Open Wunder2dream opened 4 years ago
Reinforcement learning vs. supervised learning
Supervised learning is learning from a training set of labeled examples provided by a knowledgable external supervisor. Each example is a description of a situation together with a specification—the label—of the correct action the system should take in that situation, which is often to identify a category to which the situation belongs.
Reinforcement learning: In interactive problems it is often impractical to obtain examples of desired behavior that are both correct and representative of all the situations in which the agent has to act. In RL, an agent must be able to learn from its own experience.
RL vs. unsupervised learning
Unsupervised learning: is typically about finding structure hidden in collections of unlabeled data. The terms supervised learning and unsupervised learning would seem to exhaustively classify machine learning paradigms.
RL: Uncovering structure in an agent's experience can certainly be useful in reinforcement learning, but by itself does not address the reinforcement learning problem of maximizing a reward signal.
What is constrained RL? In this part, i am not sure. where i schould focus on. Because the canstraints in RL are so different in each case. For example, the paper https://arxiv.org/pdf/1812.02900.pdf focus on Batch-Constrained deep Q- Learning(BCQ).
Good summary and usable as a sound starting point for your further work :-) I'll come back to this tomorrow.
What is the difference between Forward view linear TD(Lambda) and Backward view linear ;ß -
TD(Lambda)?
TD(Lambda) vs. TD(0)
Coarse Coding 值函数逼近
[ ] DeepTraffic https://github.com/Wunder2dream/deep-traffic-2019 阅读测试
[ ] https://github.com/Wunder2dream/highway-env 用DeepQNetwork测试
[ ] 6.1 Derivative-Free Methods for Optimal Control
[ ] Open AI Safety Gym
n-step methods span a spectrum with MC methods at one end and one-step TD methods at the other. Conclusion: The best methods are often intermediate between the two extremes.
In many applications one wants to be able to update the action very fast to take into account anything that has changed, but bootstrapping works best if it is over a length of time in which a significant and recognizable state change has occurred. 在TD(0)中我们总是在单步观测之后,利用下一个状态的估计值来更新目标值,称之为单步自举。实际上,bootstrapping最好发生在一段时间之后。在这段时间内状态发生明显的变化。
Unified View of Reinforcement Learning
n-step TD prediction the first question: What is the space of methods lying between Monte Carlo and TD methods? 在MC和TD之间存在怎样的一个方法空间或者一类算法?
Let TD target look n steps into the future
The backup diagrams of n-step methods. These methods form a spectrum ranging from one-step TD methods to Monte Carlo methods.
target of update(更新目标) n-step updates are still TD methods because they still chagne an erlier estimate based on how it differs from a later estimate. Now the later estimate is not one step later, but n steps later.
(1)for MC:
the estimate of (St) is updated in the direction of the complete return:
(2)for TD(0)/on- step TD:
(3)for two-step TD updated based on two-step return:
(4)for n-step TD based on n-step return:
Notes:
If t + n >= T (if the n-step return extends to or beyond termination), then all the missing terms are taken as zero, and the n-step return defined to be equal to the ordinary full return
Note that n-step returns for n > 1 involve future rewards and states that are not available at the time of transition from t to t + 1. No real algorithm can use the n-step return until after it has seen Rt+n and computed Vt+n−1.
For example: n-step TD Methods on the Random Walk
我们使用n -step TD方法来估计一个随机行走问题的值.
通过grid search得到不同学习步长 alpha 和step n 对应的误差。可以看到当 n 取到中间值时,误差最小,再一次说明无论是MC还是TD(0),这种处于极端情况的方法,效果都不太好。
n-step Sarsa algorithm的更新公式为:
n-step Sarsa 的backup图如下:
![]()
![]()
n- step expected Sarsa
This algorithm can be described by the same equation as n-step Sarsa (above) except with the n-step return redefined as
同Sarsa和expected Sarsa的区别一样,我们只是将更新目标的最后一项换成期望值
如果s是terminal,它的期望是0
n-step Off-policy Learning
Recall that off-policy learning is learning the value function for one policy, pi, while following another policy, b.
Often, pi is the greedy policy for the current action-value function estimate, and b is a more exploratory policy, perhaps "epislon-greedy.
In order to use the data from b we must take into account the difference between the two policies, using their relative probability of taking the actions that were taken
the value function is,
Similarly, our previous n-step Sarsa update can be completely replaced by a simple off-policy form
注意两种情况下的 importance sampling ratio 的下标, 这是因为我们是在更新一个state-action pair,我们并不关心有多大的概率选中这个action,我们现在已经选中了它,importance sampling只是用于后续actions的选择。这个解释也让我理解了为什么Q-learning和Sarsa为什么没有使用importance sampling。
回想什么叫备份?通过后续状态的值更新当前状态值的操作,叫做备份。所有的更新操作都可以叫做备份,区别在于用那些状态备份,如何备份。
![]()
Hanging off to the sides of each state are the actions that were not selected. (For the last state, all the actions are considered to have not (yet) been selected.) Because we have no sample data for the unselected actions, we bootstrap and use the estimates of their values in forming the target for the update. In the tree-backup update, the target includes all these things plus the estimated values of the dangling action nodes hanging off the sides, at all levels. This is why it is called a treebackup update; it is an update from the entire tree of estimated action values.
Eligible trace(资格迹) - Chapter12 , which enable bootstrapping over multiple time intervals simultaneously.
Step:
What to Learn in Model-Free RL
some basic concepts
(In other words) it directly learns a policy which gives you decisions about which action to take in some state.
There are few approaches for solving these kind of problems
Monte-Carlo Policy Evaluation
There are two diferent types of MC Policy Evaluation
Monte-Carlo Control
Exploration/Exploitation trade off
How can they learn about the optimal policy while behaving according to an exploratory policy? 1)The on-policy approach in the preceding section is actually a compromise—it learns action values not for the optimal policy, but for a near-optimal policy that still explores. 2)The off-policy learning: Use two policies, one that is learned about and that becomes the optimal policy(target policy), and one that is more exploratory and is used to generate behavior(behavior policy)
Sarsa( state-action-reward-state-action): On-policy TD Control Sarsa is an on-policy TD control method. In the previous section we considered transitions from state to state and learned the values of states. Now we consider transitions from state–action pair to state–action pair, and learn the values of state–action pairs.
here the TD error
The backup diagram for Sarsa is as shown as below,
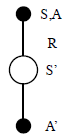
Q-Learning: Off- policy TD Control An off-policy TD control algorithm, defined by
the target policy(learned action-value function) directly aproximates , independent of the behaviour policy.
The backup diagramm for Q-learning is
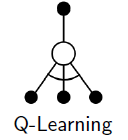
Expected Sarsa Similar to Q-learning Instead of using the maximum over next state–action pairs it uses the expected value, taking into account,
The backup diagramm for Q-learning is
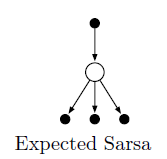
Frage: How can we understand the exploration start in MC control? How can we understand bootstrap in RL ? Is Backup the same as bootstrap?