Open whitewindmills opened 7 months ago
If the weights are set to the same, I understand that's the effect.
I understand that sometimes the number of replicas is not divisible by the number of clusters. In this case, there must be some clusters with one more replica.
In this case, there must be some clusters with one more replica.
For general scenarios, we can only achieve the maximum approximate average assignment. This is an unchangeable fact.
How about describing it in detail at a community meeting?
cc @RainbowMango
Given the plausibility of this feature, and the fact that the difficulty of implementing it is not very complicated, how about we do this requirement as an OSPP project @RainbowMango @whitewindmills
if user specified it the strategy, will it ignore the result of score
step?
if user specified it the strategy, will it ignore the result of score step?
@Vacant2333 Great to hear your thoughts. I don't think this strategy has something to do with cluster scores. Cluster scores only are used to select clusters based on the cluster spread constraint.
hello, i wonder know that when will be different with when we use AverageReplicas
, at my understanding, static weight assignment will consider the cluster can create so many replicas, but AverageReplcias
will just assign the replicas, any other situation will cause different schdule result?
(( thanks for your answer @whitewindmills
apiVersion: policy.karmada.io/v1alpha1
kind: PropagationPolicy
metadata:
name: nginx-propagation
spec:
#...
placement:
replicaScheduling:
replicaDivisionPreference: Weighted
replicaSchedulingType: Divided
weightPreference:
staticWeightList:
- targetCluster:
clusterNames:
- member1
weight: 1
- targetCluster:
clusterNames:
- member2
weight: 1
@Vacant2333
Whether it's the static weight strategy or this AverageReplicas
strategy, they're just a way of assigning replicas. At present, the static weights strategy mainly have the following two ”disadvantages“:
Hope it helps you.
@whitewindmills i got it, if this feat is not add to OSPP, i would like to implement it~~ im watch on karmada-scheduler for now
Hi @Vacant2333 We are going to add this task to the OSPP 2024. You can join in the discussion and review.
/assign
@whitewindmills explained the reason why introducing a new replica allocation method at https://github.com/karmada-io/karmada/issues/4805#issuecomment-2069026112.
I'd like to hear your opinions on the following questions:
StaticWeight
doesn't take the spread constraints into account, but do you think it should?StaticWeight
should take available resource into account?@XiShanYongYe-Chang @chaunceyjiang @whitewindmills What's your thoughts?
I prefer to keep it as it is.
Why? Can you explain it in more detail?
@RainbowMango
there is no doubt that StaticWeight
is a static assignment strategy, which refers to a set of rules or configurations that are defined before a system or process runs and generally do not change unless manually updated. and the rules are set up in advance and do not adjust automatically based on real-time data or environmental changes.
so we get the expected output based on the input. AM I RIGHT?
if we try to change its default behavior, I think at least we can no longer call it StaticWeight
.
@XiShanYongYe-Chang @chaunceyjiang What do you think?
I think a new policy can be added to represent the average. The biggest difference between it and the StaticWeight policy is that the replicas is allocated considering the resources available. StaticWeight appears to be a rigid and inflexible way of allocating replicas, and is handled exactly as the user has set it up. Perhaps the user will only try this strategy in a test environment.
@RainbowMango what's your options. anyway, this PR https://github.com/karmada-io/karmada/pull/5225 is waiting for you to push forward.
My opinion on this feature is we can try to enhance the legacy feature staticWeight
. What we can do are:
static weight
considering spread constraint to select target clusters.static weight
taking available resources into account(if any cluster with insufficient resources, fails the schedule)I think it's a mistake that let static weight
skip spread constraint and available resources.
After that, the AverageReplicas
can be done by static weight.
Speaking of the use case mentioned on this issue:
As a developer, we have a deployment with 2 replicas that need to be deployed with high availability across AZs. We hope Karmada can schedule it for two AZs and ensure that there is a replica on each AZ.
I believe this is a reasonable use case, but more commonly, replicas are not evenly distributed across clusters, because some cluster servers as primary clusters while others act as backup clusters. In that case, the AverageReplicas
shows limited capacity compared to staticWeightList
.
My opinion on this feature is we can try to enhance the legacy feature
staticWeight
. What we can do are:
- Make
static weight
considering spread constraint to select target clusters.- Make
static weight
taking available resources into account(if any cluster with insufficient resources, fails the schedule)I think it's a mistake that let
static weight
skip spread constraint and available resources. After that, theAverageReplicas
can be done by static weight.Speaking of the use case mentioned on this issue:
As a developer, we have a deployment with 2 replicas that need to be deployed with high availability across AZs. We hope Karmada can schedule it for two AZs and ensure that there is a replica on each AZ.
I believe this is a reasonable use case, but more commonly, replicas are not evenly distributed across clusters, because some cluster servers as primary clusters while others act as backup clusters. In that case, the
AverageReplicas
shows limited capacity compared tostaticWeightList
.
I agree with you. If static weight
can take distribution constraints and resource sufficiency into consideration, then AverageReplicas
is really unnecessary. In addition, static weight
is more expressive than AverageReplicas
. However, I am a little worried about compatibility issues, because such changes will affect the performance of users' original static weight
strategies when they upgrade Karmada. @RainbowMango @whitewindmills
My opinion on this feature is we can try to enhance the legacy feature
staticWeight
. What we can do are:
- Make
static weight
considering spread constraint to select target clusters.- Make
static weight
taking available resources into account(if any cluster with insufficient resources, fails the schedule)I think it's a mistake that let
static weight
skip spread constraint and available resources. After that, theAverageReplicas
can be done by static weight.Speaking of the use case mentioned on this issue:
As a developer, we have a deployment with 2 replicas that need to be deployed with high availability across AZs. We hope Karmada can schedule it for two AZs and ensure that there is a replica on each AZ.
I believe this is a reasonable use case, but more commonly, replicas are not evenly distributed across clusters, because some cluster servers as primary clusters while others act as backup clusters. In that case, the
AverageReplicas
shows limited capacity compared tostaticWeightList
.
I have just reviewed the code related to skipping spread constraints and available resources in the current static weight
strategy of Karmada. I believe that the suggestions you proposed may lead to other issues and involve significant refactoring costs. If we want the current static weight to support distribution constraints and available resources, we need to consider the following points:
The first issue is compatibility, which is unavoidable. Previously, users set strategies based on the premise that static weight
does not consider distribution constraints and available resources. The planned changes would obviously impact the expected execution results of these strategies.
If we indeed make changes, the following areas are likely to be affected:
Select
phase: The main changes would involve the shouldIgnoreSpreadConstraint
function (removing the condition that allows the static weight
strategy to skip constraints). This will have two impacts. First, clusters will be grouped not only by cluster dimension but also by Region, Zone, and Provider dimensions. This impact is relatively minor. However, the second impact involves cluster selection, which will shift from selecting all clusters in the Select
phase to selecting only clusters that meet the distribution constraints. This may result in no available clusters and errors such as the number of clusters is less than the cluster spreadConstraint.MinGroups
. Additionally, the static weight
strategy specifies the weight
of a certain type of cluster through the ClusterAffinity
type. If we modify the Select
logic of static weight
(for example, if we need to select Cluster by Region
), it is very likely that the clusters specified by the user in the yaml file will be discarded due to distribution constraints and other reasons. This does not align with the user's original intent or the design of the static weight
API.Assign
phase: The main changes would involve the assignByStaticWeightStrategy
function. Currently, this function does not consider the available capacity of each candidate cluster but directly allocates instances to candidate clusters based on weight. If we need to consider available capacity, we must ensure that the cluster type specified by the user can accommodate the number of instances corresponding to the weight ratio. Otherwise, we need to make a judgment. One option is to directly reject the allocation, resulting in scheduling failure. Another option is to distribute the excess instances to other clusters to ensure successful scheduling. I believe most users prefer successful scheduling rather than having the entire scheduling fail due to insufficient resources in any cluster, as this would increase the failure rate of the static weight
strategy. However, if we choose the latter, the final allocation result of the static weight
strategy may not match the set weight distribution, leading to discrepancies between the actual outcome and user expectations, which may not be desirable.In conclusion, I believe that enhancing static weight
to implement AverageReplica
is not appropriate. It would not only incur refactoring costs and compatibility issues but also contradict the original design intent of the static weight
API and increase the failure rate of this strategy, leading to a poor user experience. @RainbowMango @whitewindmills
Hi, As discussed with @whitewindmills @XiShanYongYe-Chang and @ipsum-0320 on a temporary meeting, we need to revisit the original design of static weight
. Just share what I found here:
The StaticWeight
feature was introduced by #1161 at the year 2021, and it was migrated from ReplicaSchedulingPolicy.
The implementation can be found at v0.9.0, both cluster available resources and spread constraint not take into account at that time.
In my opinion, currently the use case of StaticWeight
is still not clear, and I think it's a great chance for us to enhance it. The use case described on this issue is exactly the use case of StaticWeight
.
/reopen
@whitewindmills: Reopened this issue.
What would you like to be added:
Background
We want to introduce a new replica assignment strategy in the scheduler, which supports an even assignment of the target replicas across the currently selected clusters.
Explanation
After going through the filtering, prioritization, and selection phases, three clusters(
member1
,member2
,member3
) were selected. We will automatically assign 9 replicas equally among these three clusters, the result we expect is[{member1: 3}, {member2: 3}, {member3: 3}]
.Why is this needed:
User Story
As a developer, we have a deployment with 2 replicas that needs to be deployed with high availability across AZs. We hope Karmada can schedule it to two AZs and ensure that there is a replica on each AZ.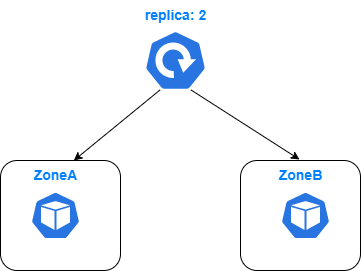
Our PropagationPolicy might look like this:
But unfortunately, the strategy
AvailableReplicas
does not guarantee that our replicas are evenly assigned.Any ideas?
We can introduce a new replica assignment strategy like
AvailableReplicas
, maybe we can name itAverageReplicas
. It is essentially different from static weight assignment, because it does not support spread constraints and is mandatory. When assigning replicas, it does not consider whether the cluster can place so many replicas.