import pandas as pd
from pandas_ods_reader import read_ods
import json
import matplotlib.pyplot as plt
import seaborn as sns
import numpy as np
from numpy import linalg
from numpy.linalg import svd
from collections import Counter
from mpl_toolkits.mplot3d import Axes3D
import random
import scipy as sp
# === === Helper functions === ===
def write_json(data, filename):
with open(filename,"w") as f:
json.dump(data,f)
return None
def get_json(filename):
with open(filename) as f:
jsonobj = json.load(f)
return jsonobj
def add_character_name(row,character_map):
return tuple(character_map[character_map['ID'] == row['unnamed.1']]['Character display name'])[0]
def add_work_name(row,character_map):
return tuple(character_map[character_map['ID'] == row['unnamed.1']]['Fictional work'])[0]
#=================================
df_bap = pd.read_json("July2021_df_bap.json")
df_traits = pd.read_json("July2021_df_traits.json")
df_std = pd.read_json("June2021_df_std_original.json")
df_n = pd.read_json("June2021_df_n_original.json")
clean_column_dict = get_json("July2021_cleaned_column_dict.json")
def runSVD(df1,dropcols=['unnamed.1','name','work'],n=None):
if len(dropcols) > 0:
for x in dropcols:
if x in df1.columns:
df1 = df1.drop(x,axis=1)
if n==None:
n=df1.shape[1]-1
X = df1.to_numpy()
#decompose
U, D, V = np.linalg.svd(X)
# get dim of X
M,N = X.shape
# Construct sigma matrix in SVD (it simply adds null row vectors to match the dim of X)
Sig = sp.linalg.diagsvd(D,M,N)
# Now you can get X back:
remakeX = np.dot(U, np.dot(Sig, V))
assert np.sum(remakeX - X) < 0.00001
return df1, U, D, V, Sig, X, remakeX
# Output from SVD without removing means
df1, U, D, V, Sig, X, remakeX = runSVD(df_traits)
# Remove the average of each trait
#df1_means = df1.mean().mean()
df1_means = 50
df1_normed = df1 - df1_means
# Output from SVD WITH removing means
df2, U2, D2, V2, Sig2, X2, remakeX2 = runSVD(df1_normed,dropcols=[])
# When remaking X, Sig2 and V2 are combined, then their product is combined with U2, so here is that first product
SigV2 = np.dot(Sig2,V2)
# the traits in order of columns
col2 = df2.columns
def get_chars_with_at_least_min_n_ratings_per_trait(df_n,df,
n=0,
verbose=True,
chart=True):
df_n = df_n.drop('unnamed.1',axis=1)
df_n['min']=df_n.apply(lambda row: min(row), axis=1)
if verbose:
print("df_n: ",df_n.shape)
#print("Avg min: ",sum(df_n['min'])/len(df_n['min']))
#print("Std for n: ",np.std(df_n['min']))
if chart:
# matplotlib histogram
plt.hist(df_n['min'], color = 'blue', edgecolor = 'black',
bins = 100)
# Add labels
plt.title('Histogram of min per trait per char')
plt.xlabel('cells of df_n')
plt.ylabel('number')
#select characters in self.df who have min ratings >= n for all cols in self.coldict
newchars = pd.DataFrame()
for chari in range(df_n.shape[0]):
if df_n.iloc[chari].min() >= n:
newchars = newchars.append(df.iloc[chari])
if verbose:
print("newdf: ",newchars.shape)
print(newchars.head())
return newchars
df_min_n = get_chars_with_at_least_min_n_ratings_per_trait(df_n,df2)
def vector_barchart(vector_names,vector,n,style="by_mag",ascending=False):
""" vector_names should be the labels for the values in the vector
vector should be the vector (ndarray)
n should be the number of values you want displayed in the chart
style should be the format of the chart
ascending=False will be most relevant traits by magnitude,
ascending=True will be least relevant traits by magnitude"""
n=min(n,len(vector_names))
vectordf = pd.DataFrame()
vectordf["Trait"] = vector_names
vectordf["Values"] = vector
if style=="by_mag":
vectordf["Magnitude"] = vectordf.apply(lambda row: abs(row["Values"]), axis = 1)
sorteddf = vectordf.sort_values(by="Magnitude",ascending=ascending)
#plotguy = sorteddf.iloc[-2*n:].iloc[::-1]
plotguy = sorteddf.iloc[0:2*n]
# if side=="half_n_half":
# sorteddf = lincombos3d.sort_values(by=d)
# top_n_top = sorteddf.iloc[0:n]
# top_n_bottom = sorteddf.iloc[-n:].iloc[::-1]
# plotguy = pd.concat([top_n_top,top_n_bottom])
# if side=="neg":
# plotguy = sorteddf.iloc[0:2*n]
# if side=="pos":
# plotguy = sorteddf.iloc[-2*n:].iloc[::-1]
#print(plotguy)
#sns.set(font_scale = 2)
sns.barplot(plotguy["Values"],plotguy["Trait"])
#sns.set(font_scale = 1)
return vectordf, plotguy
If 10 ratings are required for each trait for each character in order for that character to be included in the matrix, then: newdf: (560, 236)
First dimension (first row of V^T)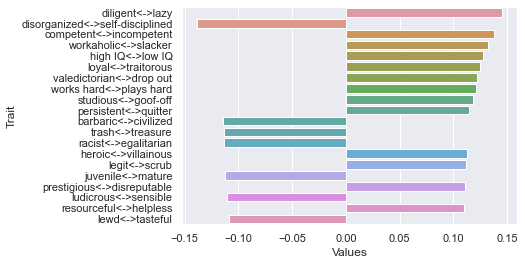
Second dimension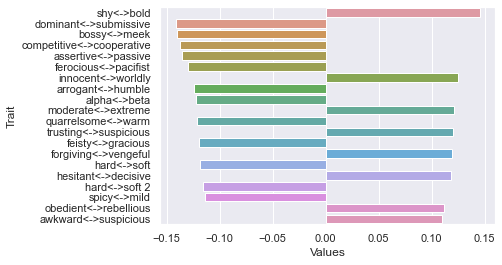
Third dimension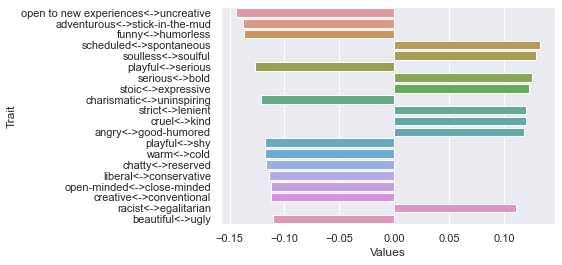
Fourth dimension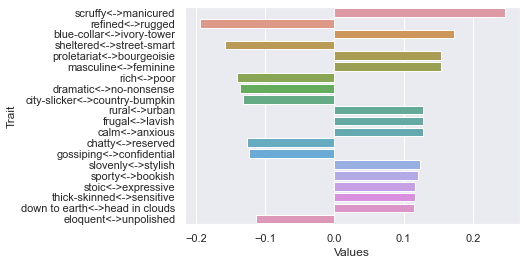
Fifth dimension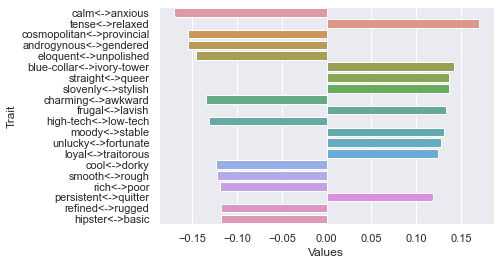
Sixth dimension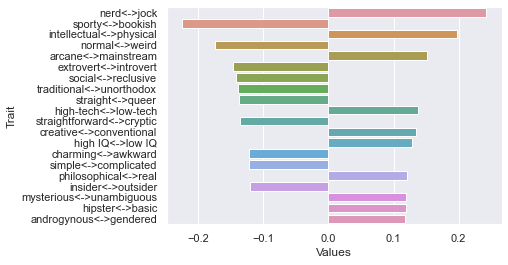
Seventh dimension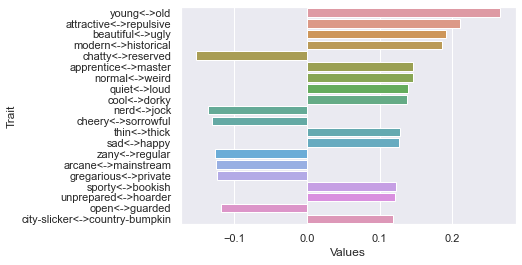
Eighth dimension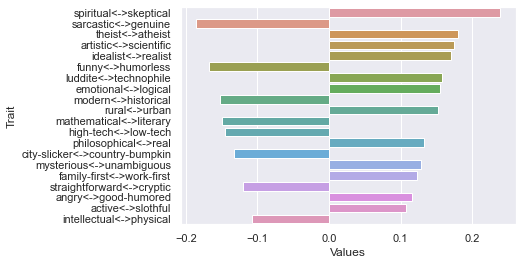
Ninth dimension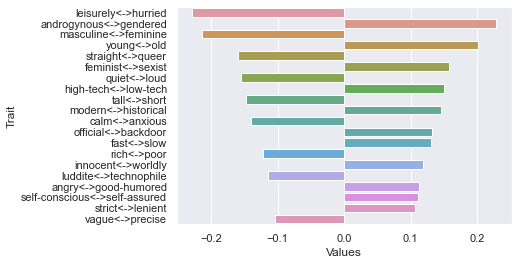
Tenth dimension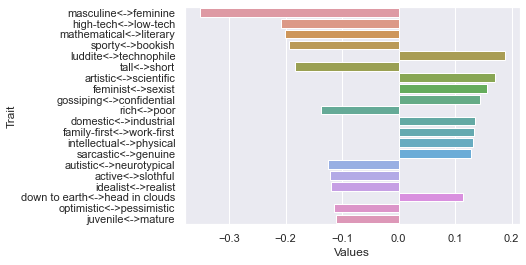
20 rating threshold: newdf: (436, 236)
First dimension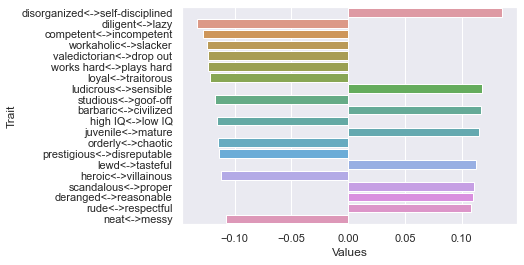
Second dimension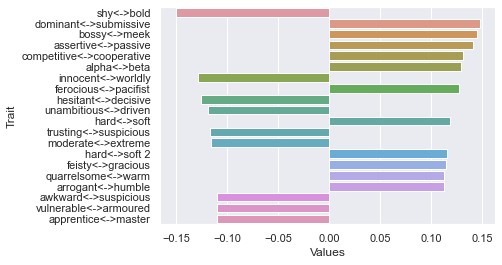
Third dimension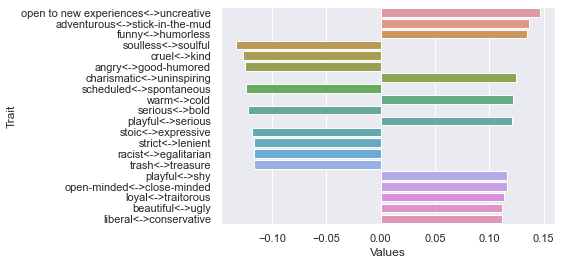
Fourth dimension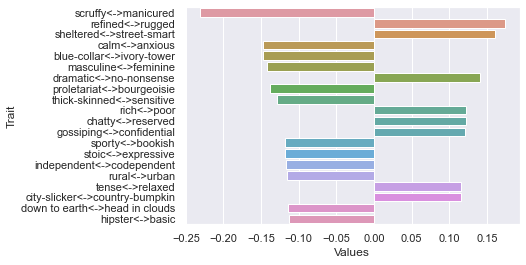
Fifth dimension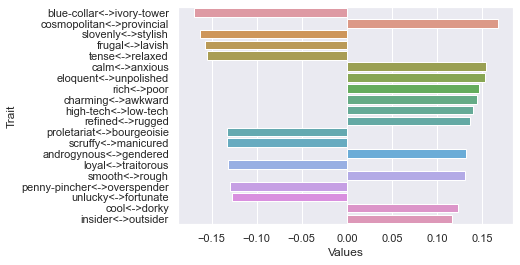
Sixth dimension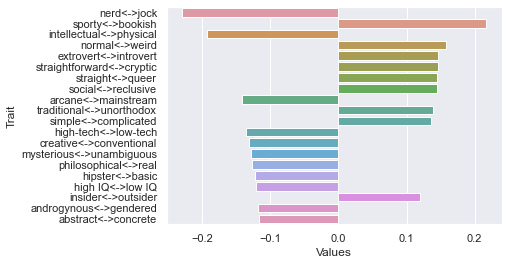
Seventh dimension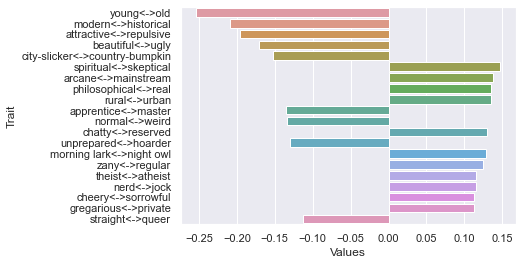
Eighth dimension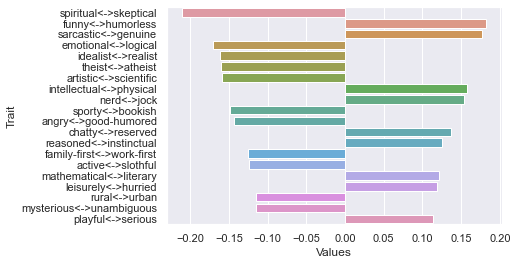
Ninth dimension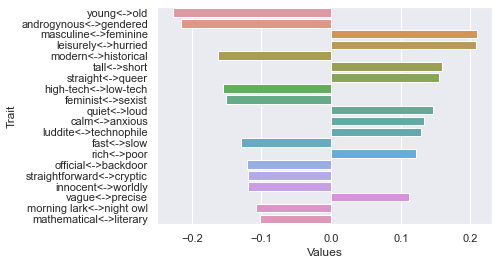
Tenth dimension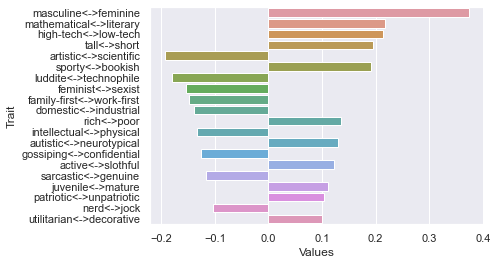
=============================== 30 rating threshold: newdf: (323, 236)
First dimension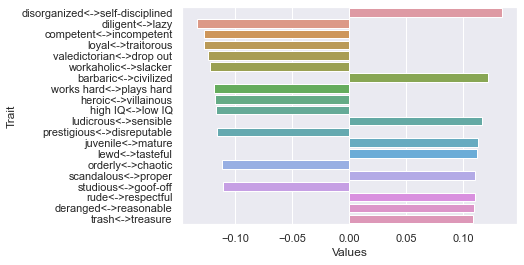
Second dimension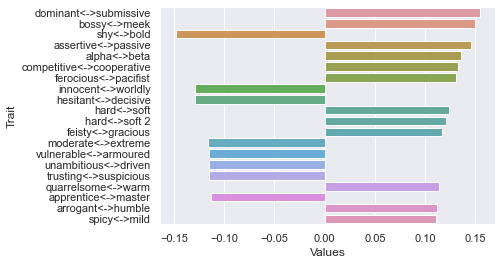
Third dimension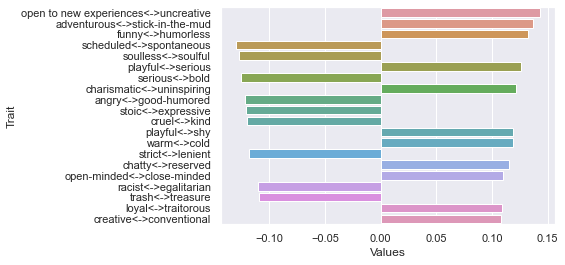
=============================== 40 rating threshold: newdf: (280, 236)
First dimension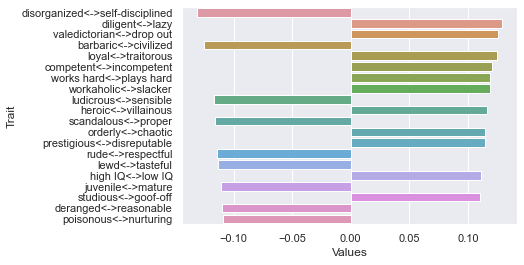
Second dimension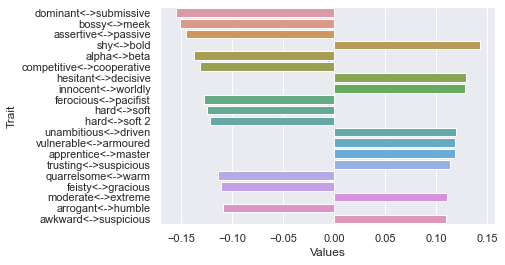
Third dimension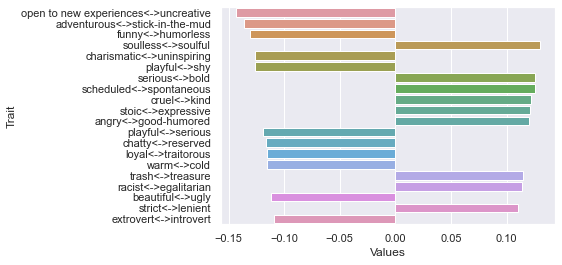
=============================== 50 rating threshold: newdf: (218, 236)
First dimension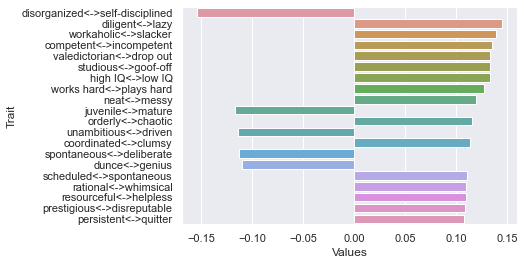
Second dimension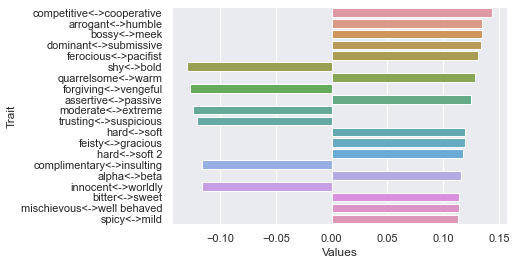
Third dimension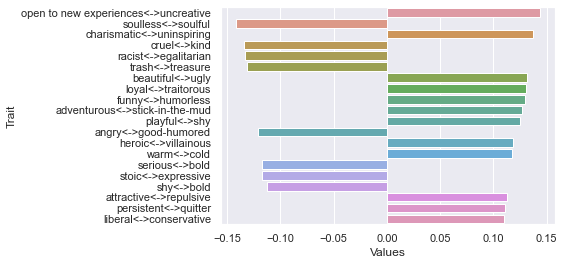
=============================== 60 rating threshold: newdf: (167, 236)
First dimension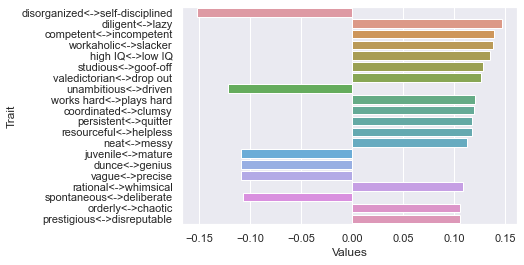
Second dimension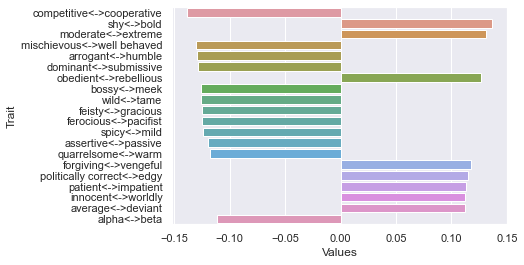
Third dimension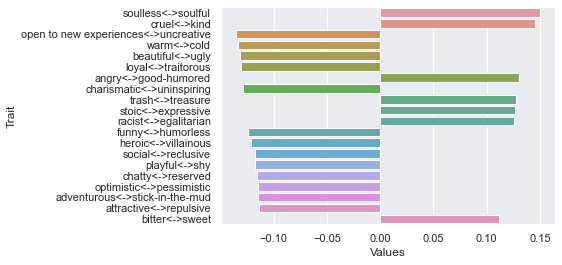
Fourth dimension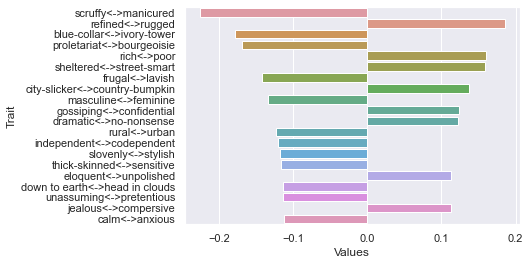
Fifth dimension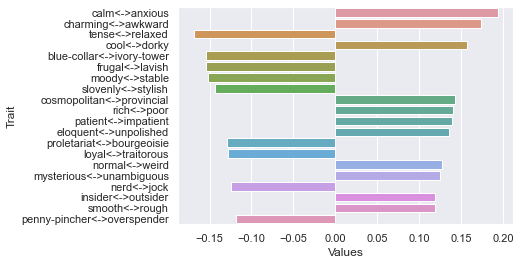
Sixth dimension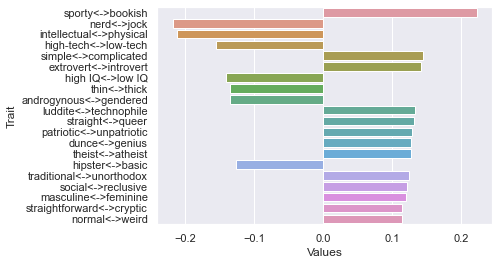
Seventh dimension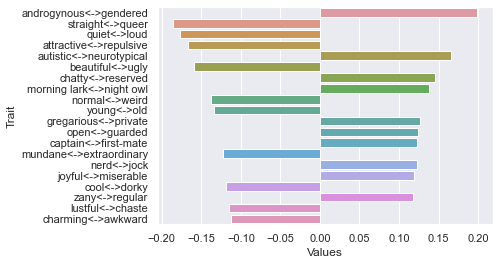
Eighth dimension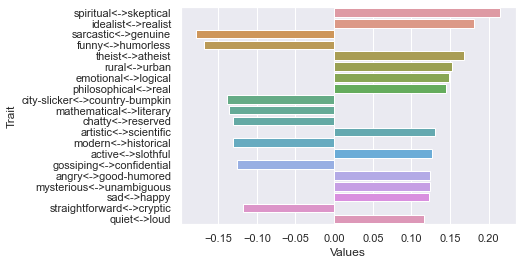
Ninth dimension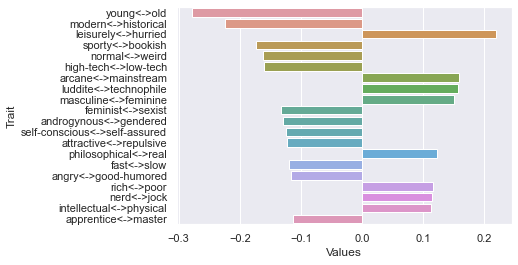
Tenth dimension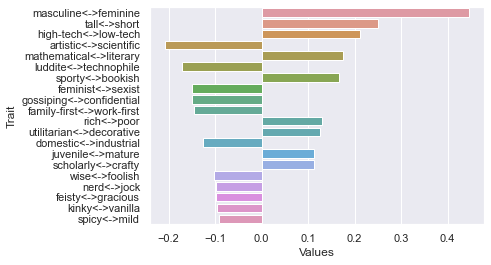
=============================== 70 rating threshold: newdf: (140, 236)
First dimension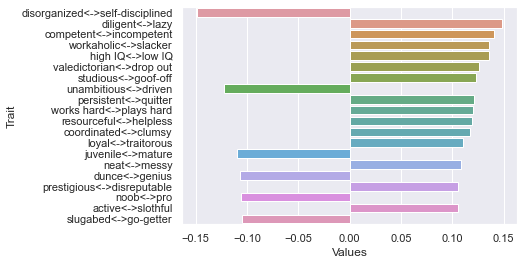
Second dimension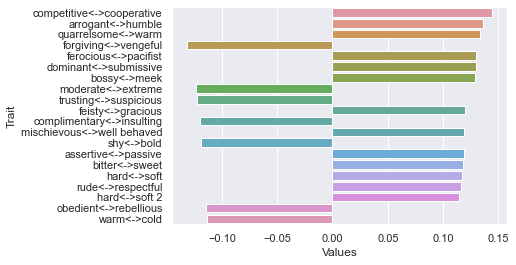
Third dimension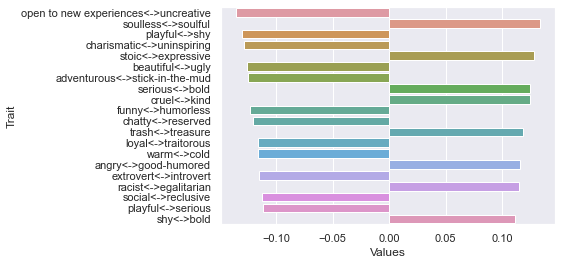
============================= 80 rating threshold: newdf: (132, 236) First dimension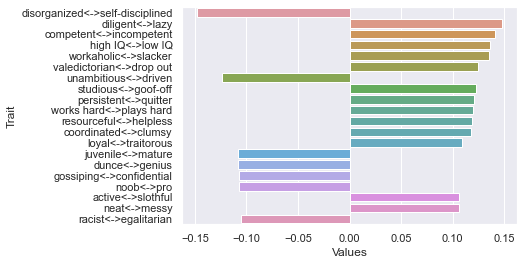
Second dimension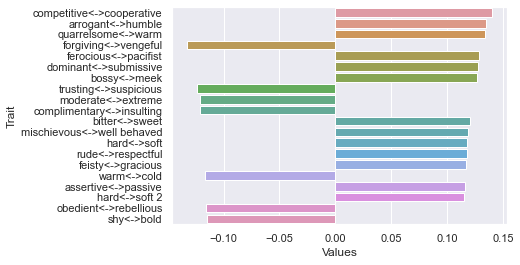
Third dimension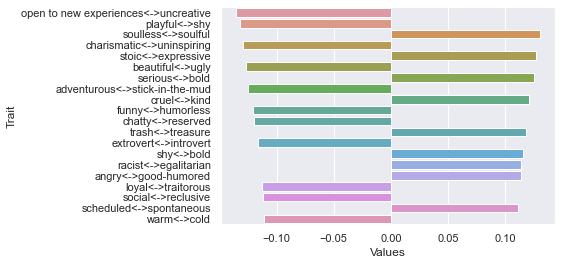
============================= 90 rating threshold: newdf: (116, 236) First dimension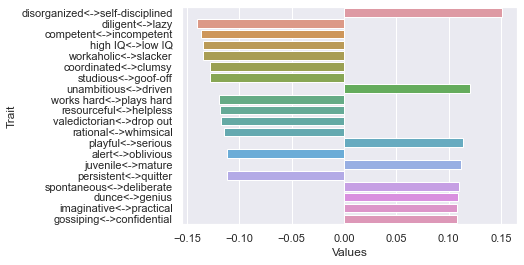
Second dimension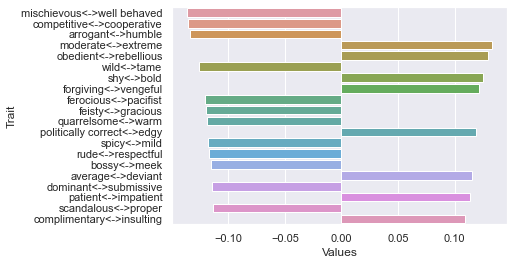
Third dimension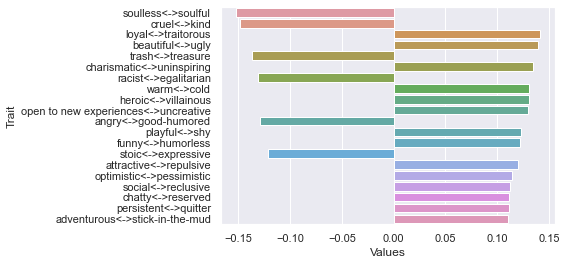
============================= 100 rating threshold: newdf: (100, 236)
First dimension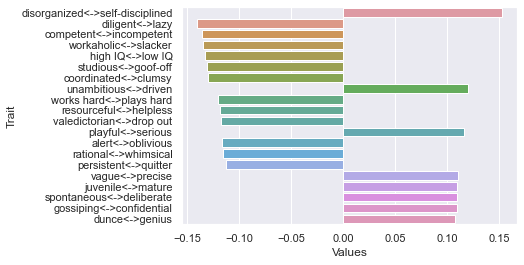
Second dimension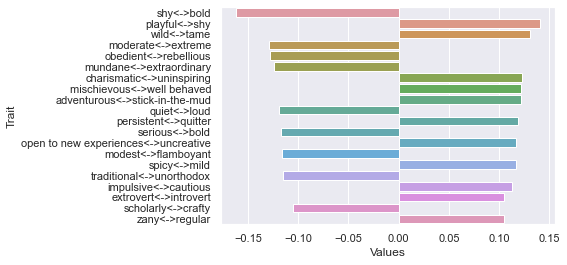
Third dimension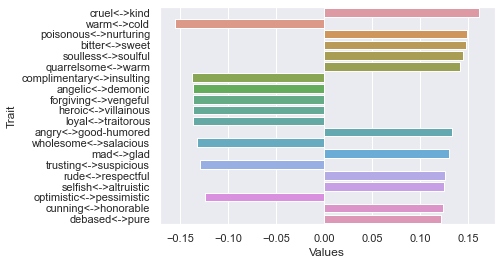
Fourth dimension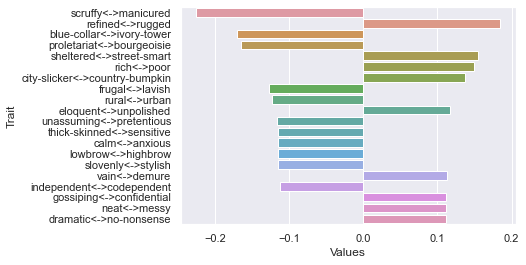
Fifth dimension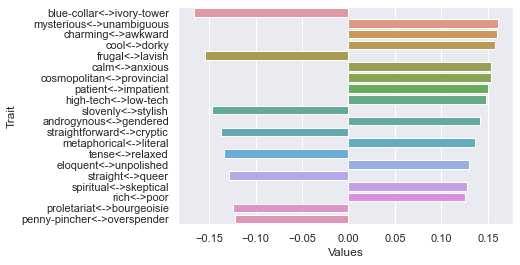
Sixth dimension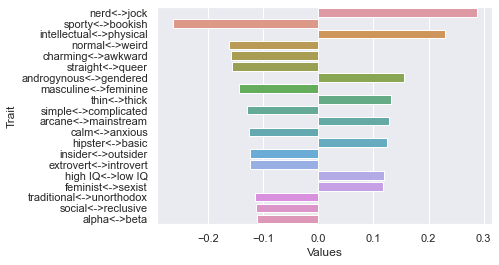
Seventh dimension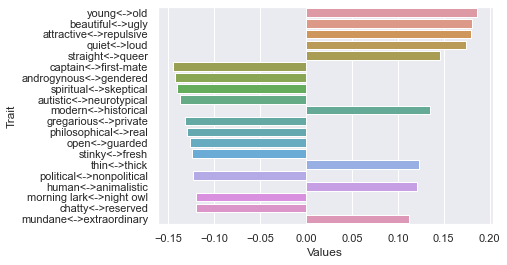
Eighth dimension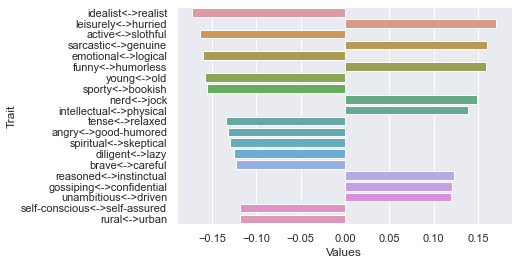
Ninth dimension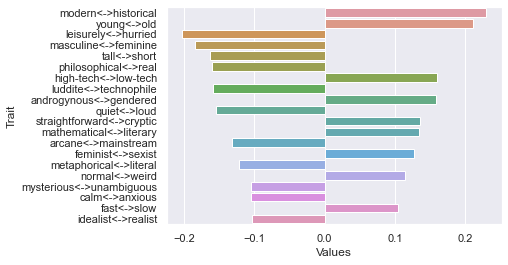
Tenth dimension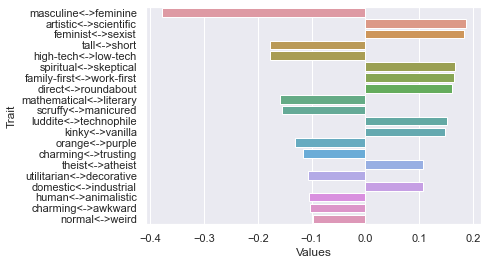
Eleventh dimension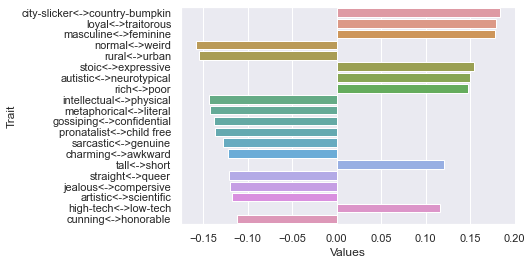
Twelfth dimension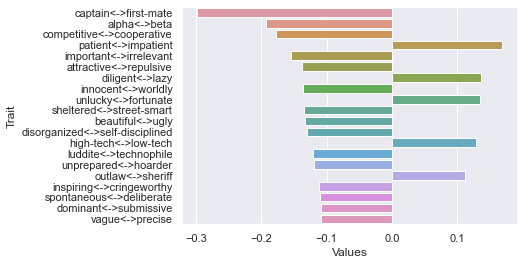
Thirteenth dimension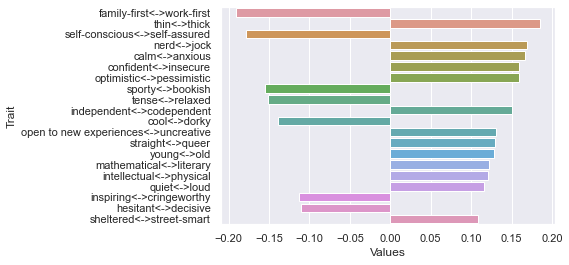
Fourteenth dimension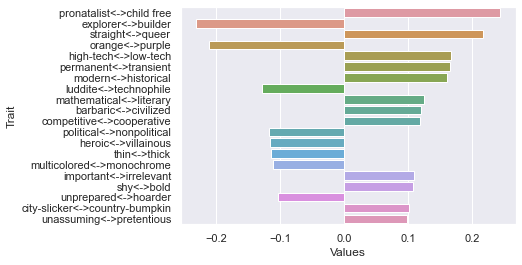
Fifteenth dimension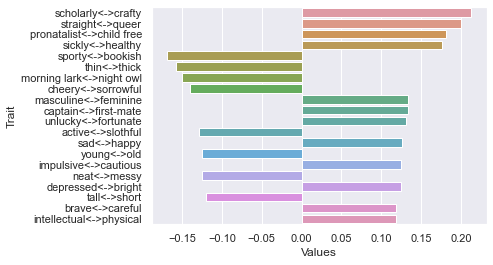
========================= Threshold 150 ratings: newdf: (40, 236)
First dimension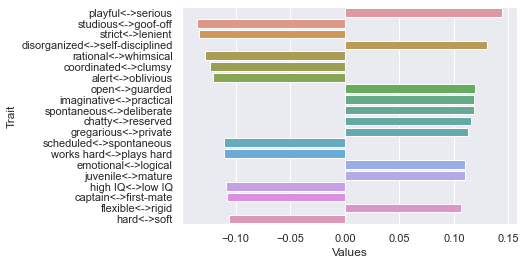
Second dimension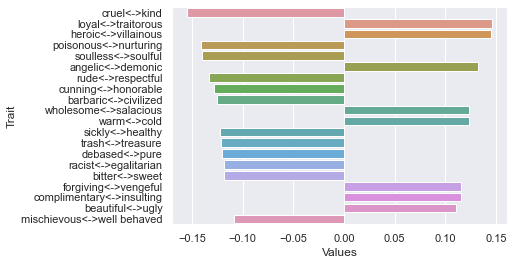
Third dimension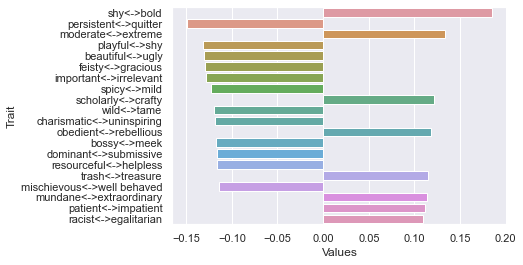
Fourth dimension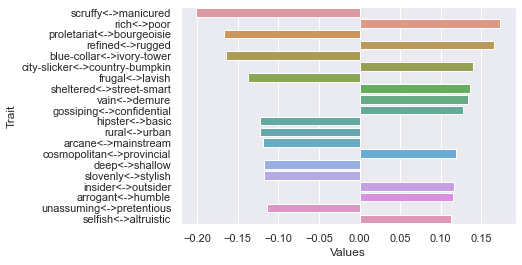
Fifth dimension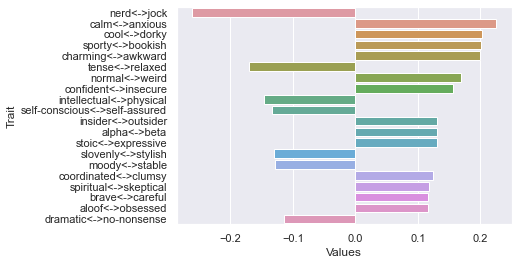
========================== Threshold 200 ratings: newdf: (19, 236)
First dimension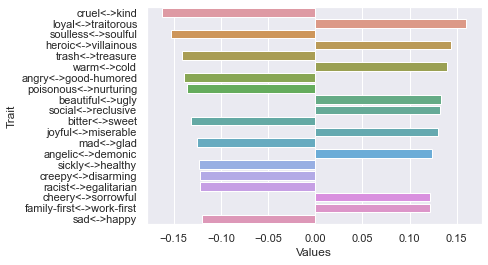
Second dimension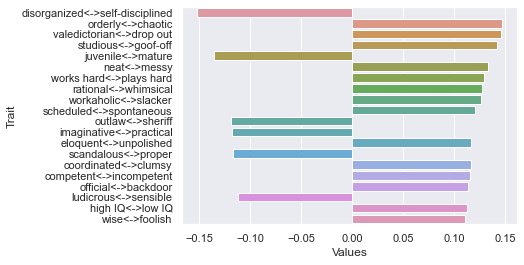
Third dimension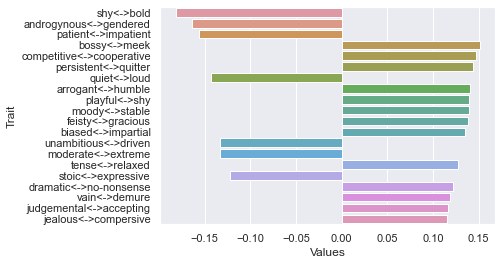
Fourth dimension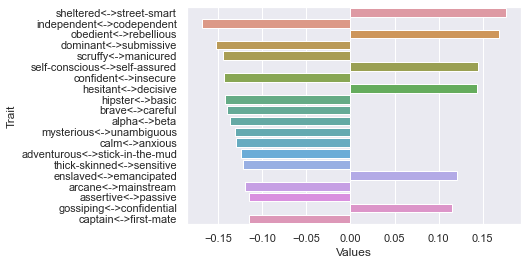
Fifth dimension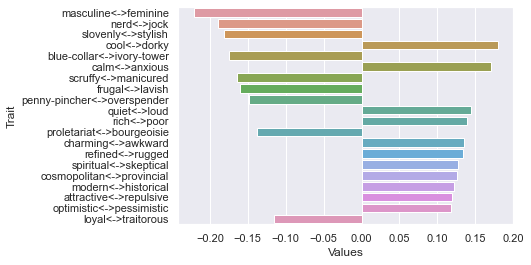
============================== Threshold 250 ratings: newdf: (12, 236)
First dimension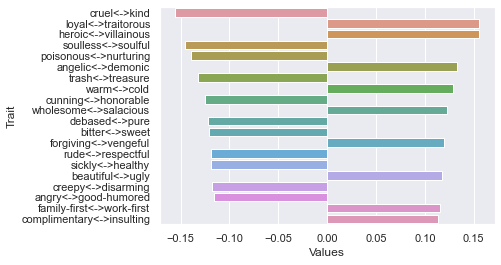
Second dimension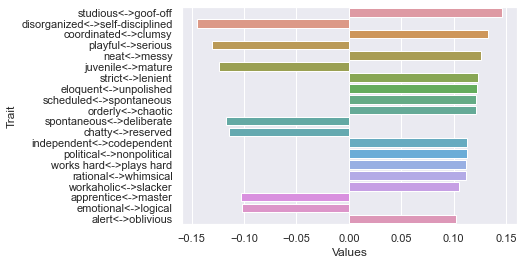
Third dimension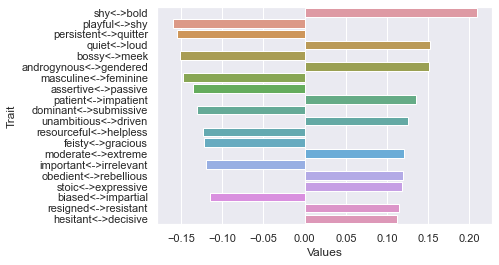
============================== Threshold 300 ratings: newdf: (5, 236)
First d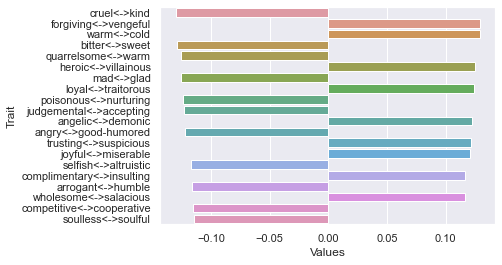
Second d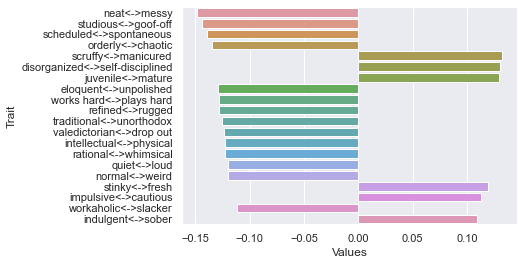
Third d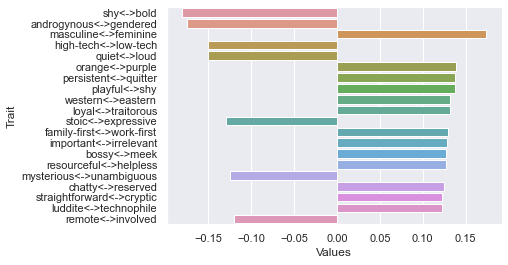
============================== To rerun (with e.g. 10 rating threshold):
Relevant code: