Open capslocknanda opened 2 years ago
That should not be possible as the network was never trained on person keypoints. Can you explain the steps you are using.
Thank you for the fast reply Dinesh!
I actually solved that error but faced one more.
I am using your pre-trained model occlusion_net.pth but did not get a good prediction. the prediction looks like this,
Am I missing some scaling factor or setting in config default.py or in config yaml, please let me know.
I also train one model for 220000 iterations and got this result which is still not satisfactory.
Thank you in advance!
are you using the docker file provided or some other config file?
Hi Dinesh, I am using occlusion_net_test.yaml this config file from your repo.
MODEL: META_ARCHITECTURE: "GeneralizedRCNN" WEIGHT: "/models/occlusion_net.pth" BACKBONE: CONV_BODY: "R-50-FPN" RESNETS: BACKBONE_OUT_CHANNELS: 256 RPN: USE_FPN: True ANCHOR_STRIDE: (4, 8, 16, 32, 64) PRE_NMS_TOP_N_TRAIN: 2000 PRE_NMS_TOP_N_TEST: 1000 POST_NMS_TOP_N_TEST: 1000 FPN_POST_NMS_TOP_N_TEST: 1000 ROI_HEADS: USE_FPN: True
ROI_BOX_HEAD: POOLER_RESOLUTION: 7 POOLER_SCALES: (0.25, 0.125, 0.0625, 0.03125) POOLER_SAMPLING_RATIO: 2 FEATURE_EXTRACTOR: "FPN2MLPFeatureExtractor" PREDICTOR: "FPNPredictor" NUM_CLASSES: 2 KEYPOINT_ON: True ROI_KEYPOINT_HEAD: POOLER_SCALES: (0.25, 0.125, 0.0625, 0.03125) FEATURE_EXTRACTOR: "KeypointRCNNFeatureExtractor" PREDICTOR: "KeypointRCNNPredictor" POOLER_RESOLUTION: 14 POOLER_SAMPLING_RATIO: 2 RESOLUTION: 56 SHARE_BOX_FEATURE_EXTRACTOR: False NUM_CLASSES: 14 GRAPH_ON: True ROI_GRAPH_HEAD: FEATURE_EXTRACTOR: "graphRCNNFeatureExtractor" SHARE_BOX_FEATURE_EXTRACTOR: False KGNN2D: True DATASETS: TRAIN: ("keypoints_carfusion_test_cocostyle", ) TEST: ("keypoints_carfusion_train_cocostyle",) INPUT: MIN_SIZE_TRAIN: (640, 672, 704, 736, 768, 800) DATALOADER: SIZE_DIVISIBILITY: 32 SOLVER: IMS_PER_BATCH: 1 BASE_LR: 0.00025 WEIGHT_DECAY: 0.0001 STEPS: (480000, 640000) MAX_ITER: 220000 CHECKPOINT_PERIOD: 5000 TEST: IMS_PER_BATCH: 1
OUTPUT_DIR: "./log"
I am not sure why this is happening. seems like a change in the GPUs??. or may be a newer version of pytorch is causing this issue. Generally if you used the same docker file it should work well.
I also meet this bad result, and the prediction looks like this:
/home/gss/anaconda3/envs/Occlusion_Net/lib/python3.8/site-packages/apex/init.py:68: DeprecatedFeatureWarning: apex.amp is deprecated and will be removed by the end of February 2023. Use PyTorch AMP
warnings.warn(msg, DeprecatedFeatureWarning)
Using MLP graph encoder.
Using learned graph decoder.
Using MLP graph encoder.
./log/demo.jpg
2023-09-30_12-59-35
2023-09-30_12-59-35
Thank you for the fast reply Dinesh! I actually solved that error but faced one more. I am using your pre-trained model occlusion_net.pth but did not get a good prediction. the prediction looks like this,
Am I missing some scaling factor or setting in config default.py or in config yaml, please let me know. I also train one model for 220000 iterations and got this result which is still not satisfactory.
Thank you in advance!
I also meet this bad results, did you solve it?
Hi Dinesh, Thank you for sharing such nice work. Although while doing inference I noticed one anomaly (but maybe I am missing some settings). The model predicts PesonKeypoints even though the target is a car.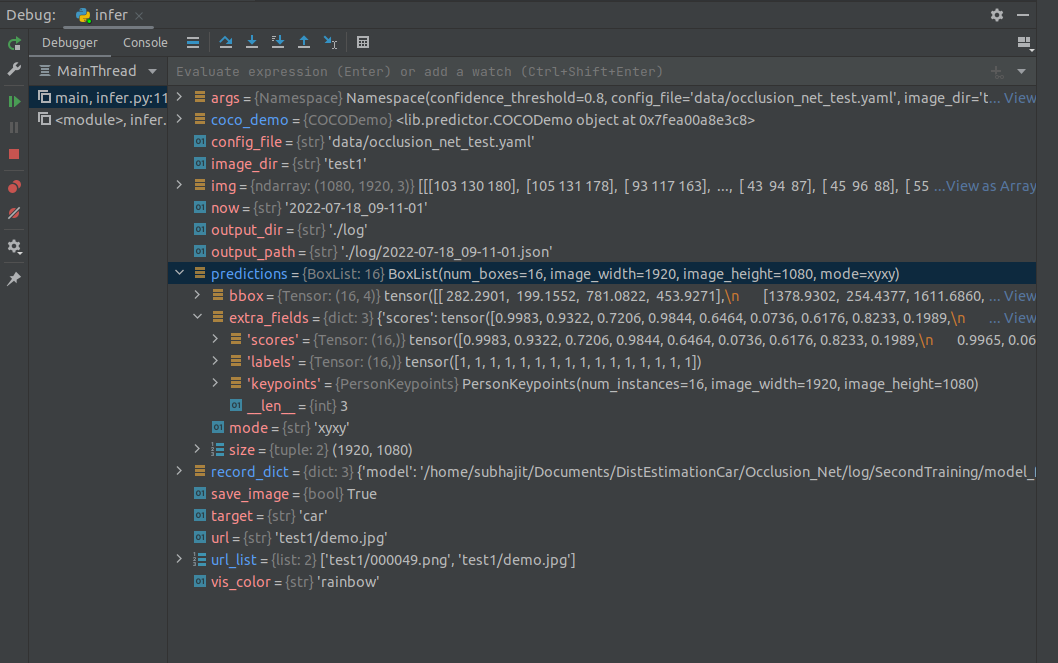