Merlin Systems provides tools for combining recommendation models with other elements of production recommender systems (like feature stores, nearest neighbor search, and exploration strategies) into end-to-end recommendation pipelines that can be served with Triton Inference Server.
Apache License 2.0
90
stars
30
forks
source link
[BUG] ValueError: Can't convert datetime64[ns] to a Triton dtype #307
This one is tricky, because Triton doesn't have a datetime dtype. It could be cast to an integer first, but that would currently need to happen in the Workflow
Bug description
Steps/Code to reproduce bug
I run this example from Merlin Models. I run it all the way (and inclusive) to the cell where I train the model:
Subsequently, I attempt to exporting the ensemble to perform inference on triton using the following code:
The following error occurs: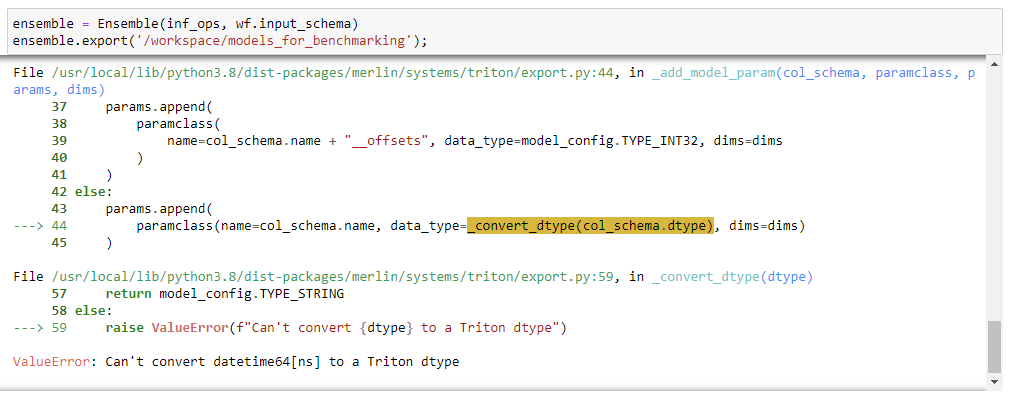
Expected behavior
The error doesn't occur and I am able to export the ensemble.
Environment details
I am running the 22.12 tensorflow container with all repos updated to current main.
Additional context