Open JNDanielson opened 3 years ago
Ok, I figured out a better solution to figure out the mean vector mean_vec. This uses the scatter matrix approach from Directional Statistics (Jupp and Mardia, 2000, pp.165).
import scipy.linalg as la
import numpy as np
# strikes, dips are variables containing orientations for the distribution of poles
lons, lats = mpl.stereonet_math.pole(np.mod(strikes,360),(dips))
x, y, z = mpl.stereonet_math.sph2cart(lons, lats)
xyz = np.hstack((x,y,z))
xyz = np.array(xyz).T
# xbar is the sample mean vector. This won't be accurate for vectors that point in opposite directions.
xbar = xyz.mean(axis=1)
# xobar is the sample mean direction
Rbar = np.linalg.norm(xbar)
xobar = xbar/Rbar
xobar = xobar.reshape(3,1)
# n is number of poles in the distribution
n = xyz.shape[1]
# initialize the scatter matrix T
T = np.zeros((3,3))
# Equation 9.2.10 from Directional Statistics (Jupp and Mardia, 2000, pp.165)
for i in range(n):
T += (xyz[:,i].reshape(3,1)).dot((xyz[:,i].reshape(3,1)).T)
T = T/n
# Mean vector of distribution is one of the eigenvectors of the scatter matrix T
eigs = la.eigh(T)
mean_vec = eigs[1][:,2]
mean_pole = mpl.stereonet_math.vector2pole(mean_vec[1],mean_vec[2],-1*mean_vec[0])
### print results to test
calculated_strike = mean_pole[0]
calculated_dip = mean_pole[1]
print(calculated_strike)
print(calculated_dip)
I'll figure out how to make a pull request, but in the meantime here's a working update to the fisher_stats function that fixes the issues with mean orientation and kappa.
def fisher_stats(lons, lats, conf=95):
"""
Returns the resultant vector from a series of longitudes and latitudes. If
a confidence is set the function additionally returns the opening angle
of the confidence small circle (Fisher, 19..) and the dispersion factor
(kappa).
Parameters
----------
lons : array-like
A sequence of longitudes (in radians)
lats : array-like
A sequence of latitudes (in radians)
conf : confidence value
The confidence used for the calculation (float). Defaults to None.
Returns
-------
mean vector: tuple
The point that lies in the center of a set of vectors.
(Longitude, Latitude) in radians.
If 1 vector is passed to the function it returns two None-values. For
more than one vector the following 3 values are returned as a tuple:
r_value: float
The magnitude of the resultant vector (between 0 and 1) This represents
the degree of clustering in the data.
angle: float
The opening angle of the small circle that corresponds to confidence
of the calculated direction.
kappa: float
A measure for the amount of dispersion of a group of layers. For
one vector the factor is undefined. Approaches infinity for nearly
parallel vectors and zero for highly dispersed vectors.
"""
xyz = sph2cart(lons, lats)
xyz = np.vstack(xyz)
# xbar is the sample mean vector
xbar = xyz.mean(axis=1)
# xobar is the sample mean direction
r_value = np.linalg.norm(xbar)
xobar = xbar/r_value
xobar = xobar.reshape(3,1)
num = xyz.shape[1]
# Equation 9.2.10 from Directional Statistics (Jupp and Mardia, 2000, pp.165)
T = np.zeros((3,3))
for i in range(num):
T += (xyz[:,i].reshape(3,1)).dot((xyz[:,i].reshape(3,1)).T)
T = T/num
# Mean vector of distribution is one of the eigenvectors of the scatter matrix T
eigs = la.eigh(T)
mean_vec = eigs[1][:,2]
if num > 1:
p = (100.0 - conf) / 100.0
r_value = np.sqrt(eigs[0].max())
vector_sum = mean_vec*num*r_value
result_vect = np.sqrt(np.sum(np.square(vector_sum)))
fract1 = (num - result_vect) / result_vect
fract3 = 1.0 / (num - 1.0)
angle = np.arccos(1 - fract1 * ((1 / p) ** fract3 - 1))
angle = np.degrees(angle)
kappa = (num - 1.0) / (num - result_vect)
mean_vec = cart2sph(*mean_vec)
return mean_vec, (r_value, angle, kappa)
else:
return None, None
Hi Joe, The find_fisher_stats, fisher_stats, and mean_vector functions don't seem to be calculating Fisher k (kappa) or the mean vector of a group of poles correctly where the group of poles includes orientations which cross a dip=90°.
For example, the Fisher K and mean vector for this group of poles is correct. Calculated mean dip is 50°, Fisher K is 50.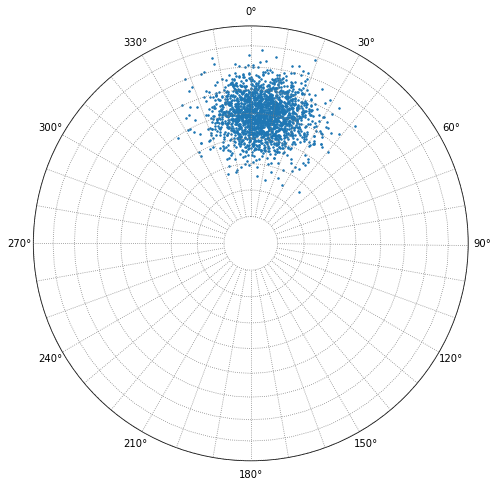
But, for this example the distribution has an actual Fisher k value of 25 and a mean dip of 85°, but the calculated kappa is 1.6 and the calculated mean dip is 62°.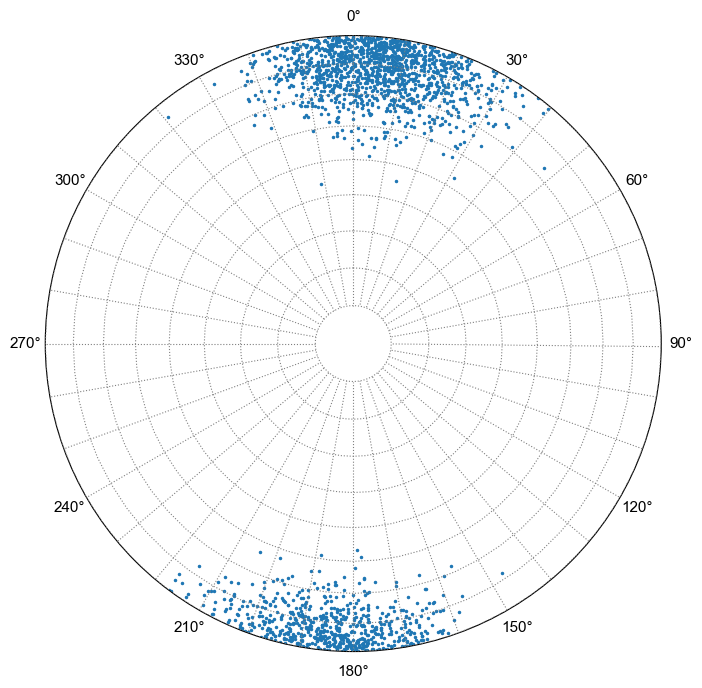
I think the issue is the lines of code where you take the mean of the vectors. xyz = np.vstack(xyz).T mean_vec = xyz.mean(axis=0)
When the largest eigenvalue flips direction, just taking the mean gives you a bad result.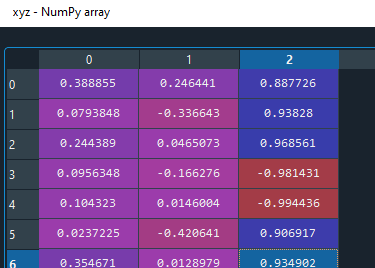
As a hack/workaround, I think you can do something like flip the normals so they all point in the same direction: le = np.argmax(np.max(np.abs(xyz), axis=0)) # largest eigenvalue is the one we should normalize the others by cond = xyz[:,le]<0 xyz = np.where(cond[:,None],xyz*-1,xyz)
But I bet you can come up with a better solution. This library is awesome, thanks for all your work on it!