Open jroshanucb opened 3 years ago
Can anyone from the fairseq team address this question or point me to the appropriate code? Thanks.
Can anyone from the fairseq team address this question or point me to the appropriate code? Thanks.
In my opinion, the multi-head position attention in the NAT-DCRF paper just to follows the Gu et al. (2018). Actually, the CRF-based sequential decoding can tackle the reordering issue which the multi-head position attention wants to solve.
thank you. that make sense. I did made changes to my local instance of fairseq and introduced the positional attention layer to see if it impacts the BLEU score. With or without it, I was able to get to around 9 as the BLEU score. Were you able to replicate the 20+ score from the paper?
🐛 Bug: Opening a bug. But really, it is a question. In the NACRF implementation, (specifically the Fast Structured Decoding for Sequence Models paper implementation), I do not see the Multi-head positional attention in the decoder.
Please let me know if I have missed it or if it is embedded somewhere. Below is the model snapshot of the last layer of the decoder and Dynamic CRF. I can see self_attn and encoder attention layers only. (5): TransformerDecoderLayer( (dropout_module): FairseqDropout() (self_attn): MultiheadAttention( (dropout_module): FairseqDropout() (k_proj): Linear(in_features=512, out_features=512, bias=True) (v_proj): Linear(in_features=512, out_features=512, bias=True) (q_proj): Linear(in_features=512, out_features=512, bias=True) (out_proj): Linear(in_features=512, out_features=512, bias=True) ) (activation_dropout_module): FairseqDropout() (self_attn_layer_norm): LayerNorm((512,), eps=1e-05, elementwise_affine=True) (encoder_attn): MultiheadAttention( (dropout_module): FairseqDropout() (k_proj): Linear(in_features=512, out_features=512, bias=True) (v_proj): Linear(in_features=512, out_features=512, bias=True) (q_proj): Linear(in_features=512, out_features=512, bias=True) (out_proj): Linear(in_features=512, out_features=512, bias=True) ) (encoder_attn_layer_norm): LayerNorm((512,), eps=1e-05, elementwise_affine=True) (fc1): Linear(in_features=512, out_features=2048, bias=True) (fc2): Linear(in_features=2048, out_features=512, bias=True) (final_layer_norm): LayerNorm((512,), eps=1e-05, elementwise_affine=True) ) ) (layer_norm): LayerNorm((512,), eps=1e-05, elementwise_affine=True) (output_projection): Linear(in_features=512, out_features=10152, bias=False) (embed_length): Embedding(256, 512) ) (crf_layer): DynamicCRF( vocab_size=10152, low_rank=32, beam_size=64 (E1): Embedding(10152, 32) (E2): Embedding(10152, 32) ) ) 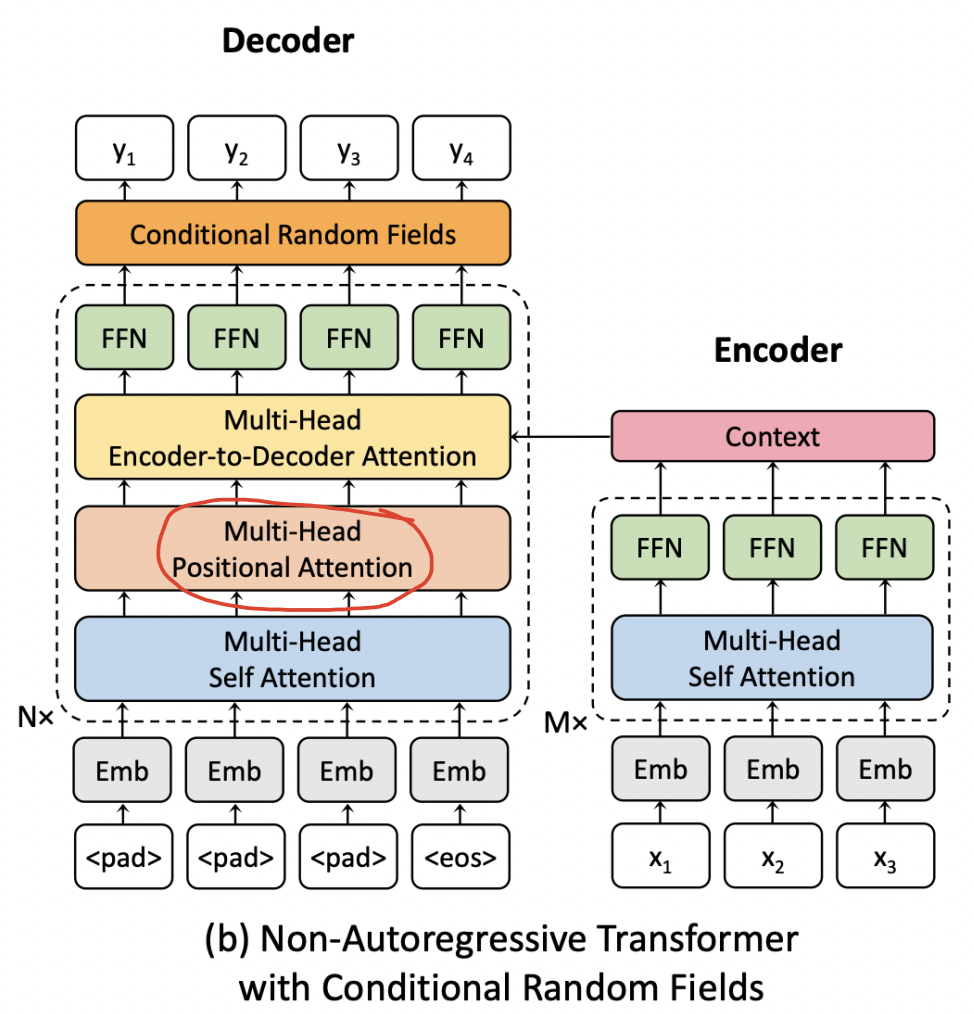 ### To Reproduce Steps to reproduce the behavior (**always include the command you ran**): 1. Run:  2. See errorCode sample
Expected behavior
Environment
pip
, source): pipAdditional context