Closed jiaweiguan closed 1 year ago
Hello, the code performs lines 8-12 by sampling the noise in batches https://github.com/jasonkyuyim/se3_diffusion/blob/master/data/r3_diffuser.py#L134 Note that N is the length of the protein.
It seems to only appear in “eval_fn” for evaluation,not during training. Maybe I don't understand the paper yet. How does adding noise work during training?
Can you point to what you're referring to in the code?
Noise is added in these lines depending on whether it is training or inference.
https://github.com/jasonkyuyim/se3_diffusion/blob/master/data/pdb_data_loader.py#L210-L224
You can follow the callstack of forward_marginal
or sample_ref
to see how noise is added.
New noise is added each time we sample from the data loader.
Yes. self._diffuser.forward_marginal
adds noise for R_0 and X_0 . But the process of adding noise should be from t=0 to t=T.
https://github.com/jasonkyuyim/se3_diffusion/blob/master/data/pdb_data_loader.py#L210-L224
So you mean during inference? That is done here https://github.com/jasonkyuyim/se3_diffusion/blob/master/experiments/train_se3_diffusion.py#L670 Reverse is the denoising function that injects noise on each Euler-maruyama step. TimestepBatch is only used during training. Maybe that should be made clearer in the paper.
New noise is added each time we sample from the data loader.
It's a diffusion process.
In my opinion, diffusion requires multiple noises, adding noise should be a process, from t=0 to t=T.
But it seems only once here for train.
https://github.com/jasonkyuyim/se3_diffusion/blob/master/data/pdb_data_loader.py#L210-L224
I think there's a fundamental misunderstanding with how diffusion models are trained. We don't run simulation of p(xt | x{t-1}) starting from t=0 until we get to t. For gaussian and IGSO3, we have the heat kernel (or diffusion kernel) p(x_t | x_0) that we can directly sample from. This only requires sampling noise once during training. This noise is appropriately scaled depending on the time t ~ U(eps, 1) that we sample.
Ok, thanks your time for my question!
Thanks for the question! To add more detail, the closed form heat kernel p(x_t | x_0) is not available for every manifold one might want to model. If it is not available then one does have to run simulation by sampling multiple noises (for instance a Geodesic Random Walk (GRW) Algorithm 1 in De bortoli et al.). We are just lucky that a closed form exists for SO(3).
Hi! In the code, I found that diffusion only adds noise once. How to realize multiple times and then denoise? Screenshot from the original paper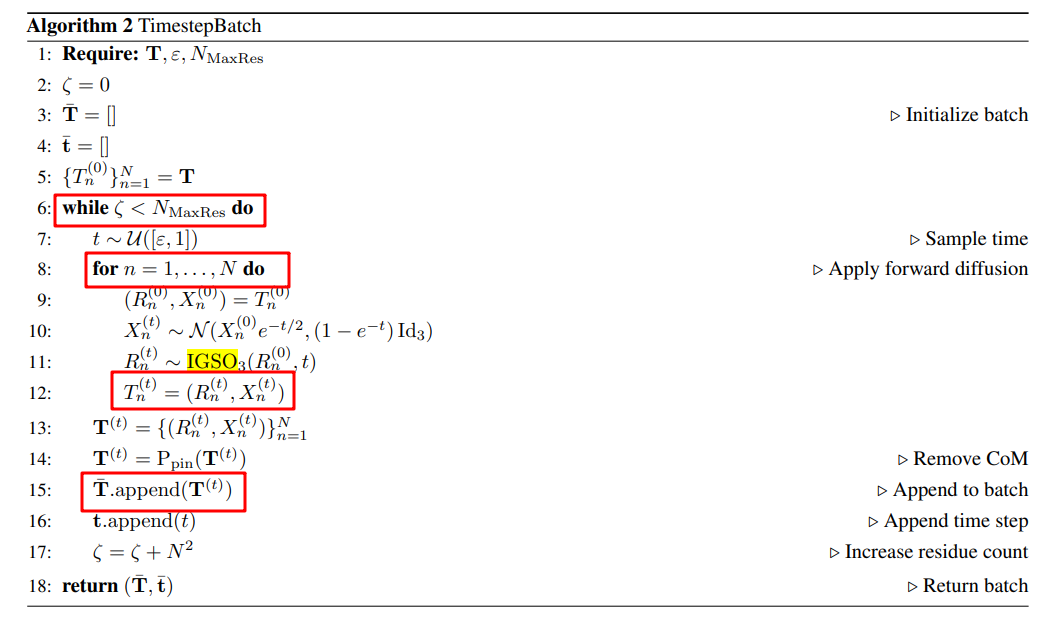