Closed jiwon-ryu closed 3 years ago
model = dict( type='SipMask', pretrained='open-mmlab://resnet101_caffe', backbone=dict( type='ResNet', depth=101, num_stages=4, out_indices=(0, 1, 2, 3), frozen_stages=1, norm_cfg=dict(type='BN', requires_grad=False), style='caffe'), neck=dict( type='FPN', in_channels=[256, 512, 1024, 2048], out_channels=256, start_level=1, add_extra_convs=True, extra_convs_on_inputs=False, # use P5 num_outs=5, relu_before_extra_convs=True), bbox_head=dict( type='SipMaskHead', num_classes=2, in_channels=256, stacked_convs=4, feat_channels=256, strides=[8, 16, 32, 64, 128], loss_cls=dict( type='FocalLoss', use_sigmoid=True, gamma=2.0, alpha=0.25, loss_weight=1.0), loss_bbox=dict(type='IoULoss', loss_weight=1.0), loss_centerness=dict( type='CrossEntropyLoss', use_sigmoid=True, loss_weight=1.0), center_sampling=True, center_sample_radius=1.5))
train_cfg = dict( assigner=dict( type='MaxIoUAssigner', pos_iou_thr=0.5, neg_iou_thr=0.4, min_pos_iou=0, ignore_iof_thr=-1), allowed_border=-1, pos_weight=-1, debug=False) test_cfg = dict( nms_pre=1000, min_bbox_size=0, score_thr=0.05, nms=dict(type='nms', iou_thr=0.5), max_per_img=100)
dataset_type = 'CocoDataset' data_root = '/home/mainuser/mount/nas/RJW/Cucumber-Dataset-Resized_shuffled/' img_norm_cfg = dict( mean=[102.9801, 115.9465, 122.7717], std=[1.0, 1.0, 1.0], to_rgb=False) train_pipeline = [ dict(type='LoadImageFromFile'), dict(type='LoadAnnotations', with_bbox=True, with_mask=True), dict(type='Resize', img_scale=[(1333, 800),(1333, 640)], keep_ratio=True), dict(type='RandomFlip', flip_ratio=0.5), dict(type='Normalize', **img_norm_cfg), dict(type='Pad', size_divisor=32), dict(type='DefaultFormatBundle'), dict(type='Collect', keys=['img', 'gt_bboxes', 'gt_labels', 'gt_masks']), ] test_pipeline = [ dict(type='LoadImageFromFile'),
dict(
type='MultiScaleFlipAug',
img_scale=(1333, 800),
flip=False,
transforms=[
dict(type='Resize', keep_ratio=True),
dict(type='RandomFlip'),
dict(type='Normalize', **img_norm_cfg),
dict(type='Pad', size_divisor=32),
dict(type='ImageToTensor', keys=['img']),
dict(type='Collect', keys=['img']),
])
] data = dict( imgs_per_gpu=2, workers_per_gpu=4, train=dict( type=dataset_type, ann_file=data_root + 'annotation/rand_train_coco.json', img_prefix=data_root + 'train/', pipeline=train_pipeline),
val=dict(
type=dataset_type,
ann_file=data_root + 'annotation/rand_val_coco.json',
img_prefix=data_root + 'val/',
pipeline=test_pipeline)
#classes=('cucumber',)),
test=dict(
type=dataset_type,
ann_file=data_root + 'annotation/rand_test_coco.json',
img_prefix=data_root + 'test/',
pipeline=test_pipeline),
#classes=('cucumber',))
) evaluation = dict(interval=1, metric='bbox')
optimizer = dict( type='SGD',
lr=0.005,
momentum=0.9,
weight_decay=0.0001,
paramwise_options=dict(bias_lr_mult=2., bias_decay_mult=0.))
optimizer_config = dict(grad_clip=dict(max_norm=35, norm_type=2))
lr_config = dict( policy='step', warmup='constant', warmup_iters=500, warmup_ratio=1.0 / 3, step=[40, 46]) checkpoint_config = dict(interval=1)
log_config = dict( interval=50, hooks=[ dict(type='TextLoggerHook'),
dict(
type='WandbLoggerHook',
init_kwargs=dict(project='YOLACT', name='SipMask_r101_4x'))
])
total_epochs = 48 dist_params = dict(backend='nccl') log_level = 'INFO' work_dir = '/home/mainuser/mount/nas/RJW/sipmask-cucumber/weights_r101_4x' load_from = None resume_from = None workflow = [('train', 1)] gpu_ids = [1]
Do you change the categories in coco.py? https://github.com/JialeCao001/SipMask/blob/bc63fa93f9291d7b664c065f41d937a65d3c72fd/SipMask-mmdetection/mmdet/datasets/coco.py#L19
Thanks for your quick response. Your solution works well :)
Hi,
I've just finished training my custom dataset using
sipmask_r101_caffe_fpn_gn_ms_4x.py
with SipMask-mmdetection.The training went fine, except that I found the label name in my inference result (image result) was wrong. It looks like the image below. (It is supposed to be 'cucumber' instead of 'person'.)
[Fig 1. Wrong label]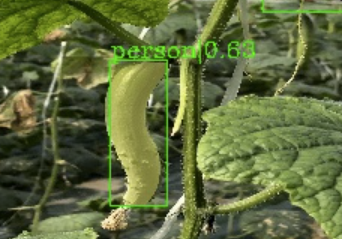
I think the label 'person' derived from the COCO label map because the class id of the 'cucumber' in my dataset and the 'person' in COCO are both 'zero'.
[Fig 2. My annotation file]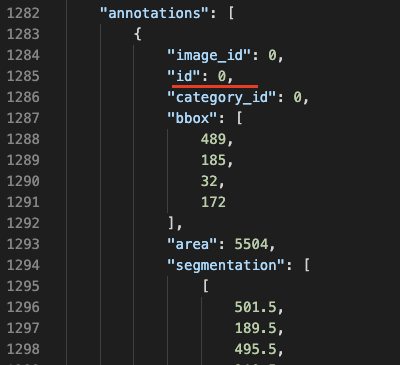
Can someone tell me how to set my own label map inside the configuration file?
I remember I could set
classes=('cucumber',)
in the config file when I was doing another task in mmdetection, but it didn't work with SipMask-mmdetection. Below is my configuration file.