Open ajay1606 opened 2 years ago
Hello, Would you please share some tips to overcome detection failure with the following scenarios?
Issues;
I currently am using a pre-trained model: condlane_r101_culane.pth
With the configuration is as follows:
net = dict( type='Detector', ) backbone = dict( type='ResNetWrapper', resnet='resnet101', pretrained=True, replace_stride_with_dilation=[False, False, False], out_conv=False, in_channels=[64, 128, 256, 512] ) ori_img_h = 2048 ori_img_w = 2448 bbox_h_start = 1024 crop_bbox = [0, bbox_h_start, ori_img_w, ori_img_h] sample_y = range(ori_img_h, bbox_h_start, -8) batch_size = 1 aggregator = dict( type='TransConvEncoderModule', in_dim=2048, attn_in_dims=[2048, 256], attn_out_dims=[256, 256], strides=[1, 1], ratios=[4, 4], pos_shape=(batch_size, 10, 25), ) neck=dict( type='FPN', in_channels=[256, 512, 1024, 256], out_channels=64, num_outs=4, #trans_idx=-1, ) loss_weights=dict( hm_weight=1, kps_weight=0.4, row_weight=1., range_weight=1., ) num_lane_classes=1 heads=dict( type='CondLaneHead', heads=dict(hm=num_lane_classes), in_channels=(64, ), num_classes=num_lane_classes, head_channels=64, head_layers=1, disable_coords=False, branch_in_channels=64, branch_channels=64, branch_out_channels=64, reg_branch_channels=64, branch_num_conv=1, hm_idx=2, mask_idx=0, compute_locations_pre=True, location_configs=dict(size=(batch_size, 1, 80, 200), device='cuda:0') ) optimizer = dict(type='AdamW', lr=3e-4, betas=(0.9, 0.999), eps=1e-8) epochs = 16 total_iter = (88880 // batch_size) * epochs import math scheduler = dict( type = 'MultiStepLR', milestones=[8, 14], gamma=0.1 ) seg_loss_weight = 1.0 eval_ep = 1 save_ep = 1 img_norm = dict( mean=[75.3, 76.6, 77.6], std=[50.5, 53.8, 54.3] ) img_height = 320 img_width = 800 cut_height = 0 mask_down_scale = 4 hm_down_scale = 16 num_lane_classes = 1 line_width = 3 radius = 6 nms_thr = 4 img_scale = (800, 320) mask_size = (1, 80, 200) train_process = [ dict(type='Alaug', transforms=[dict(type='Compose', params=dict(bboxes=False, keypoints=True, masks=False)), dict( type='Crop', x_min=crop_bbox[0], x_max=crop_bbox[2], y_min=crop_bbox[1], y_max=crop_bbox[3], p=1), dict(type='Resize', height=img_scale[1], width=img_scale[0], p=1), dict( type='OneOf', transforms=[ dict( type='RGBShift', r_shift_limit=10, g_shift_limit=10, b_shift_limit=10, p=1.0), dict( type='HueSaturationValue', hue_shift_limit=(-10, 10), sat_shift_limit=(-15, 15), val_shift_limit=(-10, 10), p=1.0), ], p=0.7), dict(type='JpegCompression', quality_lower=85, quality_upper=95, p=0.2), dict( type='OneOf', transforms=[ dict(type='Blur', blur_limit=3, p=1.0), dict(type='MedianBlur', blur_limit=3, p=1.0) ], p=0.2), dict(type='RandomBrightness', limit=0.2, p=0.6), dict( type='ShiftScaleRotate', shift_limit=0.1, scale_limit=(-0.2, 0.2), rotate_limit=10, border_mode=0, p=0.6), dict( type='RandomResizedCrop', height=img_scale[1], width=img_scale[0], scale=(0.8, 1.2), ratio=(1.7, 2.7), p=0.6), dict(type='Resize', height=img_scale[1], width=img_scale[0], p=1),] ), dict(type='CollectLane', down_scale=mask_down_scale, hm_down_scale=hm_down_scale, max_mask_sample=5, line_width=line_width, radius=radius, keys=['img', 'gt_hm'], meta_keys=[ 'gt_masks', 'mask_shape', 'hm_shape', 'down_scale', 'hm_down_scale', 'gt_points' ] ), #dict(type='Resize', size=(img_width, img_height)), dict(type='Normalize', img_norm=img_norm), dict(type='ToTensor', keys=['img', 'gt_hm'], collect_keys=['img_metas']), ] val_process = [ dict(type='Alaug', transforms=[dict(type='Compose', params=dict(bboxes=False, keypoints=True, masks=False)), dict(type='Crop', x_min=crop_bbox[0], x_max=crop_bbox[2], y_min=crop_bbox[1], y_max=crop_bbox[3], p=1), dict(type='Resize', height=img_scale[1], width=img_scale[0], p=1)] ), #dict(type='Resize', size=(img_width, img_height)), dict(type='Normalize', img_norm=img_norm), dict(type='ToTensor', keys=['img']), ] dataset_path = './data/CULane' dataset = dict( train=dict( type='CULane', data_root=dataset_path, split='train', processes=train_process, ), val=dict( type='CULane', data_root=dataset_path, split='test', processes=val_process, ), test=dict( type='CULane', data_root=dataset_path, split='test', processes=val_process, ) ) workers = 12 log_interval = 1000 lr_update_by_epoch=True
Appreciate any response.
Is there any that I can modify the confidence score?
Regards, Ajay
Hello, Would you please share some tips to overcome detection failure with the following scenarios?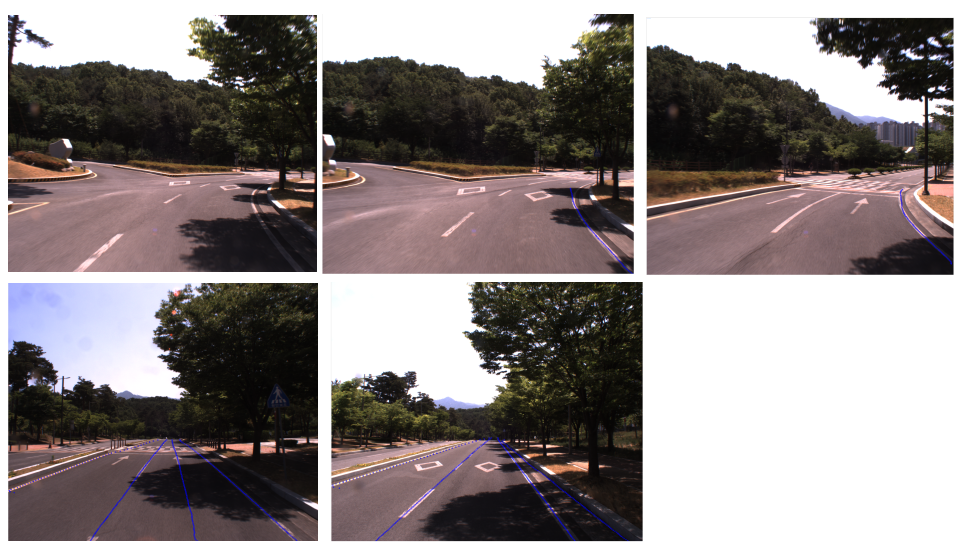
Issues;
I currently am using a pre-trained model: condlane_r101_culane.pth
With the configuration is as follows:
Appreciate any response.
Is there any that I can modify the confidence score?
Regards, Ajay