Open aiegoo opened 1 year ago
This repository contains the example code from our O'Reilly book Natural Language Processing with Transformers:
You can run these notebooks on cloud platforms like Google Colab or your local machine. Note that most chapters require a GPU to run in a reasonable amount of time, so we recommend one of the cloud platforms as they come pre-installed with CUDA.
To run these notebooks on a cloud platform, just click on one of the badges in the table below:
Nowadays, the GPUs on Colab tend to be K80s (which have limited memory), so we recommend using Kaggle, Gradient, or SageMaker Studio Lab. These platforms tend to provide more performant GPUs like P100s, all for free!
Note: some cloud platforms like Kaggle require you to restart the notebook after installing new packages.
To run the notebooks on your own machine, first clone the repository and navigate to it:
$ git clone https://github.com/nlp-with-transformers/notebooks.git
$ cd notebooks
Next, run the following command to create a conda
virtual environment that contains all the libraries needed to run the notebooks:
$ conda env create -f environment.yml
Note: You'll need a GPU that supports NVIDIA's CUDA Toolkit to build the environment. Currently, this means you cannot build locally on Apple silicon 😢.
Chapter 7 (Question Answering) has a special set of dependencies, so to run that chapter you'll need a separate environment:
$ conda env create -f environment-chapter7.yml
Once you've installed the dependencies, you can activate the conda
environment and spin up the notebooks as follows:
$ conda activate book # or conda activate book-chapter7
$ jupyter notebook
This issue is likely due to a missing internet connection. When running your first notebook on Kaggle you need to enable internet access in the settings menu on the right side.
You can enable GPU usage by selecting GPU as Accelerator in the settings menu on the right side.
If you'd like to cite this book, you can use the following BibTeX entry:
@book{tunstall2022natural,
title={Natural Language Processing with Transformers: Building Language Applications with Hugging Face},
author={Tunstall, Lewis and von Werra, Leandro and Wolf, Thomas},
isbn={1098103246},
url={https://books.google.ch/books?id=7hhyzgEACAAJ},
year={2022},
publisher={O'Reilly Media, Incorporated}
}
Useful links found in the book
TransformersLibrary
Rnn
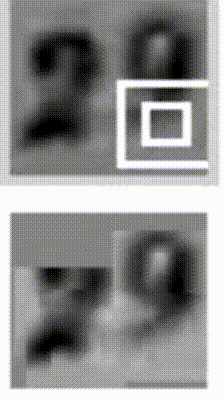
voice and speech recognition: article
Dataset korean hub
Aihub for test and train
Zeroth-korean github
Speech recognition is the ability for a device to recognize individual words or phrases from human speech. These words can be used to command the operation of a system -- computer menus, industrial controls or direct input of speech into an application -- as is the case with dictation software. Speech recognition systems can be speaker independent, typically with a limited vocabulary, or speaker dependent. The former is used when a limited vocabulary is expected to be used within a known context. The latter allows for greater vocabulary size, but at the cost of "training" the system for each specific user. This training typically consists of a user uttering a specific series of words and phrases so the system can learn the user's pronunciation techniques and speech patterns. It then creates a template specifically for each user.