Closed Some-random closed 3 years ago
This is my config
###########################################################
########################################################### sampling_rate: 16000 hop_size: 256 # Hop size. format: "npy"
###########################################################
########################################################### generator_params: out_channels: 4 # Number of output channels (number of subbands). kernel_size: 7 # Kernel size of initial and final conv layers. filters: 384 # Initial number of channels for conv layers. upsample_scales: [2, 4, 8] # List of Upsampling scales. stack_kernel_size: 3 # Kernel size of dilated conv layers in residual stack. stacks: 4 # Number of stacks in a single residual stack module. is_weight_norm: false # Use weight-norm or not.
###########################################################
########################################################### discriminator_params: out_channels: 1 # Number of output channels. scales: 3 # Number of multi-scales. downsample_pooling: "AveragePooling1D" # Pooling type for the input downsampling. downsample_pooling_params: # Parameters of the above pooling function. pool_size: 4 strides: 2 kernel_sizes: [5, 3] # List of kernel size. filters: 16 # Number of channels of the initial conv layer. max_downsample_filters: 512 # Maximum number of channels of downsampling layers. downsample_scales: [4, 4, 4] # List of downsampling scales. nonlinear_activation: "LeakyReLU" # Nonlinear activation function. nonlinear_activation_params: # Parameters of nonlinear activation function. alpha: 0.2 is_weight_norm: false # Use weight-norm or not.
###########################################################
########################################################### stft_loss_params: fft_lengths: [1024, 2048, 512] # List of FFT size for STFT-based loss. frame_steps: [120, 240, 50] # List of hop size for STFT-based loss frame_lengths: [600, 1200, 240] # List of window length for STFT-based loss.
subband_stft_loss_params: fft_lengths: [384, 683, 171] # List of FFT size for STFT-based loss. frame_steps: [30, 60, 10] # List of hop size for STFT-based loss frame_lengths: [150, 300, 60] # List of window length for STFT-based loss.
###########################################################
########################################################### lambda_feat_match: 10.0 # Loss balancing coefficient for feature matching loss lambda_adv: 2.5 # Loss balancing coefficient for adversarial loss.
###########################################################
########################################################### batch_size: 64 # Batch size. batch_max_steps: 8192 # Length of each audio in batch for training. Make sure dividable by hop_size. batch_max_steps_valid: 81920 # Length of each audio for validation. Make sure dividable by hope_size. remove_short_samples: true # Whether to remove samples the length of which are less than batch_max_steps. allow_cache: true # Whether to allow cache in dataset. If true, it requires cpu memory. is_shuffle: true # shuffle dataset after each epoch.
###########################################################
########################################################### generator_optimizer_params: lr_fn: "PiecewiseConstantDecay" lr_params: boundaries: [100000, 200000, 300000, 400000, 500000, 600000, 700000] values: [0.001, 0.0005, 0.00025, 0.000125, 0.0000625, 0.00003125, 0.000015625, 0.000001] amsgrad: false
discriminator_optimizer_params: lr_fn: "PiecewiseConstantDecay" lr_params: boundaries: [100000, 200000, 300000, 400000, 500000] values: [0.00025, 0.000125, 0.0000625, 0.00003125, 0.000015625, 0.000001] amsgrad: false
###########################################################
########################################################### discriminator_train_start_steps: 200000 # steps begin training discriminator train_max_steps: 4000000 # Number of training steps. save_interval_steps: 20000 # Interval steps to save checkpoint. eval_interval_steps: 5000 # Interval steps to evaluate the network. log_interval_steps: 200 # Interval steps to record the training log.
###########################################################
########################################################### num_save_intermediate_results: 1 # Number of batch to be saved as intermediate results.
you need to run more iters, https://github.com/kan-bayashi/ParallelWaveGAN/issues/236
My tensorboard looks like this, it seems like the my model diverged after 600k iters. But the frequency noise was there before the divergence. I'm wondering whether this will have something to do with the fact that my training data is very small (only around 1k sentences)
Also I'm wondering why does the frequency band noise always appear at 1/4 the maximum frequency (4000 Hz for 16000 Hz audio in my case and 2000 Hz for 8000 Hz audio in espnet issue)?
Also I'm wondering why does the frequency band noise always appear at 1/4 the maximum frequency (4000 Hz for 16000 Hz audio in my case and 2000 Hz for 8000 Hz audio in espnet issue)?
is this ok now ?
Also I'm wondering why does the frequency band noise always appear at 1/4 the maximum frequency (4000 Hz for 16000 Hz audio in my case and 2000 Hz for 8000 Hz audio in espnet issue)?
Because the predict of subbands of top frequency is difficult. In my opinion, fisrtly, high frequency has lower energy (for mse loss , smaller weight of total loss); secondly, top subbands of frequecy represent high frequency waveform which is difficult for transposed convolution to simulate. Continuing to train may lighten this noise.
This issue has been automatically marked as stale because it has not had recent activity. It will be closed if no further activity occurs.
same question, any other ways to solve this problem?
Hi, I found some strange looking spectograms when using multiband melgan. Can someone take a look at it?
Case 1: bright horizontal line around 4k freq band ref: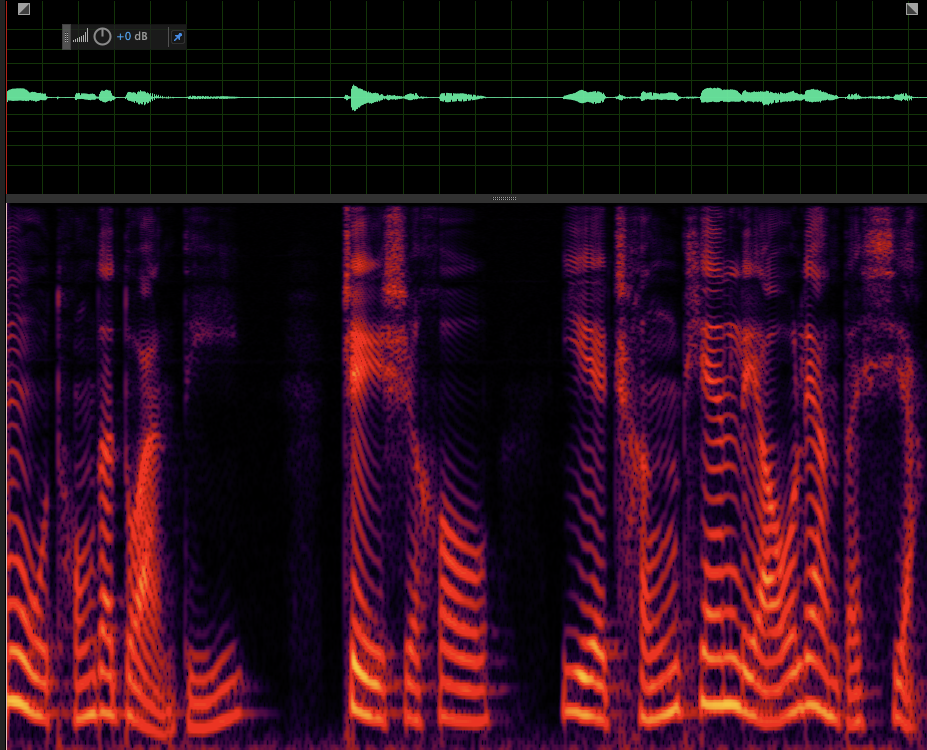
gen: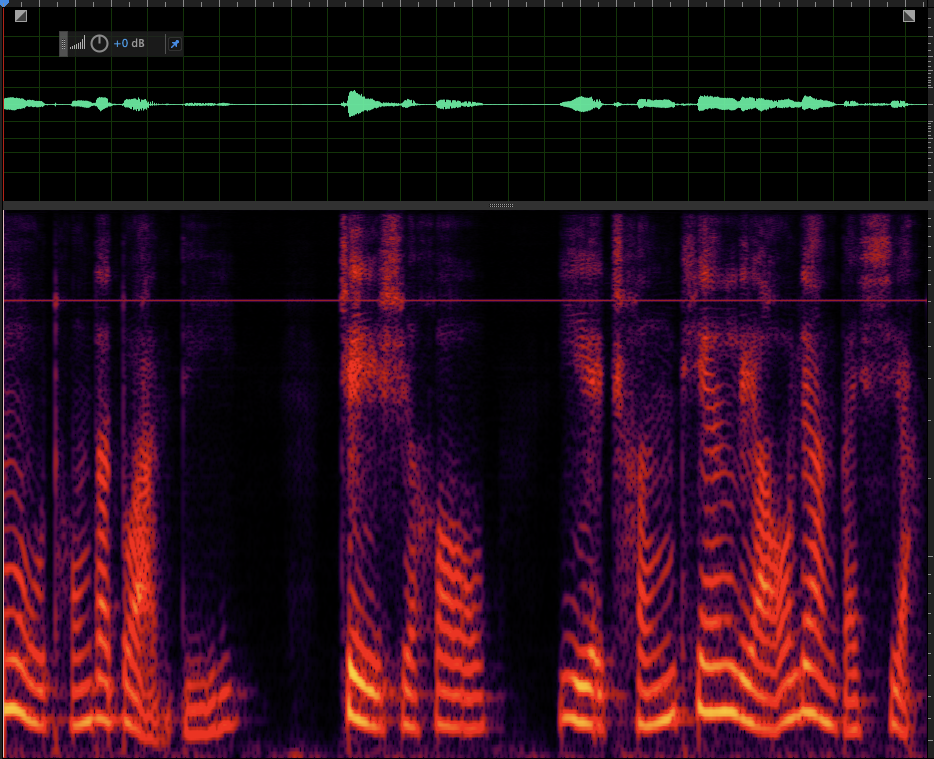
Case 2: horizontal lines when it's supposed to be silence ref: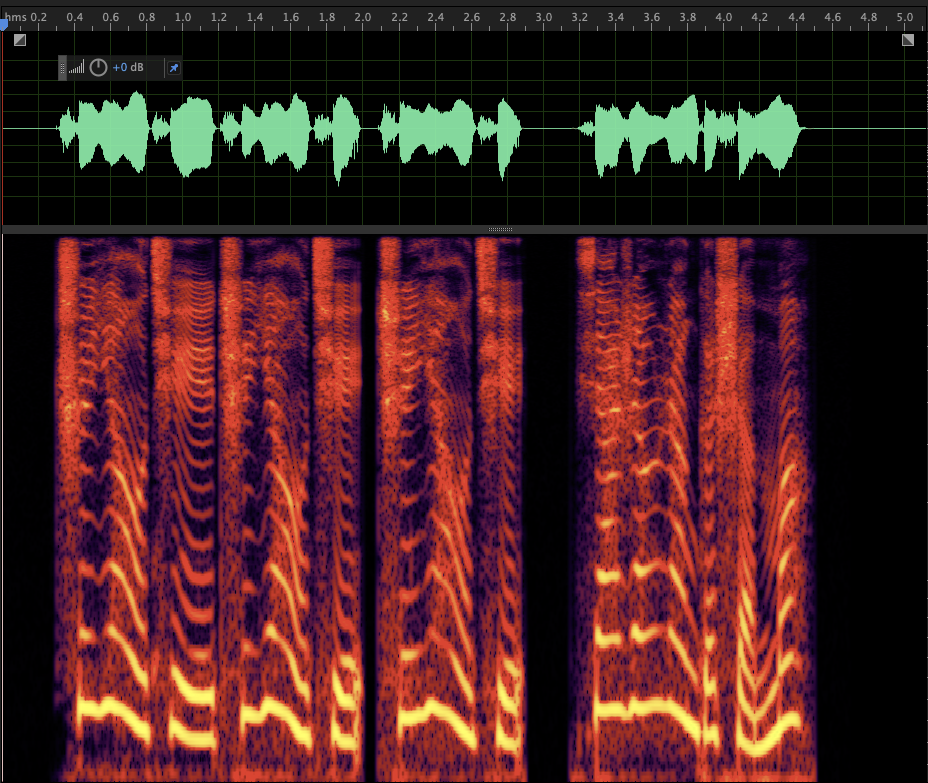
gen: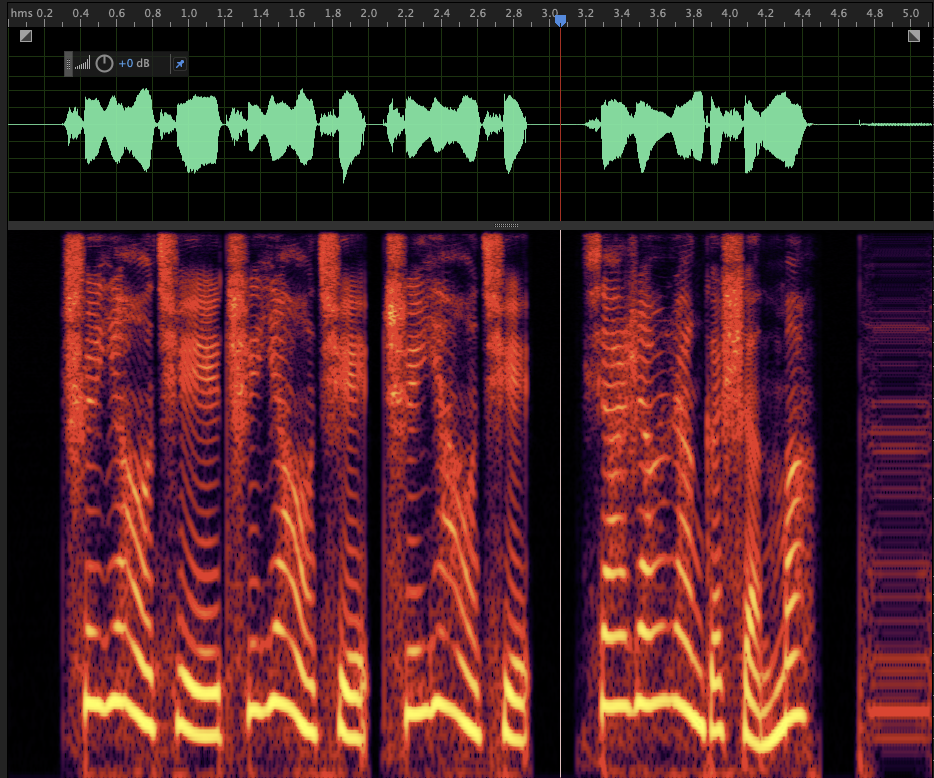