini_batch = 1, batch = 8, time_steps = 1, train = 0 layer filters size/strd(dil) input output 0 conv 32 3 x 3/ 1 608 x 608 x 3 -> 608 x 608 x 32 0.639 BF 1 conv 64 3 x 3/ 2 608 x 608 x 32 -> 304 x 304 x 64 3.407 BF 2 conv 64 1 x 1/ 1 304 x 304 x 64 -> 304 x 304 x 64 0.757 BF 3 route 1 -> 304 x 304 x 64 4 conv 64 1 x 1/ 1 304 x 304 x 64 -> 304 x 304 x 64 0.757 BF 5 conv 32 1 x 1/ 1 304 x 304 x 64 -> 304 x 304 x 32 0.379 BF 6 conv 64 3 x 3/ 1 304 x 304 x 32 -> 304 x 304 x 64 3.407 BF 7 Shortcut Layer: 4, wt = 0, wn = 0, outputs: 304 x 304 x 64 0.006 BF 8 conv 64 1 x 1/ 1 304 x 304 x 64 -> 304 x 304 x 64 0.757 BF 9 route 8 2 -> 304 x 304 x 128 10 conv 64 1 x 1/ 1 304 x 304 x 128 -> 304 x 304 x 64 1.514 BF 11 conv 128 3 x 3/ 2 304 x 304 x 64 -> 152 x 152 x 128 3.407 BF 12 conv 64 1 x 1/ 1 152 x 152 x 128 -> 152 x 152 x 64 0.379 BF 13 route 11 -> 152 x 152 x 128 14 conv 64 1 x 1/ 1 152 x 152 x 128 -> 152 x 152 x 64 0.379 BF 15 conv 64 1 x 1/ 1 152 x 152 x 64 -> 152 x 152 x 64 0.189 BF 16 conv 64 3 x 3/ 1 152 x 152 x 64 -> 152 x 152 x 64 1.703 BF 17 Shortcut Layer: 14, wt = 0, wn = 0, outputs: 152 x 152 x 64 0.001 BF 18 conv 64 1 x 1/ 1 152 x 152 x 64 -> 152 x 152 x 64 0.189 BF 19 conv 64 3 x 3/ 1 152 x 152 x 64 -> 152 x 152 x 64 1.703 BF 20 Shortcut Layer: 17, wt = 0, wn = 0, outputs: 152 x 152 x 64 0.001 BF 21 conv 64 1 x 1/ 1 152 x 152 x 64 -> 152 x 152 x 64 0.189 BF 22 route 21 12 -> 152 x 152 x 128 23 conv 128 1 x 1/ 1 152 x 152 x 128 -> 152 x 152 x 128 0.757 BF 24 conv 256 3 x 3/ 2 152 x 152 x 128 -> 76 x 76 x 256 3.407 BF 25 conv 128 1 x 1/ 1 76 x 76 x 256 -> 76 x 76 x 128 0.379 BF 26 route 24 -> 76 x 76 x 256 27 conv 128 1 x 1/ 1 76 x 76 x 256 -> 76 x 76 x 128 0.379 BF 28 conv 128 1 x 1/ 1 76 x 76 x 128 -> 76 x 76 x 128 0.189 BF 29 conv 128 3 x 3/ 1 76 x 76 x 128 -> 76 x 76 x 128 1.703 BF 30 Shortcut Layer: 27, wt = 0, wn = 0, outputs: 76 x 76 x 128 0.001 BF 31 conv 128 1 x 1/ 1 76 x 76 x 128 -> 76 x 76 x 128 0.189 BF 32 conv 128 3 x 3/ 1 76 x 76 x 128 -> 76 x 76 x 128 1.703 BF 33 Shortcut Layer: 30, wt = 0, wn = 0, outputs: 76 x 76 x 128 0.001 BF 34 conv 128 1 x 1/ 1 76 x 76 x 128 -> 76 x 76 x 128 0.189 BF 35 conv 128 3 x 3/ 1 76 x 76 x 128 -> 76 x 76 x 128 1.703 BF 36 Shortcut Layer: 33, wt = 0, wn = 0, outputs: 76 x 76 x 128 0.001 BF 37 conv 128 1 x 1/ 1 76 x 76 x 128 -> 76 x 76 x 128 0.189 BF 38 conv 128 3 x 3/ 1 76 x 76 x 128 -> 76 x 76 x 128 1.703 BF 39 Shortcut Layer: 36, wt = 0, wn = 0, outputs: 76 x 76 x 128 0.001 BF 40 conv 128 1 x 1/ 1 76 x 76 x 128 -> 76 x 76 x 128 0.189 BF 41 conv 128 3 x 3/ 1 76 x 76 x 128 -> 76 x 76 x 128 1.703 BF 42 Shortcut Layer: 39, wt = 0, wn = 0, outputs: 76 x 76 x 128 0.001 BF 43 conv 128 1 x 1/ 1 76 x 76 x 128 -> 76 x 76 x 128 0.189 BF 44 conv 128 3 x 3/ 1 76 x 76 x 128 -> 76 x 76 x 128 1.703 BF 45 Shortcut Layer: 42, wt = 0, wn = 0, outputs: 76 x 76 x 128 0.001 BF 46 conv 128 1 x 1/ 1 76 x 76 x 128 -> 76 x 76 x 128 0.189 BF 47 conv 128 3 x 3/ 1 76 x 76 x 128 -> 76 x 76 x 128 1.703 BF 48 Shortcut Layer: 45, wt = 0, wn = 0, outputs: 76 x 76 x 128 0.001 BF 49 conv 128 1 x 1/ 1 76 x 76 x 128 -> 76 x 76 x 128 0.189 BF 50 conv 128 3 x 3/ 1 76 x 76 x 128 -> 76 x 76 x 128 1.703 BF 51 Shortcut Layer: 48, wt = 0, wn = 0, outputs: 76 x 76 x 128 0.001 BF 52 conv 128 1 x 1/ 1 76 x 76 x 128 -> 76 x 76 x 128 0.189 BF 53 route 52 25 -> 76 x 76 x 256 54 conv 256 1 x 1/ 1 76 x 76 x 256 -> 76 x 76 x 256 0.757 BF 55 conv 512 3 x 3/ 2 76 x 76 x 256 -> 38 x 38 x 512 3.407 BF 56 conv 256 1 x 1/ 1 38 x 38 x 512 -> 38 x 38 x 256 0.379 BF 57 route 55 -> 38 x 38 x 512 58 conv 256 1 x 1/ 1 38 x 38 x 512 -> 38 x 38 x 256 0.379 BF 59 conv 256 1 x 1/ 1 38 x 38 x 256 -> 38 x 38 x 256 0.189 BF 60 conv 256 3 x 3/ 1 38 x 38 x 256 -> 38 x 38 x 256 1.703 BF 61 Shortcut Layer: 58, wt = 0, wn = 0, outputs: 38 x 38 x 256 0.000 BF 62 conv 256 1 x 1/ 1 38 x 38 x 256 -> 38 x 38 x 256 0.189 BF 63 conv 256 3 x 3/ 1 38 x 38 x 256 -> 38 x 38 x 256 1.703 BF 64 Shortcut Layer: 61, wt = 0, wn = 0, outputs: 38 x 38 x 256 0.000 BF 65 conv 256 1 x 1/ 1 38 x 38 x 256 -> 38 x 38 x 256 0.189 BF 66 conv 256 3 x 3/ 1 38 x 38 x 256 -> 38 x 38 x 256 1.703 BF 67 Shortcut Layer: 64, wt = 0, wn = 0, outputs: 38 x 38 x 256 0.000 BF 68 conv 256 1 x 1/ 1 38 x 38 x 256 -> 38 x 38 x 256 0.189 BF 69 conv 256 3 x 3/ 1 38 x 38 x 256 -> 38 x 38 x 256 1.703 BF 70 Shortcut Layer: 67, wt = 0, wn = 0, outputs: 38 x 38 x 256 0.000 BF 71 conv 256 1 x 1/ 1 38 x 38 x 256 -> 38 x 38 x 256 0.189 BF 72 conv 256 3 x 3/ 1 38 x 38 x 256 -> 38 x 38 x 256 1.703 BF 73 Shortcut Layer: 70, wt = 0, wn = 0, outputs: 38 x 38 x 256 0.000 BF 74 conv 256 1 x 1/ 1 38 x 38 x 256 -> 38 x 38 x 256 0.189 BF 75 conv 256 3 x 3/ 1 38 x 38 x 256 -> 38 x 38 x 256 1.703 BF 76 Shortcut Layer: 73, wt = 0, wn = 0, outputs: 38 x 38 x 256 0.000 BF 77 conv 256 1 x 1/ 1 38 x 38 x 256 -> 38 x 38 x 256 0.189 BF 78 conv 256 3 x 3/ 1 38 x 38 x 256 -> 38 x 38 x 256 1.703 BF 79 Shortcut Layer: 76, wt = 0, wn = 0, outputs: 38 x 38 x 256 0.000 BF 80 conv 256 1 x 1/ 1 38 x 38 x 256 -> 38 x 38 x 256 0.189 BF 81 conv 256 3 x 3/ 1 38 x 38 x 256 -> 38 x 38 x 256 1.703 BF 82 Shortcut Layer: 79, wt = 0, wn = 0, outputs: 38 x 38 x 256 0.000 BF 83 conv 256 1 x 1/ 1 38 x 38 x 256 -> 38 x 38 x 256 0.189 BF 84 route 83 56 -> 38 x 38 x 512 85 conv 512 1 x 1/ 1 38 x 38 x 512 -> 38 x 38 x 512 0.757 BF 86 conv 1024 3 x 3/ 2 38 x 38 x 512 -> 19 x 19 x1024 3.407 BF 87 conv 512 1 x 1/ 1 19 x 19 x1024 -> 19 x 19 x 512 0.379 BF 88 route 86 -> 19 x 19 x1024 89 conv 512 1 x 1/ 1 19 x 19 x1024 -> 19 x 19 x 512 0.379 BF 90 conv 512 1 x 1/ 1 19 x 19 x 512 -> 19 x 19 x 512 0.189 BF 91 conv 512 3 x 3/ 1 19 x 19 x 512 -> 19 x 19 x 512 1.703 BF 92 Shortcut Layer: 89, wt = 0, wn = 0, outputs: 19 x 19 x 512 0.000 BF 93 conv 512 1 x 1/ 1 19 x 19 x 512 -> 19 x 19 x 512 0.189 BF 94 conv 512 3 x 3/ 1 19 x 19 x 512 -> 19 x 19 x 512 1.703 BF 95 Shortcut Layer: 92, wt = 0, wn = 0, outputs: 19 x 19 x 512 0.000 BF 96 conv 512 1 x 1/ 1 19 x 19 x 512 -> 19 x 19 x 512 0.189 BF 97 conv 512 3 x 3/ 1 19 x 19 x 512 -> 19 x 19 x 512 1.703 BF 98 Shortcut Layer: 95, wt = 0, wn = 0, outputs: 19 x 19 x 512 0.000 BF 99 conv 512 1 x 1/ 1 19 x 19 x 512 -> 19 x 19 x 512 0.189 BF 100 conv 512 3 x 3/ 1 19 x 19 x 512 -> 19 x 19 x 512 1.703 BF 101 Shortcut Layer: 98, wt = 0, wn = 0, outputs: 19 x 19 x 512 0.000 BF 102 conv 512 1 x 1/ 1 19 x 19 x 512 -> 19 x 19 x 512 0.189 BF 103 route 102 87 -> 19 x 19 x1024 104 conv 1024 1 x 1/ 1 19 x 19 x1024 -> 19 x 19 x1024 0.757 BF 105 conv 512 1 x 1/ 1 19 x 19 x1024 -> 19 x 19 x 512 0.379 BF 106 conv 1024 3 x 3/ 1 19 x 19 x 512 -> 19 x 19 x1024 3.407 BF 107 conv 512 1 x 1/ 1 19 x 19 x1024 -> 19 x 19 x 512 0.379 BF 108 max 5x 5/ 1 19 x 19 x 512 -> 19 x 19 x 512 0.005 BF 109 route 107 -> 19 x 19 x 512 110 max 9x 9/ 1 19 x 19 x 512 -> 19 x 19 x 512 0.015 BF 111 route 107 -> 19 x 19 x 512 112 max 13x13/ 1 19 x 19 x 512 -> 19 x 19 x 512 0.031 BF 113 route 112 110 108 107 -> 19 x 19 x2048 114 conv 512 1 x 1/ 1 19 x 19 x2048 -> 19 x 19 x 512 0.757 BF 115 conv 1024 3 x 3/ 1 19 x 19 x 512 -> 19 x 19 x1024 3.407 BF 116 conv 512 1 x 1/ 1 19 x 19 x1024 -> 19 x 19 x 512 0.379 BF 117 conv 256 1 x 1/ 1 19 x 19 x 512 -> 19 x 19 x 256 0.095 BF 118 upsample 2x 19 x 19 x 256 -> 38 x 38 x 256 119 route 85 -> 38 x 38 x 512 120 conv 256 1 x 1/ 1 38 x 38 x 512 -> 38 x 38 x 256 0.379 BF 121 route 120 118 -> 38 x 38 x 512 122 conv 256 1 x 1/ 1 38 x 38 x 512 -> 38 x 38 x 256 0.379 BF 123 conv 512 3 x 3/ 1 38 x 38 x 256 -> 38 x 38 x 512 3.407 BF 124 conv 256 1 x 1/ 1 38 x 38 x 512 -> 38 x 38 x 256 0.379 BF 125 conv 512 3 x 3/ 1 38 x 38 x 256 -> 38 x 38 x 512 3.407 BF 126 conv 256 1 x 1/ 1 38 x 38 x 512 -> 38 x 38 x 256 0.379 BF 127 conv 128 1 x 1/ 1 38 x 38 x 256 -> 38 x 38 x 128 0.095 BF 128 upsample 2x 38 x 38 x 128 -> 76 x 76 x 128 129 route 54 -> 76 x 76 x 256 130 conv 128 1 x 1/ 1 76 x 76 x 256 -> 76 x 76 x 128 0.379 BF 131 route 130 128 -> 76 x 76 x 256 132 conv 128 1 x 1/ 1 76 x 76 x 256 -> 76 x 76 x 128 0.379 BF 133 conv 256 3 x 3/ 1 76 x 76 x 128 -> 76 x 76 x 256 3.407 BF 134 conv 128 1 x 1/ 1 76 x 76 x 256 -> 76 x 76 x 128 0.379 BF 135 conv 256 3 x 3/ 1 76 x 76 x 128 -> 76 x 76 x 256 3.407 BF 136 conv 128 1 x 1/ 1 76 x 76 x 256 -> 76 x 76 x 128 0.379 BF 137 conv 256 3 x 3/ 1 76 x 76 x 128 -> 76 x 76 x 256 3.407 BF 138 conv 255 1 x 1/ 1 76 x 76 x 256 -> 76 x 76 x 255 0.754 BF 139 yolo [yolo] params: iou loss: ciou (4), iou_norm: 0.07, obj_norm: 1.00, cls_norm: 1.00, delta_norm: 1.00, scale_x_y: 1.20 nms_kind: greedynms (1), beta = 0.600000 140 route 136 -> 76 x 76 x 128 141 conv 256 3 x 3/ 2 76 x 76 x 128 -> 38 x 38 x 256 0.852 BF 142 route 141 126 -> 38 x 38 x 512 143 conv 256 1 x 1/ 1 38 x 38 x 512 -> 38 x 38 x 256 0.379 BF 144 conv 512 3 x 3/ 1 38 x 38 x 256 -> 38 x 38 x 512 3.407 BF 145 conv 256 1 x 1/ 1 38 x 38 x 512 -> 38 x 38 x 256 0.379 BF 146 conv 512 3 x 3/ 1 38 x 38 x 256 -> 38 x 38 x 512 3.407 BF 147 conv 256 1 x 1/ 1 38 x 38 x 512 -> 38 x 38 x 256 0.379 BF 148 conv 512 3 x 3/ 1 38 x 38 x 256 -> 38 x 38 x 512 3.407 BF 149 conv 255 1 x 1/ 1 38 x 38 x 512 -> 38 x 38 x 255 0.377 BF 150 yolo [yolo] params: iou loss: ciou (4), iou_norm: 0.07, obj_norm: 1.00, cls_norm: 1.00, delta_norm: 1.00, scale_x_y: 1.10 nms_kind: greedynms (1), beta = 0.600000 151 route 147 -> 38 x 38 x 256 152 conv 512 3 x 3/ 2 38 x 38 x 256 -> 19 x 19 x 512 0.852 BF 153 route 152 116 -> 19 x 19 x1024 154 conv 512 1 x 1/ 1 19 x 19 x1024 -> 19 x 19 x 512 0.379 BF 155 conv 1024 3 x 3/ 1 19 x 19 x 512 -> 19 x 19 x1024 3.407 BF 156 conv 512 1 x 1/ 1 19 x 19 x1024 -> 19 x 19 x 512 0.379 BF 157 conv 1024 3 x 3/ 1 19 x 19 x 512 -> 19 x 19 x1024 3.407 BF 158 conv 512 1 x 1/ 1 19 x 19 x1024 -> 19 x 19 x 512 0.379 BF 159 conv 1024 3 x 3/ 1 19 x 19 x 512 -> 19 x 19 x1024 3.407 BF 160 conv 255 1 x 1/ 1 19 x 19 x1024 -> 19 x 19 x 255 0.189 BF 161 yolo [yolo] params: iou loss: ciou (4), iou_norm: 0.07, obj_norm: 1.00, cls_norm: 1.00, delta_norm: 1.00, scale_x_y: 1.05 nms_kind: greedynms (1), beta = 0.600000 Total BFLOPS 128.459 avg_outputs = 1068395 Allocate additional workspace_size = 52.43 MB Loading weights from yolov4.weights... seen 64, trained: 32032 K-images (500 Kilo-batches_64) Done! Loaded 106 layers from weights-file Detection layer: 139 - type = 28 Detection layer: 150 - type = 28 Detection layer: 161 - type = 28 data/person.jpg: Predicted in 331.207000 milli-seconds. person: 100% motorbike: 100% aeroplane: 100% bus: 100% train: 100% truck: 100% boat: 100% stop sign: 100% parking meter: 100% bench: 100% bird: 100% dog: 100% horse: 100% cow: 100% bear: 100% zebra: 100% backpack: 100% tie: 100% suitcase: 100% frisbee: 100% snowboard: 100% kite: 100% baseball glove: 100% surfboard: 100% fork: 100% . . ->>>>>> THIS PART IS LONG SO I DID NOT ENTER IT HERE. . kite: 100% baseball glove: 100% surfboard: 100% tennis racket: 100% wine glass: 100% fork: 100% banana: 100% apple: 100% pizza: 100% donut: 100% pottedplant: 100% diningtable: 100% tvmonitor: 100% laptop: 100% mouse: 100% cell phone: 100% microwave: 100% oven: 100% book: 100% scissors: 100%
The output image is: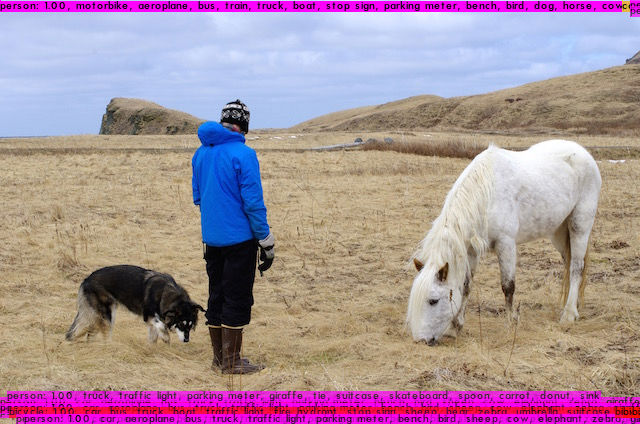
What i am tried: I deleted the folder then do the same things, but I've taken same outputs.
Can CUDA is a problem? I just want to say my cuda version is lower than the cuda version that said in YOLOv4 requirement (CUDA >= 10.0).
kiremitci@kiremitci:~/repos/yolov4_theaiguy/darknet$ nvcc --version nvcc: NVIDIA (R) Cuda compiler driver Copyright (c) 2005-2017 NVIDIA Corporation Built on Fri_Sep__1_21:08:03_CDT_2017 Cuda compilation tools, release 9.0, V9.0.176
What should i do?