Open KathyGCY opened 1 year ago
I have a theory. I did more testing and realized this is very unique to LOGISTIC trend. In fact if I simply switch to linear trend, the MCMC vs non-MCMC results are more aligned.
Is this because when we MCMC, the cap / floor also participate in the simulation? Meaning that the cap for each simulated path might be slightly different? Would that explain the discrepancy?
FYI Here's the code for replication
import numpy as np
import pandas as pd
import matplotlib.pyplot as plt
# Parameters
num_days = 365
slope = 2
intercept = 100
weekly_amplitude = 50
# Time indices
time_indices = np.arange(num_days)
# Linear growth function
def linear_growth(t, m, b):
return m * t + b
# Weekly seasonality function
def weekly_seasonality(t, amplitude):
return amplitude * np.sin(2 * np.pi * t / 7)
# Generate time series data
trend = linear_growth(time_indices, slope, intercept)
seasonality = weekly_seasonality(time_indices, weekly_amplitude)
time_series = trend + seasonality
# Create a DataFrame with dates and values
dates = pd.date_range(start='2023-01-01', periods=num_days, freq='D')
data = pd.DataFrame({'date': dates, 'value': time_series})
# Extend the DataFrame for 365 more days
additional_dates = pd.date_range(start='2024-01-01', periods=365, freq='D')
additional_data = pd.DataFrame({'date': additional_dates, 'value': np.nan})
# Concatenate the two DataFrames
data = pd.concat([data, additional_data], ignore_index=True)
# Plot the time series
plt.figure(figsize=(10, 6))
plt.plot(data['date'], data['value'], label='Linear Trend with Weekly Seasonality')
plt.xlabel('date')
plt.ylabel('value')
plt.legend()
plt.show()
input_hp = {'changepoint_prior_scale': 0.05, 'changepoint_range': 0.8, 'growth': 'linear', 'seasonality_mode': 'additive', 'seasonality_prior_scale': 0.01, 'yearly_seasonality': True}
input_arg = {'forecast_length': 365,
'train_timestamp_col': "date",
'train_value_col': "value",
'reg_timestamp_col': None,
'reg_value_col': None,
"cap_timestamp_col": None,
"cap_value_col": None,
"floor_timestamp_col": None,
"floor_value_col": None,
'time_freq': "d"}
And you run through step 4 and 5 exactly the same with no code change.
Forecast-Interval: MCMC simulation results are shifted compared to non-MCMC results Here's a replicable code. Using prophet
pip install --upgrade --force-reinstall --no-cache prophet==1.0.1
RESULTS:
Green ones are the Here's the demo data
Here's the results: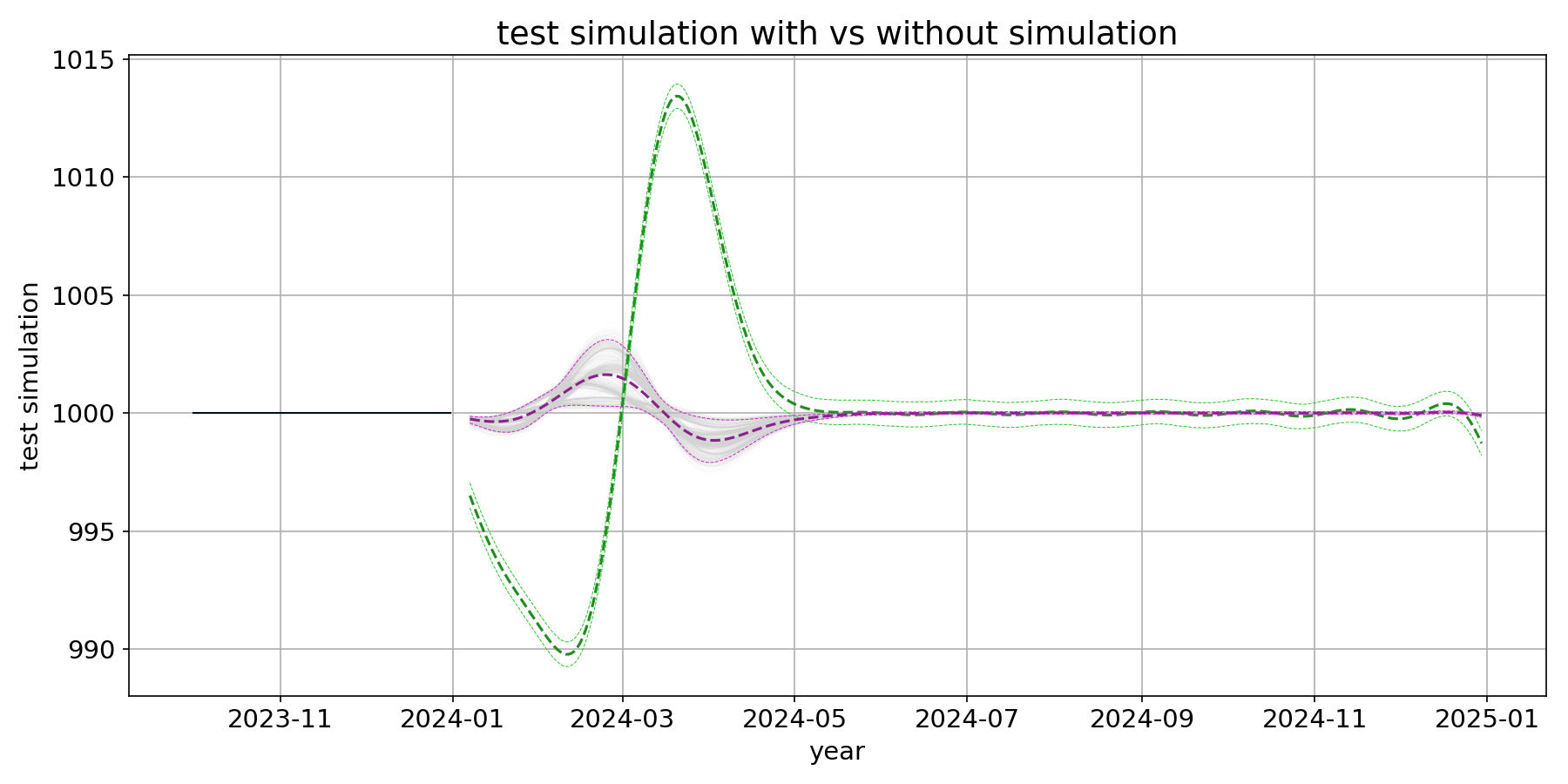
CODE:
Step 1 get the demo data
Step 2, Get Prophet set up
Step 3, Hyperparam and Arguments
Step 4, Run 2 versions, with and without MCMC
Step 5, Compare output