Open beerzyp opened 3 years ago
Hey @beerzyp the plots in Example 2 and 3 seem symmetric because they are forced to by the model. The Cox proportional hazard relies on the proportional hazards assumption. This basically means that the ratio of the hazards are constant over time. If you were to plot the hazard ratio, it would look like a flat line over all Event_at
. Another way of saying this is that the hazard ratios are constant over time.
How that shows up in a survival plot is a little different because of the relationship between hazards and survival. The survival curves end up following the pattern exemplified in Example 2. There is some useful documentation on how to check the proportional hazards assumption HERE
As for example 3, I would guess that there are few individuals with event times past 3500. This is why you have those large jumps.
First of all, thank you very much for your answer, you helped me a lot already!
Regarding what you said about the ratio of hazards being constant over time I can understand some of the behaviour in example's 2 and 3, however, doesn't that invalidate example 1?
I've read about the proportional hazard assumption and to my understanding, the violation of a proportional hazard assumption occurs when a covariate doesn't impact the hazards constantly over time? I tried to test it on my variable ajcc_pathologic_stage
using check_assumptions()
, however, I'm getting very weird behaviour:
CoxPHFitter.check_assumptions()
isn't the same as the one in fit. In fit p-value is <0.005 and when I use check assumptions it is 0.1655Edit:
I think my mistake was I though the km-time varying p-value should be the same as the normal cox-ph fit p-value. Still, I'm having some difficulty interpreting the results. E.g taking ajcc_pathologic_stage
who's p-value is <0.005 with proportional hazards assumptions and 0.1655 with KM-time varying, does this mean this covariate shouldn't have a significant impact on hazards with time variation?
Below are some plots of hazard functions to help your intuition on what the proportional hazards assumption means. In all of the following scenarios, the hazard ratio is 2 for blue-to-red. However, the proportional hazards assumption is only true in A, B, and C.
Since the proportion is remaining constant, it can look a little hard to see. To make it easier on the eyes, you can log-transform the hazard functions. Below is a plot that does that.
As you can see, the hazards are a constant distance from each other for A, B, and C. Basically, the proportional hazards assumption is saying that your scenario looks something like one of those plots.
In scenario D, the hazard ratio varies over time. At the beginning it is basically HR=1. At the end it is basically HR=4. With the proportional hazards assumption, Cox regression will essentially take the 'average' of the hazards over time. In this case it would give you an estimate of HR=2. Depending on the scenario, this 'smoothing' may be more or less acceptable. But this is basically what the proportional hazards assumption does in the background.
The proportional hazards assumption is actually more problematic in the cases where the two hazard functions cross each other. Below is an example of that.
In this scenario, blue has an increased hazard at the start (relative to red) then a decreased at the end. A Cox proportional hazards model would give you HR=1 in this case, because the average over all time points is no relationship.
So back to the question regarding the check_assumptions
. The hypothesis test that there is no time-varying for the covariate is rejected (p=0.005) for ajc_pathologic_stage
. The next question is does this matter? Based on the attached plots, probably not. The values stay pretty close to 0 (except for the start), so it is probably fine to smooth over that part with the proportional hazards assumption. You may want to use a stratified Kaplan-Meier by ajc_pathologic_stage
to get an idea of what the hazards look like without stipulating the proportional hazards assumption. You can use KaplanMeierFitter.hazard_at_times()
to get the hazards and then log-transform to get a plot like the second.
Hi pzivich, Your answer was very complete and straight to the point, do you mind I copy it to the bioinformatics.stackexchange as the accepted answer, if you have an account tell me. Once again, thank you very much 👍
No problem! Yeah I can put the answer into the stackexchange if you could link it for me
@pzivich great answer and visuals 👍, I may copy some of this to lifelines docs, too
Hi and sorry for the long post in advance,
According to the documentation, this function plots the effect of a covariate on the observer's survival. I do understand that the CoxPH model assumes that the log-hazard of an individual is modelled by a linear function of their covariates, however, in some cases the effect of these covariates seems to perfect when plotted. I'll illustrate these with three images below describing the issues I have in my data analysis.
Example 1: This one I'm providing as it looks realistic to me, there is a clear difference in the effect of the covariate when positive or negative.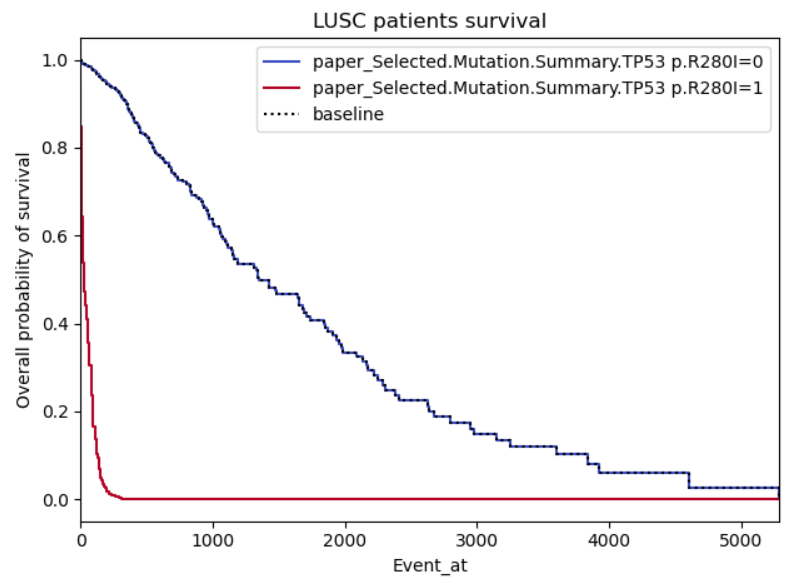
Example 2: This one just looks gimmicky, my concern is firstly there seems to be a perfect relation between the pathologic stage of cancer and the survival. It's logical that higher stages should impact the survival negatively, however, I don't understand why the graph is so symmetric as the survivability of cancer shouldn't be proportional to the patient cancer staging.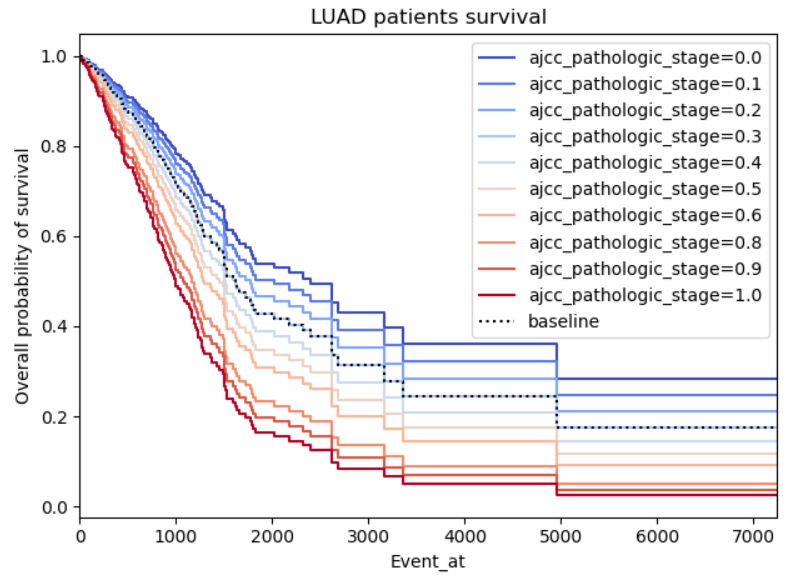
Example 3: Same problems as for example 2, this time with a binary not categorical variable (so I'm less reluctant on disbelieving), however, the curves do seem to symmetric, almost like it's the same curve but with a standard deviation. I also highlighted a region of interest which I don't understand why both curves stabilize (maybe lack of patients in those regions?)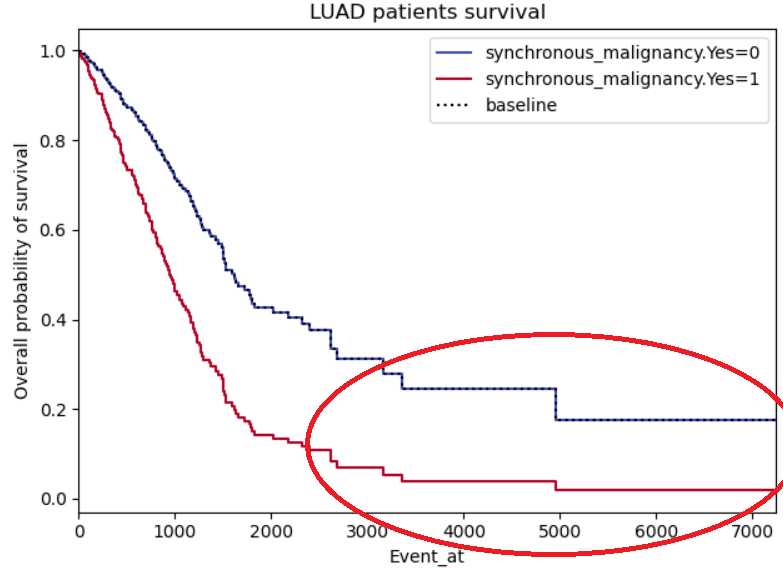