Open noemi-mauro opened 5 years ago
Hi, I tested your configuration with 3.0.0 and here is my result:
It seems the result is consistent with the 1.3 version.
And here is my configuration:
# set data directory
dfs.data.dir=../data
# set result directory
# recommender result will output in this folder
dfs.result.dir=../result
# convertor
# load data and splitting data
# into two (or three) set
# setting dataset name
#data.input.path=ml-10M100k/ratings.dat
data.input.path=filmtrust/rating
#data.input.path=movielens/ml-1m/ratings.dat
#data.input.path=Gowalla/all.txt
# setting dataset format(UIR, UIRT)
data.column.format=UIRT
#data.convert.sep = ::
#data.convert.sep = \t
#data.convert.sep =
data.cache = true
# setting method of split data
# value can be ratio, loocv, given, KCV
data.model.splitter=kcv
data.splitter.cv.number=5
# using rating to split dataset
data.splitter.ratio=rating
# filmtrust dataset is saved by text
# text, arff is accepted
data.model.format=text
# the ratio of trainset
# this value should in (0,1)
data.splitter.trainset.ratio=0.8
# Detailed configuration of loocv, given, KCV
# is written in User Guide
# set the random seed for reproducing the results (split data, init parameters and other methods using random)
# default is set 1l
# if do not set ,just use System.currentTimeMillis() as the seed and could not reproduce the results.
rec.random.seed=1
# binarize threshold mainly used in ranking
# -1.0 - maxRate, binarize rate into -1.0 and 1.0
# binThold = -1.0, do nothing
# binThold = value, rating > value is changed to 1.0 other is 0.0, mainly used in ranking
# for PGM 0.0 maybe a better choose
data.convert.binarize.threshold=-1
# evaluation the result or not
rec.eval.enable=true
# specifies evaluators
# rec.eval.classes=auc,precision,recall...
# if rec.eval.class is blank
# every evaluator will be calculated
# rec.eval.classes=auc,precision,recall
# evaluator value set is written in User Guide
# if this algorithm is ranking only true or false
rec.recommender.isranking=false
#can use user,item,social similarity, default value is user, maximum values:user,item,social
#rec.recommender.similarities=user
Hello, I'm trying to reproduce the results reported in https://www.librec.net/release/v1.3/example.html for filmtrust (35k).
I started from the globalaverage algorithm and I run it with the following configuration: rec.recommender.class=globalaverage data.input.path=filmtrust data.column.format=UIR data.model.splitter=kcv data.splitter.cv.number=5 rec.random.seed=1 rec.recommender.isranking=false rec.learnrate.bolddriver=true rec.learnrate.decay=1.0
I run the following command: ./librec rec -exec -D rec.recommender.class=globalaverage -conf ../core/src/main/resources/rec/baseline/globalaverage-test.properties -libjars ../lib/log4j-1.2.17.jar
But I obtained the following results: 19/01/12 12:06:04 INFO RecommenderJob: Average Evaluation Result of Cross Validation: 19/01/12 12:06:04 INFO RecommenderJob: Evaluator value:MAE is 0.805817455750766 19/01/12 12:06:04 INFO RecommenderJob: Evaluator value:MPE is 1.0 19/01/12 12:06:04 INFO RecommenderJob: Evaluator value:RMSE is 0.994350785102186 19/01/12 12:06:04 INFO RecommenderJob: Evaluator value:MSE is 0.9887738589975241
In the link you reported these results: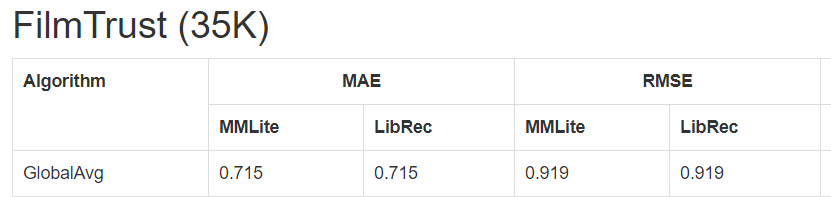
I did the same for the epinions dataset and I obtained different results as well. Can you help me, please? I don't know if I used different configurations from the ones that you used.
Thank you, Noemi