Closed catacopil closed 4 years ago
That’s weird. I don’t think you’re doing something wrong. Let me look into it more. You might want to pull the most recent changes and build it yourself, because I think I fixed some of the issues you’re seeing here since the last release.
The reason you needed to switch to a string key is because, when Bayesian networks are parallelized, they get serialized to disk and then loaded up in each process. However, there was a bug where serialization and deserialization of Bayesian networks accidentally caused the keys to get stringified. I think that has since been fixed.
On Tue, Dec 18, 2018 at 9:09 AM Cătălin Copil notifications@github.com wrote:
Hello everyone, I started using pomegranate after a long search for some Python packages that implements Bayesian Networks. Unfortunatelly, after discovering the opportunity to parallelize the execution of predict_proba() with n_jobs, I discovered there is a bug that cause estimating the same distribution of probability for different combination of nodes.
My network is quite simple, as you can see the schema below: [image: bayes net for issue] https://user-images.githubusercontent.com/3440454/50169139-433bad80-02ed-11e9-96c3-3e722d69d1ee.png
I use this network to understand the probability that some customers have to use my service during a specific day of week, during a specific moment of that day. After fixing day_of_week and time_slot, I query the distribution for each customer and then I sum each probability in order to estimate the number of customers that will use my service during a specific time.
Here is my code, I also attached a dataset to try it. Customers usages and not - issue dataset.zip https://github.com/jmschrei/pomegranate/files/2691475/Customers.usages.and.not.-.issue.dataset.zip Use the use_joblib variable to switch between using or not the parameter n_jobs `
import pandas as pd import time, datetime import pomegranate as pg import random
pd.options.display.max_rows = 999 pd.set_option('display.max_columns', 500) pd.set_option('display.width', 1000)
Creating Bayes Net simple structure
def simple_structure(): usage_tuple = () day_of_week_tuple = (0, ) time_slot_tuple = (0, ) customer_tuple = (0, )
tuples = (usage_tuple, day_of_week_tuple, time_slot_tuple, customer_tuple) return tuples
print("\n ---------- PACKAGE VERSIONS ---------- \n") for p in [pg, pd]: print(p.name, p.version)
filename = 'Customers usages and not - issue dataset.csv' customers = [123, 234, 345, 456, 567, 678, 789, 890] use_joblib = True
dtypes = {'Customer': 'int', 'TimeSlot': 'int', 'Date': 'str', 'Usage': 'int', 'DayOfWeek': 'int'} df_merged = pd.read_csv(filename, index_col='index', dtype=dtypes, parse_dates=['Date'])
print("\n ---------- CREATING BAYES NET ---------- \n")
Selecting the feature I use in Bayes Net
df_bayes_net = df_merged[['Usage', 'DayOfWeek', 'TimeSlot', 'Customer']]
BNet = pg.BayesianNetwork("Customer Bayes Net") my_structure = simple_structure() BNet = BNet.from_structure(X=df_bayes_net.values, structure=my_structure, state_names=df_bayes_net.columns.tolist()) print('Structure of Network: ', BNet.structure)
print("\n ---------- QUERY ON THE NET ---------- \n") preferred_days = [3] preferred_hours = [9]
for d in preferred_days: for h in preferred_hours: startHour = time.perf_counter() to_predict = [] for c in customers: to_predict.append([None, d, h, c])
pred_cust = None if use_joblib: pred_cust = BNet.predict_proba(to_predict, n_jobs=4) else: pred_cust = BNet.predict_proba(to_predict) i = 0 tot_prob = 0 for c in customers: values = pred_cust[i] distrib = values[0] usage_vals = distrib.parameters[0] usage_one = None if use_joblib: usage_one = usage_vals["1"] # I do not understand why I must use the string key when I parallelize the execution else: usage_one = usage_vals[1] print("Customer ", c, " has probability of usage = ", usage_one) # I sum the probabilities of usage for each customer tot_prob += usage_one i += 1 print("TOTAL Probability for [Usage = 1, DayOfWeek = ", d, ", TimeSlot= ", h, ", all customers]: ", tot_prob)
`
Output with use_joblib = True ` ---------- QUERY ON THE NET ----------
Customer 123 has probability of usage = 0.510997982902699 Customer 234 has probability of usage = 0.510997982902699 Customer 345 has probability of usage = 0.510997982902699 Customer 456 has probability of usage = 0.510997982902699 Customer 567 has probability of usage = 0.510997982902699 Customer 678 has probability of usage = 0.510997982902699 Customer 789 has probability of usage = 0.510997982902699 Customer 890 has probability of usage = 0.510997982902699 TOTAL Probability for [Usage = 1, DayOfWeek = 3 , TimeSlot= 9 , all customers]: 4.087983863221592`
Output with use_joblib = False ` ---------- QUERY ON THE NET ----------
Customer 123 has probability of usage = 0.4422745917574053 Customer 234 has probability of usage = 0.1992400366060012 Customer 345 has probability of usage = 0.224246853703186 Customer 456 has probability of usage = 0.5513281924572804 Customer 567 has probability of usage = 0.5706562322740955 Customer 678 has probability of usage = 0.3364213952359427 Customer 789 has probability of usage = 0.5911750759976838 Customer 890 has probability of usage = 0.47006766541644274 TOTAL Probability for [Usage = 1, DayOfWeek = 3 , TimeSlot= 9 , all customers]: 3.385410043448038`
I am missing something? Does anyone had the same problem? If my code is not so clear just ask. Thanks in advance for any suggestion.
— You are receiving this because you are subscribed to this thread. Reply to this email directly, view it on GitHub https://github.com/jmschrei/pomegranate/issues/530, or mute the thread https://github.com/notifications/unsubscribe-auth/ADvEEEu2kM0-tmaTmzAYpHzRBAPVsB9Oks5u6SFMgaJpZM4ZYuKL .
I get exactly the same problem with the newest version from pip. If I use n_jobs > 1 it predicts always the same value / probability.
The same problem
Hi! I am new to open source but quite well versed with stats...if this issue is still open, I would like to work on it. Thanks!
Obviously I haven't been able to get to this issue yet, but I have noted that if you set all of your data to strings, that parallelism appears to work correctly. This is likely due to a bug in how bayesian networks are serialized, and because parallelizing the predictions involved first serializing the model and then reading it in with each core.
Ultimately, the issue had to do with serializing JSONs, because when you read a JSON the package doesn't know whether the number (which now appears as a string) is a string or an int or a float or whatever so it just casts it as a string. The way parallel processing works in pomegranate is that a model is serialized and then read in to each of the workers. These workers now had versions of the model that thought all the numbers were strings. I thought I fixed this a little bit ago but the issue was complicated by numpy data types being separate from Python ones, where numpy.int64 != int.
tl;dr this, and all other issues related to Bayesian network serialization, should be completely resolved in 0.12.0.
Hello everyone, I started using pomegranate after a long search for some Python packages that implements Bayesian Networks. Unfortunatelly, after discovering the opportunity to parallelize the execution of predict_proba() with n_jobs, I discovered there is a bug that cause estimating the same distribution of probability for different combination of nodes.
My network is quite simple, as you can see the schema below: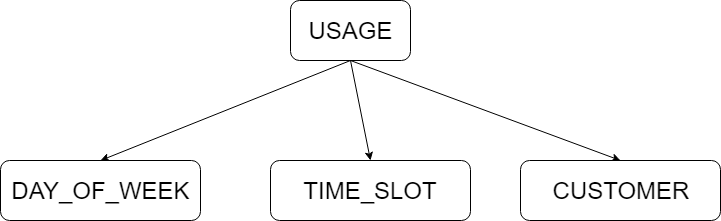
I use this network to understand the probability that some customers have to use my service during a specific day of week, during a specific moment of that day. After fixing day_of_week and time_slot, I query the distribution for each customer and then I sum each probability in order to estimate the number of customers that will use my service during a specific time.
Here is my code, I also attached a dataset to try it. Customers usages and not - issue dataset.zip Use the use_joblib variable to switch between using or not the parameter n_jobs `
`
Output with use_joblib = True ` ---------- QUERY ON THE NET ----------
Customer 123 has probability of usage = 0.510997982902699 Customer 234 has probability of usage = 0.510997982902699 Customer 345 has probability of usage = 0.510997982902699 Customer 456 has probability of usage = 0.510997982902699 Customer 567 has probability of usage = 0.510997982902699 Customer 678 has probability of usage = 0.510997982902699 Customer 789 has probability of usage = 0.510997982902699 Customer 890 has probability of usage = 0.510997982902699 TOTAL Probability for [Usage = 1, DayOfWeek = 3 , TimeSlot= 9 , all customers]: 4.087983863221592`
Output with use_joblib = False ` ---------- QUERY ON THE NET ----------
Customer 123 has probability of usage = 0.4422745917574053 Customer 234 has probability of usage = 0.1992400366060012 Customer 345 has probability of usage = 0.224246853703186 Customer 456 has probability of usage = 0.5513281924572804 Customer 567 has probability of usage = 0.5706562322740955 Customer 678 has probability of usage = 0.3364213952359427 Customer 789 has probability of usage = 0.5911750759976838 Customer 890 has probability of usage = 0.47006766541644274 TOTAL Probability for [Usage = 1, DayOfWeek = 3 , TimeSlot= 9 , all customers]: 3.385410043448038`
I am missing something? Does anyone had the same problem? If my code is not so clear just ask. Thanks in advance for any suggestion.