Closed bparaj closed 7 years ago
Have you done any finetuning?
You've changed the last layer to recognize fewer classes, so the weights that assign an image to a class are indeed random (kinda, it's actually glorot_uniform
). You need to train the last layer in order to get results that make sense with your new setup.
Closing this for now, feel free to reopen it if you still have issues.
I changed the setup as per your suggestion (Thank you!) as follows:
Set the number of classes to 20: get_model(20)
I chose 20 because when I called transfer_weights()
with keep_top=True
, I got the error:
ValueError: Layer weight shape (2, 2, 11, 16) not compatible with provided weight shape (2, 2, 20, 16)
And I assumed the weights were trained for 20 classes. Did I make a wrong assumption?
Didn't discard the top layer: autoencoder = transfer_weights(model=autoencoder, keep_top=True)
Since I didn't discard the top layer, is it okay to use the function save_output()
as defined above?
This time, for the same input image included above, the segmented output created with save_output()
is as follows:
Also, by finetuning, do you mean retraining the model?
I'm not sure what I'm seeing. You're assigning random colors to each class so you can't see what class e.g. the pink color corresponds to. The most dominant one should be the background class though.
How many classes do you have in your dataset? class 0 corresponds to some concept, you can't just assume it will translate well to your problem. If you want to do inference for 11 classes on a furniture dataset you can't reuse the 20 classes that were trained on a dataset that recognizes cars and pedestrians. You have to retrain in order to reassign the internal representation of the intermediate layers to actual high-level concepts that we understand.
If you discard the (weights of the) top layer, then it makes sense to get random outputs like the ones you got before. On the other hand, if you don't discard the (weights of the) top layer, then you'll only be able to segment the objects that exist in the original dataset.
Prediction works with argmax and there's no threshold to filter noise, so if you look more closely to the actual one-hot-vector predictions for each (non-background) pixel, you should be seeing low values for all classes (low confidence). Technically you should get 100% background but the network isn't perfect and I'm pretty sure there's a bug somewhere in my implementation of enet that I haven't pinpointed yet.
Re: Finetuning. Come on, it's pretty common stuff, you could have looked it up. ;)
Thanks @PavlosMelissinos! That clarifies a ton!
I have the following function
get_model()
which returns the enet model with weights loaded fromtorch_enet.pkl
. The functionsbuild()
andtransfer_weights()
are fromsrc/test.py
.I created a model with 11 classes by calling
get_model(11)
. I fed the image2015-11-08T13.52.54.655-0000011482.jpg
from SUNRGBD dataset. The model gave a prediction tensor which I reshaped to (256, 256, 11). To visualize the predictions, I used the following function to save that tensor as an image:The output shows almost random assignment of colors and there's no visible segmentation at all.
The input and the corresponding segmented output can be found below:
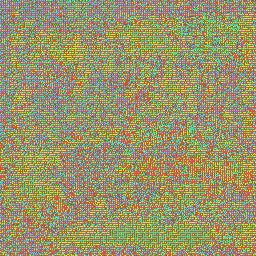