Open felix-do-wizardry opened 2 years ago
It is kind of a shot in the dark as I don't have access to several GPUs. However, maybe this would help so I am posting it below.
You can see at the lines below that the learning rate is scaled with respect to batch size and "world size": https://github.com/facebookresearch/xcit/blob/82f5291f412604970c39a912586e008ec009cdca/main.py#L309-L310
I wonder if the world size is the number of GPUs. If true, maybe try to lower the learning rate or the batch size, so that the linearly scaled learning rate is lower.
Personally, I would check the value returned by world size with the debugger, and then try to lower the learning rate args.lr
, maybe divide it by 2 (or 4 since you have 4 GPU). Moreover, I think it makes sense if the training is unstable.
@woctezuma Thanks for the quick response,
I also saw that the learning rate is scaled linearly w.r.t the total batch size (batch-size * num-gpus) I can confirm that utils.get_world_size() does return the number of gpus I gave it to run (so 4 here)
I have tried lowering the lr by 4 times when training with 4 gpus, and got basically the same results (it did take about twice the epochs before the training becomes unstable with the lower lr)
Some other combinations of lr, batch size, and gpu count also did not work (e.g: default/lower lr with 2 gpus, default/lower lr with 8 gpus with same/lower batch-size)
@felix-do-wizardry Could you please share your logs file ?
@aelnouby Sorry, our server is currently down for a few days, I'll try to grab the log and get back to you as soon as I can, Thanks in advance.
@aelnouby hi, sorry for the delayed reply
Here's the log for a run with 4-gpu lr=5e-4 bs=128 https://gist.github.com/felix-do-wizardry/0a9b4bbd8fb0770ee3416209f19231a1
I have run the same command you used above:
CUDA_VISIBLE_DEVICES=0,1,2,3 python -m torch.distributed.launch --nproc_per_node=4 --use_env main.py \ --model xcit_small_12_p16 --batch-size 128 --drop-path 0.05 --epochs 400
The training seems to be behaving as expected, the logs are here: https://gist.github.com/aelnouby/540738cf88dda6a2fa5197915d1f2931
I am not sure where is the discrepancy. Could you try to re-run with a fresh clone of the repo and a fresh conda environment ?
Most of my (unstable) runs were done with a completely fresh clone of the repo, And I believe the conda environment is fresh from a docker build as well, But I'll try to double-check/re-run and report back, many thanks.
Hi, I'm trying to reproduce the classification training results.
I tried on 2 different machines, machine A with one RTX 3090 and machine B with four A100 gpus.
The training on machine A with a single GPU is fine; see green line (with default parameters). But on machine B with 4 gpus, it's not training properly and very erratic; see gray, yellow, teal lines (with default and custom parameters). Purple line is DeiT training on the same machine B (default parameters).
All experiments done with --batch-size=128 (128 samples per gpu).
This is validation loss, other metrics tell the same story, some even worse.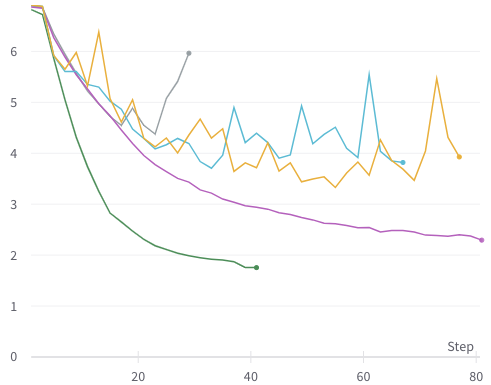
Example of the commands I used:
Anyone's seen this or know how to fix it? Many thanks.