@inproceedings{mrksic-etal-2016-counter,
title = "Counter-fitting Word Vectors to Linguistic Constraints",
author = "Mrk{\v{s}}i{\'c}, Nikola and
{\'O} S{\'e}aghdha, Diarmuid and
Thomson, Blaise and
Ga{\v{s}}i{\'c}, Milica and
Rojas-Barahona, Lina M. and
Su, Pei-Hao and
Vandyke, David and
Wen, Tsung-Hsien and
Young, Steve",
booktitle = "Proceedings of the 2016 Conference of the North {A}merican Chapter of the Association for Computational Linguistics: Human Language Technologies",
month = jun,
year = "2016",
address = "San Diego, California",
publisher = "Association for Computational Linguistics",
url = "https://aclanthology.org/N16-1018",
doi = "10.18653/v1/N16-1018",
pages = "142--148",
}
1. What is it?
They propose a new postprocessing approach to consider antonymy.
2. What is amazing compared to previous works?
The previous postprocessing (#210) considers only a synonym relation.
That method and the "distributional hypothesis" make antonym words ("east" and "west") similar.
3. Where is the key to technologies and techniques?
Their method is based on three loss functions.
synonym attract (#210)
antonym rapel
vector preservation
Total loss is defined as below:
4. How did evaluate it?
Word similarity task: their method achieves state-of-the-art performance
5. Is there a discussion?
Benefits: their method can fix relations that the pre-trained model made a mistake in the past
0. Paper
@inproceedings{mrksic-etal-2016-counter, title = "Counter-fitting Word Vectors to Linguistic Constraints", author = "Mrk{\v{s}}i{\'c}, Nikola and {\'O} S{\'e}aghdha, Diarmuid and Thomson, Blaise and Ga{\v{s}}i{\'c}, Milica and Rojas-Barahona, Lina M. and Su, Pei-Hao and Vandyke, David and Wen, Tsung-Hsien and Young, Steve", booktitle = "Proceedings of the 2016 Conference of the North {A}merican Chapter of the Association for Computational Linguistics: Human Language Technologies", month = jun, year = "2016", address = "San Diego, California", publisher = "Association for Computational Linguistics", url = "https://aclanthology.org/N16-1018", doi = "10.18653/v1/N16-1018", pages = "142--148", }
1. What is it?
They propose a new postprocessing approach to consider antonymy.
2. What is amazing compared to previous works?
The previous postprocessing (#210) considers only a synonym relation. That method and the "distributional hypothesis" make antonym words ("east" and "west") similar.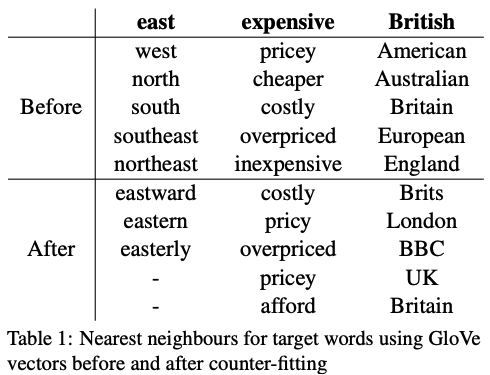
3. Where is the key to technologies and techniques?
Their method is based on three loss functions.
synonym attract (#210)
antonym rapel
vector preservation
Total loss is defined as below:
4. How did evaluate it?
Word similarity task: their method achieves state-of-the-art performance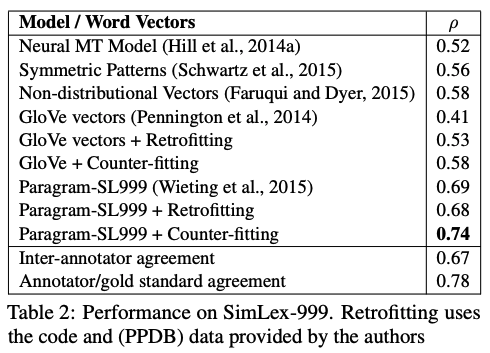
5. Is there a discussion?
Benefits: their method can fix relations that the pre-trained model made a mistake in the past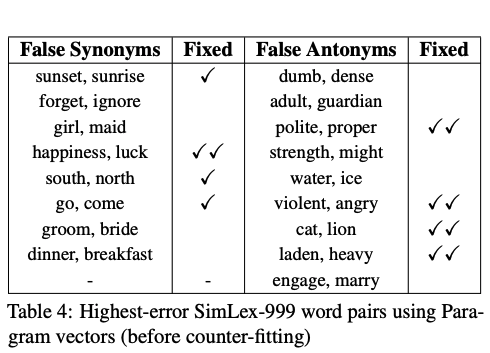
6. Which paper should read next?