Open Oldpan opened 6 years ago
For the purpose that more people can read it, I will still reply using English but you can contact me by email if you still have troubles in reproducing it in PyTorch...😄
Firstly, the VGG-19 requires input image to be [0, 255] and then...
Secondly, did you subtract the mean pixel?
One easier way to debug might be starting from a PyTorch version of style transfer implementation such as this one from original author Leon Gatys: https://github.com/leongatys/PytorchNeuralStyleTransfer
Wow, so fast.. The image I input is [0-1]. I use the code in pytorch:
def toTensor(img):
assert type(img) == np.ndarray,'the img type is {}, but ndarry expected'.format(type(img))
img = cv2.cvtColor(img, cv2.COLOR_BGR2RGB)
img = torch.from_numpy(img.transpose((2, 0, 1))).unsqueeze(0).clone()
return img.float().div(255.0)
And I also do the normalization to the image.
cnn_normalization_mean = torch.tensor([0.485, 0.456, 0.406]).to(device)
cnn_normalization_std = torch.tensor([0.229, 0.224, 0.225]).to(device)
# create a module to normalize input image so we can easily put it in a
# nn.Sequential
class Normalization(nn.Module):
def __init__(self, mean, std):
super(Normalization, self).__init__()
# .view the mean and std to make them [C x 1 x 1] so that they can
# directly work with image Tensor of shape [B x C x H x W].
# B is batch size. C is number of channels. H is height and W is width.
self.mean = torch.tensor(mean).view(-1, 1, 1)
self.std = torch.tensor(std).view(-1, 1, 1)
def forward(self, img):
# normalize img
return (img - self.mean) / self.std
But the problem still exists. So I'm trying to figure out ... Yeah,if I can't fix thie problem maybe I should start from Pytorch version of other's. Uh.uhuh..I think I need to contact you with email.
I think VGG-19 requires input to be [0, 255] subtracted by mean pixel?
thanks!
I had sent you a gmail the day before yesterday and I don't know if you have received it.
The model I use is from Pytorch and the VGG-19 model requires input to be [0-1]...I think the model I use is right because it works in common style transfer.
I compared two ways to load the image: PIL vs OpenCV-python, and I found something weird but I'm still not sure where the problem comes from.
I'm confused about the code below from 'neural_gram.lua':
if name == style_layers[next_style_idx] then
print("Setting up style layer ", i, ":", layer.name)
local gram = GramMatrix():float():cuda()
local input = net:forward(content_image_caffe):clone()
local target = net:forward(style_image_caffe):clone()
local mask = mask_image:clone():repeatTensor(1,1,1):expandAs(target):cuda()
# if I don't use histogram match and just input target to gram-loss function
# like this : target_gram = gram:forward(target):clone()
local match, correspondence =
cuda_utils.patchmatch_r(input, target, params.patchmatch_size, 1)
match:cmul(mask)
local target_gram = gram:forward(match):clone()
target_gram:div(mask:sum())
local norm = params.normalize_gradients
local loss_module = nn.StyleLoss(params.style_weight, target_gram, norm, mask_image):float():cuda()
net:add(loss_module)
table.insert(style_losses, loss_module)
next_style_idx = next_style_idx + 1
end
I haven't use histogram and just input activation layer from style-image to gram-loss function. If I do this, the image I produced is totally wrong? Or just not looks so well but still right? Thank you for helping me!
If only style loss but no histogram loss the quality will be less good but not like the one you posted. There might still have some bug so I would first ensure entire image style transfer works and then debug masked region using unit tests.
Yeah...The entire image style transfer works. So I'm still debugging in mask mode and when I change the style layers and content layers position the result changes drastically.
I check my code over again and expel all the bugs I could find
There are some of my results...
When I change the style weight and content weight the result also changes.Some times the image seemed to get better.
But I still can't find the proper weight to produce a nice image...
[sad][sad][sad]
After a long parameters tuning the first step is almost working.
But when I run patchmatch like patchmatch_r()
function I often run out of memory.
Does this function needs memory more than 10GB?
Thanks~
It's a nice work and I want to use pytorch to re-accomplish it. But during the first step 'IndependentMapping' I meet a pixel mess problem...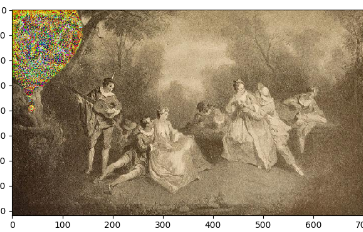
I do clamp and use mask image to do backward but the pixels seem to be out of range. The loss function I use are content loss,gram loss, and TV loss. I haven't use histogram loss. The model I use is Vgg19 from pytorch model zoo which the data range is 0-1.I'm sure the image I put into is right format(RGB) and when I tune the style weight or content the result changes a bit. I have no clear idea where the problem is. Can you help me?Thanks! 貌似我该说中文?