Closed SheldonZHANG closed 6 years ago
模型配置如下: def deepctr_net(input_dim, class_dim=2, emb_dim=64, fea_dim=128, is_predict=False):
E_AgeId = embedding_layer(input=data_layer("AgeId", 5+2), size=8)
E_GenderId = embedding_layer(input=data_layer("GenderId", 2+2), size=8)
embedding2 = embedding_layer(input=data_layer("MultiPreqIds", input_dim), \
size=emb_dim)
E_MultiPreqIds = text_conv_pool(input=embedding2, context_len=5, hidden_size=fea_dim)
embedding3 = embedding_layer(input=data_layer("QueryProfileIds", input_dim), \
size=emb_dim)
E_QueryProfileIds = text_conv_pool(input=embedding3, context_len=5, hidden_size=fea_dim)
embedding4 = embedding_layer(input=data_layer("WordProfileIds", input_dim), \
size=emb_dim)
E_WordProfileIds = text_conv_pool(input=embedding4, context_len=5, hidden_size=fea_dim)
embedding5 = embedding_layer(input=data_layer("TitleProfileIds", input_dim), \
size=emb_dim)
E_TitleProfileIds = text_conv_pool(input=embedding5, context_len=5, hidden_size=fea_dim)
E_TotalProfile = data_layer("TotalProfile", 2)
E_CmatchProfile = data_layer("CmatchProfile", 4)
E_MtidProfile = data_layer("MtidProfile", 4)
embedding6 = embedding_layer(input=data_layer("GsFeedTitleListIds", input_dim), \
size=emb_dim)
E_GsFeedTitleListIds = text_conv_pool(input=embedding6, context_len=5, hidden_size=fea_dim)
embedding7 = embedding_layer(input=data_layer("GsFeedMultiPreqIds", input_dim), \
size=emb_dim)
E_GsFeedMultiPreqIds = text_conv_pool(input=embedding7, context_len=5, hidden_size=fea_dim)
E_Cmatch = embedding_layer(input=data_layer("Cmatch", 33+2), size=4)
embedding9 = embedding_layer(input=data_layer("Ua", input_dim), size=emb_dim)
E_Ua = text_conv_pool(input=embedding9, context_len=5, hidden_size=fea_dim)
E_WnetType = embedding_layer(input=data_layer("WnetType", 4+2), size=8)
E_WosId = embedding_layer(input=data_layer("WosId", 2+2), size=8)
E_Pid = embedding_layer(input=data_layer("Pid", 37+2), size=8)
#gs1_embedding = embedding_layer(input=data_layer("GsFeedCatHisIds", input_dim), \
# size=emb_dim)
#E_GsFeedCatHisIds = text_conv_pool(input=gs1_embedding, context_len=5,
# hidden_size=fea_dim)
#gs2_embedding = embedding_layer(input=data_layer("GsFeedSubCatHisIds", input_dim), \
# size=emb_dim)
#E_GsFeedSubCatHisIds = text_conv_pool(input=gs2_embedding, context_len=5,
# hidden_size=fea_dim)
#gs3_embedding = embedding_layer(input=data_layer("GsFeedSiteHisIds", input_dim), \
# size=emb_dim)
#E_GsFeedSiteHisIds = text_conv_pool(input=gs3_embedding, context_len=5,
# hidden_size=fea_dim)
title_embedding = embedding_layer(input=data_layer("Title", input_dim), \
size=emb_dim)
E_Title = text_conv_pool(input=title_embedding, context_len=5, hidden_size=fea_dim)
#brand_embedding = embedding_layer(input=data_layer("BrandIds", input_dim), \
# size=emb_dim)
#E_BrandIds = text_conv_pool(input=brand_embedding, context_len=5, hidden_size=fea_dim)
E_TransType = embedding_layer(input=data_layer("TransType", 5+2), size=8)
#E_OcpcLevel = embedding_layer(input=data_layer("OcpcLevel", 2+2), size=8)
emb_vec = [
E_AgeId,
E_GenderId,
E_MultiPreqIds,
E_QueryProfileIds,
E_WordProfileIds,
E_TitleProfileIds,
E_TotalProfile,
E_CmatchProfile,
E_MtidProfile,
E_GsFeedTitleListIds,
E_GsFeedMultiPreqIds,
E_Cmatch,
E_Ua,
E_WnetType,
E_WosId,
E_Pid,
#E_GsFeedCatHisIds,
#E_GsFeedSubCatHisIds,
#E_GsFeedSiteHisIds,
E_Title,
#E_BrandIds,
E_TransType,
#E_OcpcLevel,
]
user_emb_vec = [
E_AgeId,
E_GenderId,
E_MultiPreqIds,
E_QueryProfileIds,
E_WordProfileIds,
E_TitleProfileIds,
E_TotalProfile,
E_CmatchProfile,
E_MtidProfile,
E_GsFeedTitleListIds,
E_GsFeedMultiPreqIds,
E_Cmatch,
E_Ua,
E_WnetType,
E_WosId,
E_Pid,
#E_GsFeedCatHisIds,
#E_GsFeedSubCatHisIds,
#E_GsFeedSiteHisIds,
]
ad_emb_vec = [
E_Title,
#E_BrandIds,
E_TransType,
#E_OcpcLevel
]
user_emb1 = fc_layer(name="user_emb1", input=concat_layer(input=user_emb_vec), size=256)
user_emb2 = fc_layer(name="user_emb2", input=user_emb1, size=128)
#user_emb3 = fc_layer(name="user_emb3", input=user_emb2, size=128)
#user_emb4 = fc_layer(name="user_emb4", input=user_emb3, size=128)
ad_emb1 = fc_layer(name="ad_emb1", input=concat_layer(input=ad_emb_vec), size=256)
ad_emb2 = fc_layer(name="ad_emb2", input=ad_emb1, size=128)
#ad_emb3 = fc_layer(name="ad_emb3", input=ad_emb2, size=128)
#ad_emb4 = fc_layer(name="ad_emb4", input=ad_emb3, size=128)
sim = cos_sim(a=user_emb2, b=ad_emb2)
output2 = fc_layer(name="output2", input=sim, size=class_dim, act=SoftmaxActivation())
emb = concat_layer(input=emb_vec)
fc1 = fc_layer(name="fc1", input=emb, size=512)
fc2 = fc_layer(name="fc2", input=fc1, size=256)
fc3 = fc_layer(name="fc3", input=fc2, size=128)
fc4 = fc_layer(name="fc4", input=fc3, size=64)
fc5 = fc_layer(name="fc5", input=fc4, size=32)
output1 = fc_layer(name="output1", input=fc5, size=class_dim, act=SoftmaxActivation())
if not is_predict:
lbl = data_layer("label", 1)
evalor = evaluators.auc_evaluator(output1,lbl)
evalor = evaluators.auc_evaluator(output2,lbl)
outputs([classification_cost(input=output1, label=lbl), classification_cost(input=output2, label=lbl)])
#outputs([classification_cost(input=output2, label=lbl)])
else:
outputs([output1, output2, ad_emb2])
#outputs([output2, ad_emb2])
def hook(settings, dict_file, schema_file, feature_file, **kwargs):
"""
init
"""
del kwargs
settings.logger.info("hook")
word_dict = util.load_dict(dict_file)
dict_size = len(word_dict)
settings.logger.info("dict_size is %d" % dict_size)
settings.input_types = [
integer_value(5+2), #ageid
integer_value(2+2), #genderid
integer_value_sequence(dict_size + 1), #multi_preq_ids
integer_value_sequence(dict_size + 1), #query_profile
integer_value_sequence(dict_size + 1), #word_profile
integer_value_sequence(dict_size + 1), #title_profile
dense_vector(2), #total_profile
dense_vector(4), #cmatch_profile
dense_vector(4), #mtid_profile
integer_value_sequence(dict_size + 1), #GsFeedTitleListIds
integer_value_sequence(dict_size + 1), # GsFeedMultiPreqIds
integer_value(33+2), #cmatch_fea
integer_value_sequence(dict_size + 1), #ua_fea
integer_value(4+2), #nettype
integer_value(2+2), #os
integer_value(37+2), #pid
#integer_value_sequence(dict_size + 1), #GsFeedCatHisIds
#integer_value_sequence(dict_size + 1), # GsFeedSubCatHisIds
#integer_value_sequence(dict_size + 1), # GsFeedSiteHisIds
integer_value_sequence(dict_size + 1), #title_ids
#integer_value_sequence(dict_size + 1), #BrandIds
integer_value(5+2), # trans_type
#integer_value(2+2), # ocpc_level
integer_value(2)] #conv
settings.default_ins = [
8, #age
2, #gender
[5131], #MultiPreqIds
[0], #QueryProfiles
[0], #WordProfile
[0], #TitleProfile
[0,0], #TotalProfile
[0,0,0,0], #CmatchProfile
[0,0,0,0], #MtidProfile
[0], #GsFeedTitleList
[0], #GsFeedMultiPreqIds
0, #cmatch
[0], #ua
0, #nettype
0, #os
0, #pid
#[0], #GsFeedCatHisIds
#[0], #GsFeedSubCatHisIds
#[0], #GsFeedSiteHisIds
[0], #title
#[0], #BrandIds
0, #transtype
#0, #ocpc_level
0, #conv
]
@provider(pool_size=8192, init_hook=hook)
def process_deep(settings, file_name):
"""
process feednnq shitu
"""
settings.logger.info("read begin of %s" % file_name)
try:
ins_count=0
for line in util.smart_open(file_name):
slot_list = list()
arr = line.strip('\n').split('\t')
ins_arr = arr[0].split(" ")
show = int(ins_arr[0])
clk = int(ins_arr[1])
slot_num = len(ins_arr)
for idx in range(2, slot_num):
tmp = ins_arr[idx].split(":")
slot_idx = int(tmp[1])
if slot_idx in [17, 18, 19, 21, 23]:
continue
if slot_idx in [3, 4, 5, 6, 7, 8, 9, 10, 11, 13, 17, 18, 19, 20, 21]:
if slot_idx in [7,8,9]:
slot_content = map(float, tmp[0].split(","))
else:
slot_content = map(int, tmp[0].split(','))
else:
slot_content = int(tmp[0])
slot_list.append(slot_content)
slot_list.append(clk)
if len(slot_list) != 19:
continue
ins_count+=1
yield slot_list
except Exception as e:
settings.logger.info("error: %s" % e)
settings.logger.info("read finish %s: %d" % (file_name, ins_count))
can you please paste the node status like memory usage and the if all the process are running? and try decrease the pool_size to reduce memory usage.
您好,此issue在近一个月内暂无更新,我们将于今天内关闭。若在关闭后您仍需跟进提问,可重新开启此问题,我们将在24小时内回复您。因关闭带来的不便我们深表歉意,请您谅解~感谢您对PaddlePaddle的支持! Hello, this issue has not been updated in the past month. We will close it today for the sake of other user‘s experience. If you still need to follow up on this question after closing, please feel free to reopen it. In that case, we will get back to you within 24 hours. We apologize for the inconvenience caused by the closure and thank you so much for your support of PaddlePaddle Group!
模型是v1.11.0版本,双塔DNN,模型在读入输入的时候卡住了;而且不再继续打印日志 ;z这种情况总是反复出现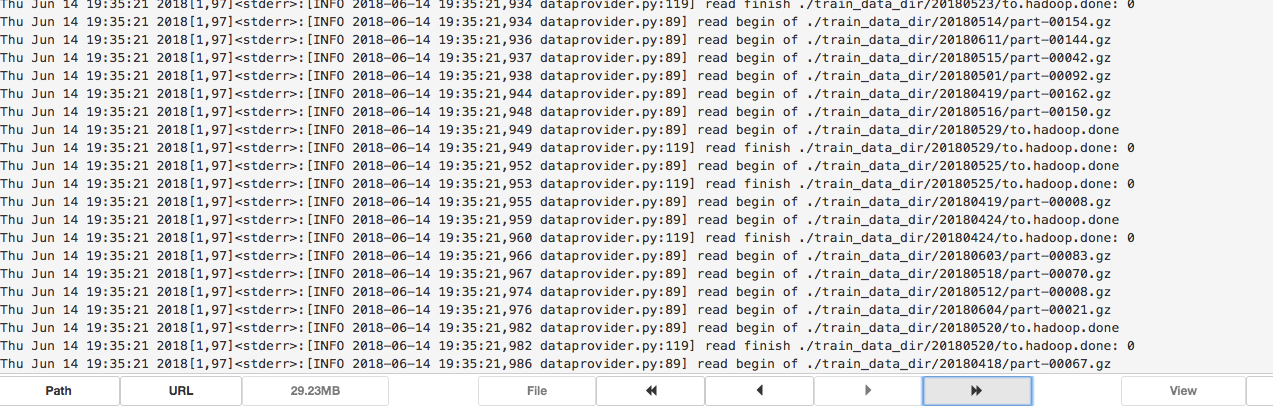