Closed kehuantiantang closed 3 years ago
I'll try to repeat your questions to make sure I understand what you're asking.
Hope that answers all of your questions. I will close this issue tomorrow if there are no follow-up questions :)
You solved all my questions,thank you very much for your answer.
Dear xksteven: Thank you so much for your work. I still confuse about detecting the anomalous class. As you said that we can evaluate how confident the model is on the predictions for the anomalous class or classes during testing (@xksteven in https://github.com/hendrycks/anomaly-seg/issues/17#), which can evalue the performance according to the evaluation metrics
auroc, aupr
. However,auroc, aupr
calculated by adjusting the threshold of confidence score.If we want to visualze the final anomaly segmentation result like 'Figure 4, prediction result', we should define a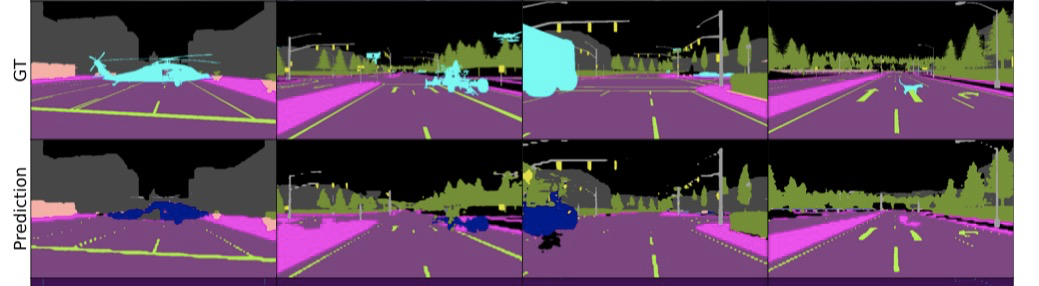
certain threshold
(for example, 0.5 for binary classification), is it means calculate the threshold value in test and use the same value for prediction ?Also, if we select the threshold Θ to split normal/anomaly, how to classify classes in in-distribution class ? Argmax ?
@xksteven in https://github.com/hendrycks/anomaly-seg/issues/20# , because model only train in in-distribution classes, so line 174 only visualize the mask(RGB) in in_distribution classes, how to visualize the out-distribtuion classes ? and 'MaxLogit' probability image (heatmap, anomaly score) drawed by conf?
Look forward to your reply, have a nice day, ^_^, thank you so much.