Joint detection and tracking model named DEFT, or ``Detection Embeddings for Tracking." Our approach relies on an appearance-based object matching network jointly-learned with an underlying object detection network. An LSTM is also added to capture motion constraints.
Hi @MedChaabane ,
first of all great work with this repository. Have tested on other videos and found the results to be quiet good.
However, to improve the results for the kind of scenarios that i plan to use this for, have been trying to train the LSTM based 2D tracker on my custom dataset following the process similar to training for kitti dataset.
I get a recursion error in the trajectory_dataset.py script. Have attached a screenshot below for reference.
There was a similar issue raised previously, where the solution was to manually give the "--num_classes" parameter. Tried that, but still facing the same issue.
Let me know if anyone has any fix or solution for this.
Hi @MedChaabane , first of all great work with this repository. Have tested on other videos and found the results to be quiet good. However, to improve the results for the kind of scenarios that i plan to use this for, have been trying to train the LSTM based 2D tracker on my custom dataset following the process similar to training for kitti dataset. I get a recursion error in the trajectory_dataset.py script. Have attached a screenshot below for reference. There was a similar issue raised previously, where the solution was to manually give the "--num_classes" parameter. Tried that, but still facing the same issue. Let me know if anyone has any fix or solution for this.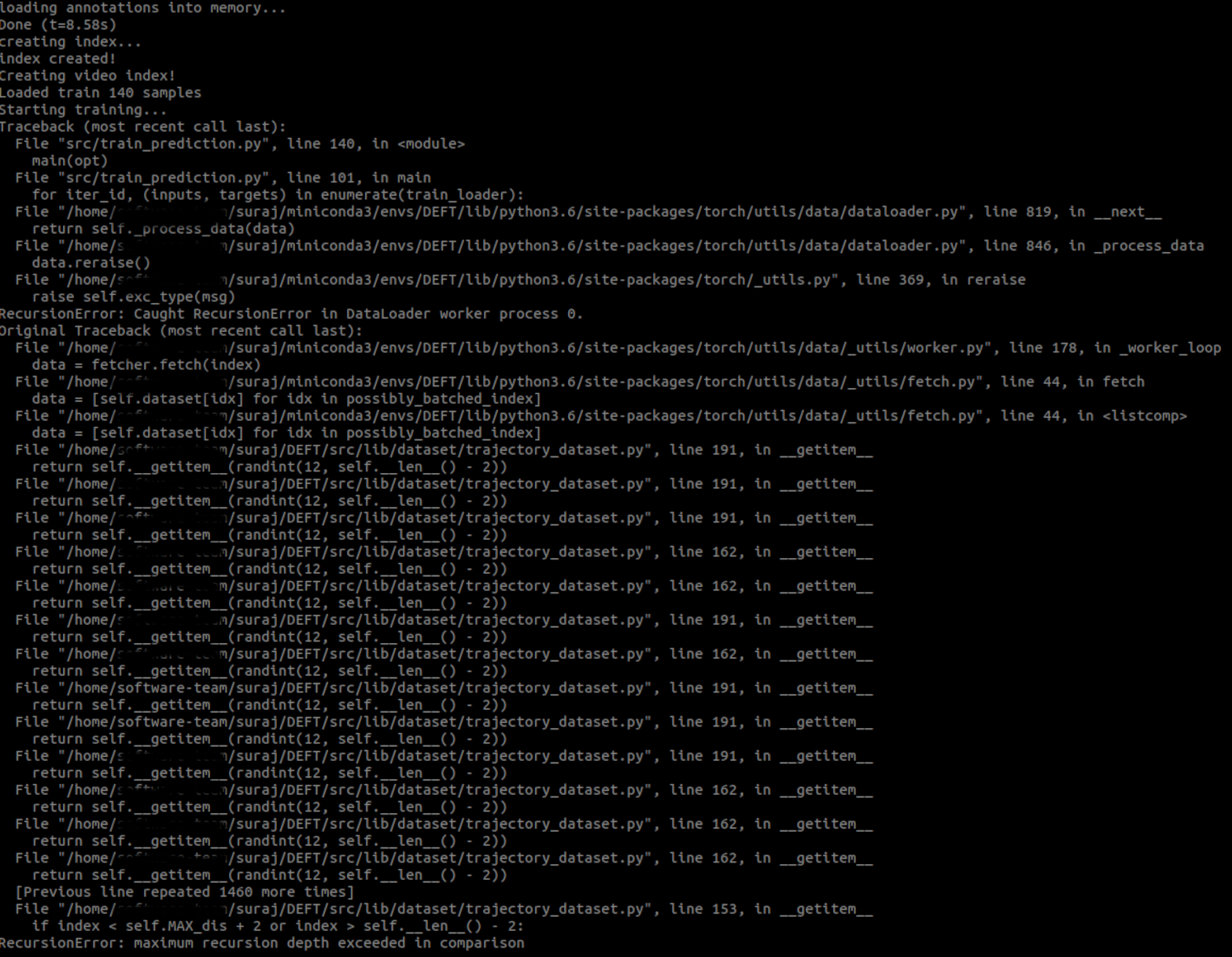