Closed ghost closed 3 years ago
训练使用代码里默认的参数,推理使用1080*1920的图直接进行预测
NMS应该可以去掉吧
@gray2bgr 这个应该是训练或者推断的时候没有设置对.
@YonghaoHe config中下面的这几组数字是怎么计算得到的
param_feature_map_size_list = [159, 159, 79, 79, 39, 19, 19, 19]
param_bbox_small_list = [10, 15, 20, 40, 70, 110, 250, 400] assert len(param_bbox_small_list) == param_num_output_scales
param_bbox_large_list = [15, 20, 40, 70, 110, 250, 400, 560] assert len(param_bbox_large_list) == param_num_output_scales
@gray2bgr param_feature_map_size_list = [159, 159, 79, 79, 39, 19, 19, 19] 这个直接算feature map的变化就直接得到了,非常简单吧? param_bbox_small_list = [10, 15, 20, 40, 70, 110, 250, 400]和param_bbox_large_list = [15, 20, 40, 70, 110, 250, 400, 560]是根据检测任务和自己的需求来定的,目前通过经验来设置.
@YonghaoHe 明白了,谢谢
如图所示,人脸框上出现两个f分值很高的框,用阈值没法去掉,并且框也不太准,迭代了68W次,batch=96。请问这个情况是没训练好,还是训练参数没设置造成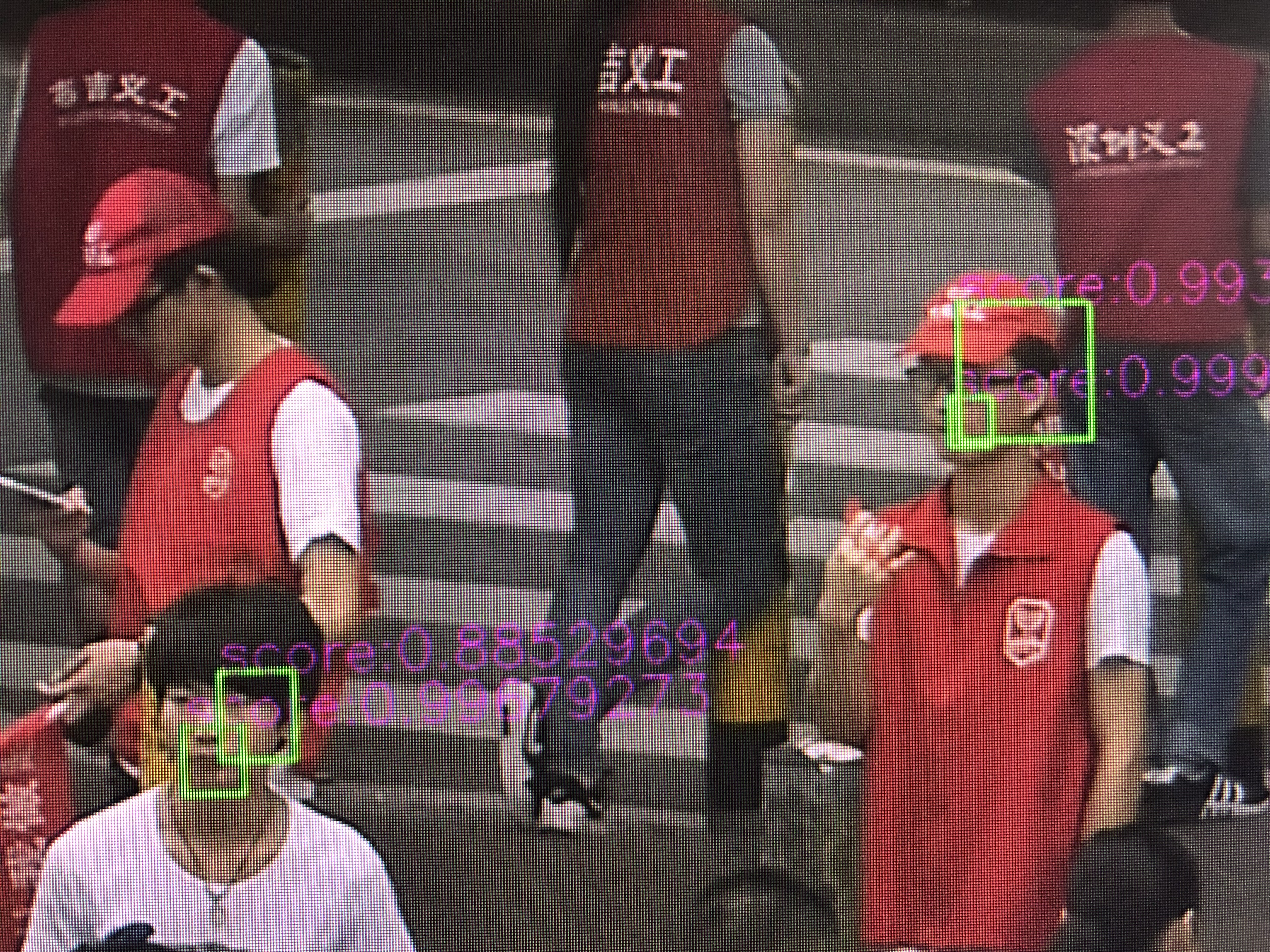