Open Bearwithchris opened 1 year ago
Hi, I have faced the same problem and found out that the default configuration on the model is different from the pre-trained one.
Change the configuration from --cfg=auto
(default) to --cfg=paper256
for this pre-trained model.
(For other pre-trained models, use the same model configuration as they were trained)
fakes_init.png
with --cfg=auto
:
fakes_init.png
with --cfg=paper256
:
The configuration controls the model's channel_base, the number of the mapping network's layers, and the minibatch standard deviation layer of the discriminator. For instance, the mapping network has 8 layers with --cfg=paper256
, while it has only 2 layers with --cfg=auto
.
To keep the model structure the same as the pre-trained one, you should ensure that fmaps
, map
, and mdstd
in the configuration are the same as it was trained.
In addition, when loading the pre-trained model, the function copy_params_and_buffers
would ignore the unexpected parameters in the pre-trained model without informing of such inconsistency.
Hi, I have faced the same problem and found out that the default configuration on the model is different from the pre-trained one.
Solution
Change the configuration from
--cfg=auto
(default) to--cfg=paper256
for this pre-trained model. (For other pre-trained models, use the same model configuration as they were trained)
fakes_init.png
with--cfg=auto
:
fakes_init.png
with--cfg=paper256
:
Explanation
The configuration controls the model's channel_base, the number of the mapping network's layers, and the minibatch standard deviation layer of the discriminator. For instance, the mapping network has 8 layers with
--cfg=paper256
, while it has only 2 layers with--cfg=auto
.To keep the model structure the same as the pre-trained one, you should ensure that
fmaps
,map
, andmdstd
in the configuration are the same as it was trained.In addition, when loading the pre-trained model, the function
copy_params_and_buffers
would ignore the unexpected parameters in the pre-trained model without informing of such inconsistency.
@JackywithaWhiteDog Hi. Where did this weight come from? I only get 256*256 pretrained weight in transfer-lerarning folder. The website is different from the one in this issue.
Hi @githuboflk, sorry that I didn't notice your question. I also used the pre-trained weight provided in README as you mentioned.
However, I think the checkpoint in this issue is available at NVIDIA NGC Catalog.
Hi,
Utilize the pre-trained pkl file: https://api.ngc.nvidia.com/v2/models/nvidia/research/stylegan2/versions/1/files/stylegan2-ffhq-256x256.pkl. I've attempted to transfer learning (without augmentation) from (FFHQ->CelebA-HQ).
However, when looking a the init generated images, I see this: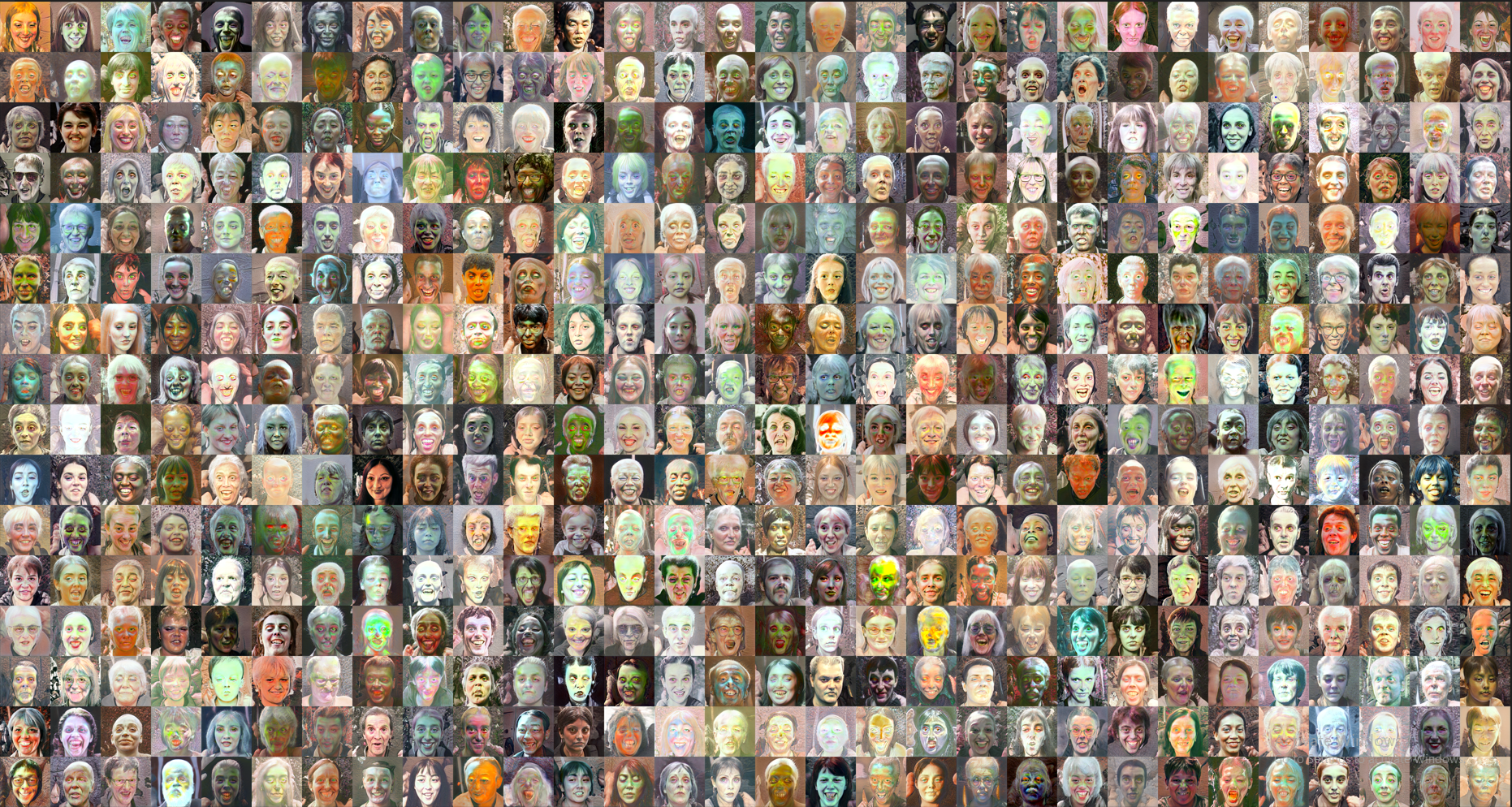
but when checking the FID against the FFHQ dataset FID=~9.
Can anyone explain what is going?