Closed xxoospring closed 4 years ago
Please describe more info.
Please describe more info.
- What kind of recipe (or dataset) did you use?
- Which model did you train? (Attach your config)
- How was the training curve?
I use LJSpeech1.1(trim leading and tail silence and do volume normalization, this is what I used for training Tacotron2)to train mb-melgan.v2, copy from egs/ljspeech/conf/multi_band_melgan.v2.yaml and set the fmax 8000
training cure is weird
training
eval
It seems loss value became NaN around 80k steps. I'm not sure which loss became NaN. I've never met loss = NaN so I'm wondering your data or config is something wrong. Please paste your whole config here.
It seems loss value became NaN around 80k steps. I'm not sure which loss became NaN. I've never met loss = NaN so I'm wondering your data or config is something wrong. Please paste your whole config here.
# This is the hyperparameter configuration file for MelGAN. # Please make sure this is adjusted for the LJSpeech dataset. If you want to # apply to the other dataset, you might need to carefully change some parameters. # This configuration requires ~ 8GB memory and will finish within 5 days on Titan V.
###########################################################
########################################################### sampling_rate: 22050 # Sampling rate. fft_size: 1024 # FFT size. hop_size: 256 # Hop size. win_length: null # Window length.
window: "hann" # Window function. num_mels: 80 # Number of mel basis. fmin: 80 # Minimum freq in mel basis calculation.
fmax: 8000 # Maximum frequency in mel basis calculation.
global_gain_scale: 1.0 # Will be multiplied to all of waveform. trim_silence: true # Whether to trim the start and end of silence. trim_threshold_in_db: 60 # Need to tune carefully if the recording is not good. trim_frame_size: 2048 # Frame size in trimming. trim_hop_size: 512 # Hop size in trimming. format: "hdf5" # Feature file format. "npy" or "hdf5" is supported.
###########################################################
########################################################### generator_type: "MelGANGenerator" # Generator type. generator_params: in_channels: 80 # Number of input channels. out_channels: 4 # Number of output channels. kernel_size: 7 # Kernel size of initial and final conv layers. channels: 384 # Initial number of channels for conv layers. upsample_scales: [8, 4, 2] # List of Upsampling scales. stack_kernel_size: 3 # Kernel size of dilated conv layers in residual stack. stacks: 4 # Number of stacks in a single residual stack module. use_weight_norm: True # Whether to use weight normalization. use_causal_conv: False # Whether to use causal convolution.
###########################################################
########################################################### discriminator_type: "MelGANMultiScaleDiscriminator" # Discriminator type. discriminator_params: in_channels: 1 # Number of input channels. out_channels: 1 # Number of output channels. scales: 3 # Number of multi-scales. downsample_pooling: "AvgPool1d" # Pooling type for the input downsampling. downsample_pooling_params: # Parameters of the above pooling function. kernel_size: 4 stride: 2 padding: 1 count_include_pad: False kernel_sizes: [5, 3] # List of kernel size. channels: 16 # Number of channels of the initial conv layer. max_downsample_channels: 512 # Maximum number of channels of downsampling layers. downsample_scales: [4, 4, 4] # List of downsampling scales. nonlinear_activation: "LeakyReLU" # Nonlinear activation function. nonlinear_activation_params: # Parameters of nonlinear activation function. negative_slope: 0.2 use_weight_norm: True # Whether to use weight norm.
###########################################################
########################################################### stft_loss_params: fft_sizes: [1024, 2048, 512] # List of FFT size for STFT-based loss. hop_sizes: [120, 240, 50] # List of hop size for STFT-based loss win_lengths: [600, 1200, 240] # List of window length for STFT-based loss. window: "hann_window" # Window function for STFT-based loss use_subband_stft_loss: true subband_stft_loss_params: fft_sizes: [384, 683, 171] # List of FFT size for STFT-based loss. hop_sizes: [30, 60, 10] # List of hop size for STFT-based loss win_lengths: [150, 300, 60] # List of window length for STFT-based loss. window: "hann_window" # Window function for STFT-based loss
###########################################################
########################################################### use_feat_match_loss: false # Whether to use feature matching loss. lambda_adv: 2.5 # Loss balancing coefficient for adversarial loss.
###########################################################
########################################################### batch_size: 64 # Batch size. batch_max_steps: 16384 # Length of each audio in batch. Make sure dividable by hop_size. pin_memory: true # Whether to pin memory in Pytorch DataLoader. num_workers: 4 # Number of workers in Pytorch DataLoader. remove_short_samples: true # Whether to remove samples the length of which are less than batch_max_steps. allow_cache: true # Whether to allow cache in dataset. If true, it requires cpu memory.
###########################################################
########################################################### generator_optimizer_type: "Adam" # Generator's optimizer type. generator_optimizer_params: lr: 1.0e-3 # Generator's learning rate. eps: 1.0e-7 # Generator's epsilon. weight_decay: 0.0 # Generator's weight decay coefficient. amsgrad: true generator_grad_norm: -1 # Generator's gradient norm. generator_scheduler_type: "MultiStepLR" # Generator's scheduler type. generator_scheduler_params: gamma: 0.5 # Generator's scheduler gamma. milestones: # At each milestone, lr will be multiplied by gamma.
###########################################################
########################################################### discriminator_train_start_steps: 200000 # Number of steps to start to train discriminator. train_max_steps: 1000000 # Number of training steps. save_interval_steps: 50000 # Interval steps to save checkpoint. eval_interval_steps: 1000 # Interval steps to evaluate the network. log_interval_steps: 1000 # Interval steps to record the training log.
###########################################################
########################################################### num_save_intermediate_results: 4 # Number of results to be saved as intermediate results.
Config seems fine.
Maybe your data has some perfect silence part? (Not sure)
Following part is doubtful. Why don't you try to use clamp
here?
https://github.com/kan-bayashi/ParallelWaveGAN/blob/88a829b5ef923657b4fb590e0614dec5e106a971/parallel_wavegan/losses/stft_loss.py#L52 https://github.com/kan-bayashi/ParallelWaveGAN/blob/88a829b5ef923657b4fb590e0614dec5e106a971/parallel_wavegan/losses/stft_loss.py#L73
Config seems fine. Maybe your data has some perfect silence part? (Not sure) Following part is doubtful. Why don't you try to use
clamp
here?
I didnt modify these code, I just modify run.sh and conf file
I mean, please modify these parts to avoid NaN errors by yourself and check how it works.
I checked the code but the following clamp should avoid NaN errors. https://github.com/kan-bayashi/ParallelWaveGAN/blob/88a829b5ef923657b4fb590e0614dec5e106a971/parallel_wavegan/losses/stft_loss.py#L31 I do not have an idea of why NaN has happened in your training.
when it came to 80K steps, all the prediction generated audios were blank audio: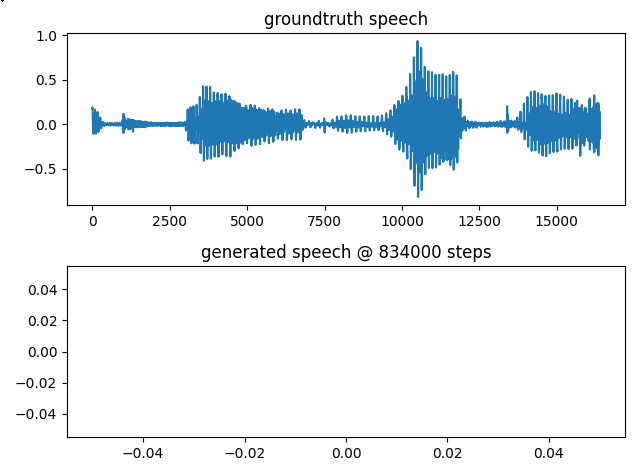