Closed invisprints closed 4 years ago
Sorry, it's my problem. I put the original 68 landmarks in the folder. Maybe you can see our testset. Thanks!
Ok, another question is that the model will update the indices of 3D silhouette landmarks in your paper for correspondences between 3D vertices and 2D landmarks(Fig. 6 in paper), I wonder how it works in code, I haven't found it. Thank you very much if you could help me.
Yes. Due to COVID-19, I left some data (such as the nominated points of the silhouette) behind the school, so I didn't put that in our code. Maybe you can follow this function to renew the points of silhouette, but you have to label the nominated points manually. I feel sorry for that.
#V_NUM the number of vertices
#euler_angle a euler_angle tensor with size (m,3)
#scale a scale tensor with size (m,1)
#trans a translation matrix with size (m,2)
#points a point tensor with size (m,3,V_NUM)
#parallel a long tensor with size (17,4)
#best_51 a long tensor with size (51)
def FittingIndicesPlus(euler_angle, scale, trans, points, parallel, best_51):
batch_size = euler_angle.shape[0]
theta = euler_angle[:,0].reshape(-1,1,1)
phi = euler_angle[:,1].reshape(-1,1,1)
psi = euler_angle[:,2].reshape(-1,1,1)
one = torch.ones(batch_size,1,1).to(euler_angle.device)
zero = torch.zeros(batch_size, 1, 1).to(euler_angle.device)
rot_x = torch.cat((
torch.cat((one,zero,zero),1),
torch.cat((zero,theta.cos(), theta.sin()),1),
torch.cat((zero,-theta.sin(),theta.cos()),1),
),2)
rot_y = torch.cat((
torch.cat((phi.cos(),zero,-phi.sin()),1),
torch.cat((zero,one, zero),1),
torch.cat((phi.sin(),zero,phi.cos()),1),
),2)
rot_z = torch.cat((
torch.cat((psi.cos(),psi.sin(),zero),1),
torch.cat((-psi.sin(),psi.cos(), zero),1),
torch.cat((zero,zero,one),1),
),2)
rot = torch.bmm(rot_z, torch.bmm(rot_y,rot_x))
rott_geo = torch.bmm(rot, points)
mu = points
parallel_ids = parallel.reshape(-1)
parallels_vertex = torch.index_select(mu, 2, parallel_ids)
parallels_xy_t = torch.bmm(scale.reshape(-1,1,1)*rot[:,0:2,:].reshape(-1,2,3), parallels_vertex)
parallels_xy_t += trans.reshape(-1,2,1).expand_as(parallels_xy_t)
parallels_xy = torch.cat((parallels_xy_t[:,0,:].reshape(-1,1), parallels_xy_t[:,1,:].reshape(-1,1)), 1).reshape(-1,68,2)
front_part = parallels_xy[:,0:32,0].view(-1,8,parallel.shape[1])
behind_part = parallels_xy[:,32:68,0].view(-1,9,parallel.shape[1])
_, min_ids = torch.min(front_part,2)
_, max_ids = torch.max(behind_part,2)
ids = torch.cat((min_ids, max_ids), 1)
parallels_xy = parallels_xy.view(-1, parallel.shape[1],2)
landmarks = parallels_xy[torch.arange(0, parallels_xy.shape[0]).to(ids.device), ids.view(-1),:].reshape(batch_size,-1,2)
idx_51 = best_51
vertex = torch.index_select(mu, 2, idx_51)
xy_t = torch.bmm(scale.reshape(-1,1,1)*rot[:,0:2,:].reshape(-1,2,3), vertex)
xy_t += trans.reshape(-1,2,1).expand_as(xy_t)
xy = torch.cat((xy_t[:,0,:].reshape(-1,1), xy_t[:,1,:].reshape(-1,1)), 1).reshape(-1,51,2)
landmarks = torch.cat((landmarks,xy),1)
return rot, landmarks
The parallel
is a (17, 4) tensor (3D indices), which means each silhouette point has 4 nominated points (you can change the number). Then best_51
is other 51 landmarks (3D indices, 68 - 17 = 51).
Nice work, thank you!
You are welcome. I did not very good before, but I will improve in the feature.
All images in exp.zip are of size 224x224,but landmarks files seem not.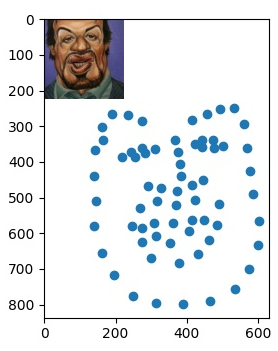