Closed HenryZhangJianhe closed 1 year ago
dist方式跑eval我还没测过的,目前不太清楚会有什么坑,或许等忙过这一阵我一并检查下dist方式的训练和测试流程。
Henry @.***> 于2023年4月8日周六 14:15写道:
我下载了作者train的模型本地使用pytorch进行test和eval, 具体命令参照作者workdir文件下对应的log文件,但是我用torch推理后测评的结果比作者log中的结果低很多,不同之处: 作者是用slurm我是用pytorch,我两张A100推理,难道与这有关?还是其他什么因素 [image: image] https://user-images.githubusercontent.com/52202915/230706508-112ee1ee-4d5b-48ec-aa26-a9d590ca2826.png
我对作者m2模型测评结果 [image: image] https://user-images.githubusercontent.com/52202915/230706547-bca061e2-b4d8-4668-b73a-812cd2cea3bb.png
具体测试命令如下,请指教
test
python3 -m torch.distributed.launch --nnodes=1 --node_rank=0 --nproc_per_node 2 --master_addr 127.0.0.1 tools/test.py \ configs/fastbev/exp/paper/fastbev_m2_r34_s256x704_v200x200x4_c224_d4_f4.py work_dirs/fastbev/exp/paper/fastbev_m2_r34_s256x704_v200x200x4_c224_d4_f4/epoch_20.pth \ --launcher=pytorch --out work_dirs/fastbev/exp/paper/fastbev_m2_r34_s256x704_v200x200x4_c224_d4_f4/results/results.pkl \ --format-only \ --eval-options jsonfile_prefix=work_dirs/fastbev/exp/paper/fastbev_m2_r34_s256x704_v200x200x4_c224_d4_f4/results
eval
python3 -m torch.distributed.launch --nnodes=1 --node_rank=0 --nproc_per_node 2 --master_addr 127.0.0.1 tools/eval.py \ configs/fastbev/exp/paper/fastbev_m2_r34_s256x704_v200x200x4_c224_d4_f4.py \ --launcher=pytorch --out work_dirs/fastbev/exp/paper/fastbev_m2_r34_s256x704_v200x200x4_c224_d4_f4/results/results.pkl \ --eval bbox
— Reply to this email directly, view it on GitHub https://github.com/Sense-GVT/Fast-BEV/issues/47, or unsubscribe https://github.com/notifications/unsubscribe-auth/A2SSIGY2GMCTK6F7R235L6DXAD673ANCNFSM6AAAAAAWXIPKZI . You are receiving this because you are subscribed to this thread.Message ID: @.***>
此外我想请教个问题,对于论文Multi-view to one-Voxel中For the case of multiple views with overlapping areas, we directly adopt the first encountered view,对于重叠的2d特征并没做任何手段进行利用,这是为什么,或者您有尝试其他什么方法进行利用吗?
另外设置n_voxels为200x200x6, 然后voxel size为[0.5,0.5,1] 这里有什么根据吗?按道理projection是lidartoimg,这里图像特征维度发生变化,点云维度也该发生变化才能通过projection对应,也就是200x200x6应该和points-range对应起来,但是您在get-points函数里并未使用points-range信息?为什么voxel resolution增加到40040012,not help improveing performance?期待您的回复!
def get_points(n_voxels, voxel_size, origin):
points = torch.stack(
torch.meshgrid(
[
torch.arange(n_voxels[0]),
torch.arange(n_voxels[1]),
torch.arange(n_voxels[2]),
]
)
)
new_origin = origin - n_voxels / 2.0 * voxel_size
points = points * voxel_size.view(3, 1, 1, 1) + new_origin.view(3, 1, 1, 1)
return points
与NuscenesMultiView_Map_Dataset2()内的bev-gt有关吗
xbound = [-50, 50, 0.5]
ybound = [-50, 50, 0.5]
zbound = [-10, 10, 20.0]
dbound = [4.0, 45.0, 1.0]
self.nx = np.array([(row[1] - row[0]) / row[2] for row in [xbound, ybound, zbound]], dtype='int64')
self.dx = np.array([row[2] for row in [xbound, ybound, zbound]])
self.bx = np.array([row[0] + row[2] / 2.0 for row in [xbound, ybound, zbound]])
dist方式跑eval我还没测过的,目前不太清楚会有什么坑,或许等忙过这一阵我一并检查下dist方式的训练和测试流程。 Henry @.> 于2023年4月8日周六 14:15写道: … 我下载了作者train的模型本地使用pytorch进行test和eval, 具体命令参照作者workdir文件下对应的log文件,但是我用torch推理后测评的结果比作者log中的结果低很多,不同之处: 作者是用slurm我是用pytorch,我两张A100推理,难道与这有关?还是其他什么因素 [image: image] https://user-images.githubusercontent.com/52202915/230706508-112ee1ee-4d5b-48ec-aa26-a9d590ca2826.png 我对作者m2模型测评结果 [image: image] https://user-images.githubusercontent.com/52202915/230706547-bca061e2-b4d8-4668-b73a-812cd2cea3bb.png 具体测试命令如下,请指教 # test python3 -m torch.distributed.launch --nnodes=1 --node_rank=0 --nproc_per_node 2 --master_addr 127.0.0.1 tools/test.py \ configs/fastbev/exp/paper/fastbev_m2_r34_s256x704_v200x200x4_c224_d4_f4.py work_dirs/fastbev/exp/paper/fastbev_m2_r34_s256x704_v200x200x4_c224_d4_f4/epoch_20.pth \ --launcher=pytorch --out work_dirs/fastbev/exp/paper/fastbev_m2_r34_s256x704_v200x200x4_c224_d4_f4/results/results.pkl \ --format-only \ --eval-options jsonfile_prefix=work_dirs/fastbev/exp/paper/fastbev_m2_r34_s256x704_v200x200x4_c224_d4_f4/results # eval python3 -m torch.distributed.launch --nnodes=1 --node_rank=0 --nproc_per_node 2 --master_addr 127.0.0.1 tools/eval.py \ configs/fastbev/exp/paper/fastbev_m2_r34_s256x704_v200x200x4_c224_d4_f4.py \ --launcher=pytorch --out work_dirs/fastbev/exp/paper/fastbev_m2_r34_s256x704_v200x200x4_c224_d4_f4/results/results.pkl \ --eval bbox — Reply to this email directly, view it on GitHub <#47>, or unsubscribe https://github.com/notifications/unsubscribe-auth/A2SSIGY2GMCTK6F7R235L6DXAD673ANCNFSM6AAAAAAWXIPKZI . You are receiving this because you are subscribed to this thread.Message ID: @.>
我发现问题可能是nms-gpu,因为我发现生成boxes的时候,进行到mmdet3d/ops/iou3d/iou3d_utils.py的第46行
num_out = iou3d_cuda.nms_gpu(boxes, keep, thresh, boxes.device.index)
会在这打印出“Error”,但是程序不会挂,我发现nms处理前后的boxes数量没变化,如图所示.
我进一步排查是mmdet3d/ops/iou3d/src/iou3d.cpp代码的
if (cudaSuccess != cudaGetLastError()) printf("Error!\n");
请问可能是什么问题呢,编译的版本问题?
int nms_gpu(at::Tensor boxes, at::Tensor keep,
float nms_overlap_thresh, int device_id) {
// params boxes: (N, 5) [x1, y1, x2, y2, ry]
// params keep: (N)
CHECK_INPUT(boxes);
CHECK_CONTIGUOUS(keep);
cudaSetDevice(device_id);
int boxes_num = boxes.size(0);
const float *boxes_data = boxes.data_ptr<float>();
int64_t *keep_data = keep.data_ptr<int64_t>();
const int col_blocks = DIVUP(boxes_num, THREADS_PER_BLOCK_NMS);
unsigned long long *mask_data = NULL;
CHECK_ERROR(cudaMalloc((void **)&mask_data,
boxes_num * col_blocks * sizeof(unsigned long long)));
nmsLauncher(boxes_data, mask_data, boxes_num, nms_overlap_thresh);
// unsigned long long mask_cpu[boxes_num * col_blocks];
// unsigned long long *mask_cpu = new unsigned long long [boxes_num *
// col_blocks];
std::vector<unsigned long long> mask_cpu(boxes_num * col_blocks);
// printf("boxes_num=%d, col_blocks=%d\n", boxes_num, col_blocks);
CHECK_ERROR(cudaMemcpy(&mask_cpu[0], mask_data,
boxes_num * col_blocks * sizeof(unsigned long long),
cudaMemcpyDeviceToHost));
cudaFree(mask_data);
unsigned long long *remv_cpu = new unsigned long long[col_blocks]();
int num_to_keep = 0;
for (int i = 0; i < boxes_num; i++) {
int nblock = i / THREADS_PER_BLOCK_NMS;
int inblock = i % THREADS_PER_BLOCK_NMS;
if (!(remv_cpu[nblock] & (1ULL << inblock))) {
keep_data[num_to_keep++] = i;
unsigned long long *p = &mask_cpu[0] + i * col_blocks;
for (int j = nblock; j < col_blocks; j++) {
remv_cpu[j] |= p[j];
}
}
}
delete[] remv_cpu;
if (cudaSuccess != cudaGetLastError()) printf("Error!\n");
return num_to_keep;
}
dist方式跑eval我还没测过的,目前不太清楚会有什么坑,或许等忙过这一阵我一并检查下dist方式的训练和测试流程。 Henry @._> 于2023年4月8日周六 14:15写道: … 我下载了作者train的模型本地使用pytorch进行test和eval, 具体命令参照作者workdir文件下对应的log文件,但是我用torch推理后测评的结果比作者log中的结果低很多,不同之处: 作者是用slurm我是用pytorch,我两张A100推理,难道与这有关?还是其他什么因素 [image: image] https://user-images.githubusercontent.com/52202915/230706508-112ee1ee-4d5b-48ec-aa26-a9d590ca2826.png 我对作者m2模型测评结果 [image: image] https://user-images.githubusercontent.com/52202915/230706547-bca061e2-b4d8-4668-b73a-812cd2cea3bb.png 具体测试命令如下,请指教 # test python3 -m torch.distributed.launch --nnodes=1 --node_rank=0 --nproc_per_node 2 --master_addr 127.0.0.1 tools/test.py \ configs/fastbev/exp/paper/fastbev_m2_r34_s256x704_v200x200x4_c224_d4_f4.py work_dirs/fastbev/exp/paper/fastbev_m2_r34_s256x704_v200x200x4_c224_d4_f4/epoch_20.pth \ --launcher=pytorch --out work_dirs/fastbev/exp/paper/fastbev_m2_r34_s256x704_v200x200x4_c224_d4_f4/results/results.pkl \ --format-only \ --eval-options jsonfile_prefix=work_dirs/fastbev/exp/paper/fastbev_m2_r34_s256x704_v200x200x4_c224_d4_f4/results # eval python3 -m torch.distributed.launch --nnodes=1 --node_rank=0 --nproc_per_node 2 --master_addr 127.0.0.1 tools/eval.py \ configs/fastbev/exp/paper/fastbev_m2_r34_s256x704_v200x200x4_c224_d4_f4.py \ --launcher=pytorch --out work_dirs/fastbev/exp/paper/fastbev_m2_r34_s256x704_v200x200x4_c224_d4f4/results/results.pkl \ --eval bbox — Reply to this email directly, view it on GitHub <#47>, or unsubscribe https://github.com/notifications/unsubscribe-auth/A2SSIGY2GMCTK6F7R235L6DXAD673ANCNFSM6AAAAAAWXIPKZI . You are receiving this because you are subscribed to this thread.Message ID: @_._>
我发现问题可能是nms-gpu,因为我发现生成boxes的时候,进行到mmdet3d/ops/iou3d/iou3d_utils.py的第46行 num_out = iou3d_cuda.nms_gpu(boxes, keep, thresh, boxes.device.index) 会在这打印出“Error”,但是程序不会挂,我发现nms处理前后的boxes数量没变化,如图所示. 我进一步排查是mmdet3d/ops/iou3d/src/iou3d.cpp代码的 if (cudaSuccess != cudaGetLastError()) printf("Error!\n"); 请问可能是什么问题呢,编译的版本问题?
int nms_gpu(at::Tensor boxes, at::Tensor keep, float nms_overlap_thresh, int device_id) { // params boxes: (N, 5) [x1, y1, x2, y2, ry] // params keep: (N) CHECK_INPUT(boxes); CHECK_CONTIGUOUS(keep); cudaSetDevice(device_id); int boxes_num = boxes.size(0); const float *boxes_data = boxes.data_ptr<float>(); int64_t *keep_data = keep.data_ptr<int64_t>(); const int col_blocks = DIVUP(boxes_num, THREADS_PER_BLOCK_NMS); unsigned long long *mask_data = NULL; CHECK_ERROR(cudaMalloc((void **)&mask_data, boxes_num * col_blocks * sizeof(unsigned long long))); nmsLauncher(boxes_data, mask_data, boxes_num, nms_overlap_thresh); // unsigned long long mask_cpu[boxes_num * col_blocks]; // unsigned long long *mask_cpu = new unsigned long long [boxes_num * // col_blocks]; std::vector<unsigned long long> mask_cpu(boxes_num * col_blocks); // printf("boxes_num=%d, col_blocks=%d\n", boxes_num, col_blocks); CHECK_ERROR(cudaMemcpy(&mask_cpu[0], mask_data, boxes_num * col_blocks * sizeof(unsigned long long), cudaMemcpyDeviceToHost)); cudaFree(mask_data); unsigned long long *remv_cpu = new unsigned long long[col_blocks](); int num_to_keep = 0; for (int i = 0; i < boxes_num; i++) { int nblock = i / THREADS_PER_BLOCK_NMS; int inblock = i % THREADS_PER_BLOCK_NMS; if (!(remv_cpu[nblock] & (1ULL << inblock))) { keep_data[num_to_keep++] = i; unsigned long long *p = &mask_cpu[0] + i * col_blocks; for (int j = nblock; j < col_blocks; j++) { remv_cpu[j] |= p[j]; } } } delete[] remv_cpu; if (cudaSuccess != cudaGetLastError()) printf("Error!\n"); return num_to_keep; }
破案了 原因是我用的docker中mmdet3d不是在A100上编译的,我在A100重新编译后,运行就没这个错误了。 然后重新eval了你的m2模型,精度不错赞赞赞
@HenryZhangJianhe 您好,我按照您的方案重新编译运行后依然碰到不断print Error!的问题,指标劣化,请问还有哪些细节需要修改吗?
我下载了作者train的模型本地使用pytorch进行test和eval, 具体命令参照作者workdir文件下对应的log文件,但是我用torch推理后测评的结果比作者log中的结果低很多,不同之处: 作者是用slurm我是用pytorch,我两张A100推理,难道与这有关?还是其他什么因素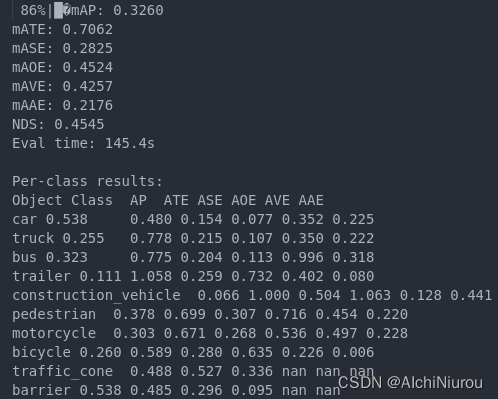
我对作者m2模型测评结果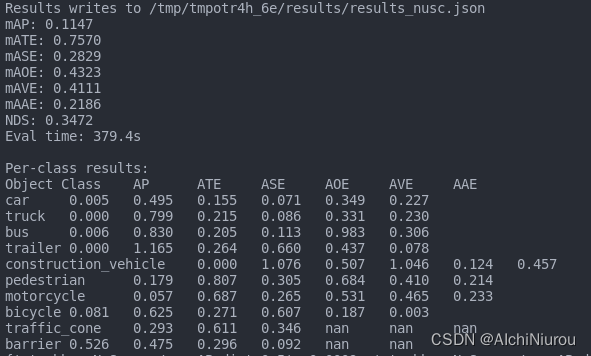
具体测试命令如下,请指教