Closed zyoohv closed 5 years ago
The samples you’re showing seem to be from super early in training (3000 iterations). Do you have samples from later on?
On Fri, Sep 21, 2018 at 8:23 AM zyoohv notifications@github.com wrote:
I run your code in cifar10, but the result seems not as good as our expected.
- system information:
system: debian 8 python: python2 pytorch: torch==0.3.1
- run command:
$python main.py --dataset cifar10 --dataroot ~/.torch/datasets --cuda
- output part:
[24/25][735/782][3335] Loss_D: -1.287177 Loss_G: 0.642245 Loss_D_real: -0.651701 Loss_D_fake 0.635477 [24/25][740/782][3336] Loss_D: -1.269792 Loss_G: 0.621307 Loss_D_real: -0.657210 Loss_D_fake 0.612582 [24/25][745/782][3337] Loss_D: -1.250543 Loss_G: 0.636843 Loss_D_real: -0.667046 Loss_D_fake 0.583497 [24/25][750/782][3338] Loss_D: -1.196252 Loss_G: 0.589907 Loss_D_real: -0.606480 Loss_D_fake 0.589772 [24/25][755/782][3339] Loss_D: -1.189609 Loss_G: 0.564263 Loss_D_real: -0.612895 Loss_D_fake 0.576714 [24/25][760/782][3340] Loss_D: -1.178156 Loss_G: 0.586755 Loss_D_real: -0.600268 Loss_D_fake 0.577888 [24/25][765/782][3341] Loss_D: -1.087157 Loss_G: 0.508717 Loss_D_real: -0.522565 Loss_D_fake 0.564592 [24/25][770/782][3342] Loss_D: -1.092081 Loss_G: 0.674212 Loss_D_real: -0.657483 Loss_D_fake 0.434598 [24/25][775/782][3343] Loss_D: -0.937950 Loss_G: 0.209016 Loss_D_real: -0.310877 Loss_D_fake 0.627073 [24/25][780/782][3344] Loss_D: -1.316574 Loss_G: 0.653665 Loss_D_real: -0.693675 Loss_D_fake 0.622899 [24/25][782/782][3345] Loss_D: -1.222763 Loss_G: 0.558372 Loss_D_real: -0.567426 Loss_D_fake 0.655337
fake_samples_500.png [image: fake_samples_500] https://user-images.githubusercontent.com/16134679/45865905-9a46ea80-bdb1-11e8-99c5-7ee2c8432cf6.png
fake_samples_1000.png [image: fake_samples_1000] https://user-images.githubusercontent.com/16134679/45865910-9c10ae00-bdb1-11e8-8158-acc3f2e42146.png
fake_samples_1500.png [image: fake_samples_1500] https://user-images.githubusercontent.com/16134679/45865913-9dda7180-bdb1-11e8-9a41-0cd490c124f7.png
fake_samples_2000.png [image: fake_samples_2000] https://user-images.githubusercontent.com/16134679/45865917-9f0b9e80-bdb1-11e8-8f02-a4ddb21c2f85.png
fake_samples_2500.png [image: fake_samples_2500] https://user-images.githubusercontent.com/16134679/45865921-a0d56200-bdb1-11e8-9e2c-101644e49cda.png
fake_samples_3000.png [image: fake_samples_3000] https://user-images.githubusercontent.com/16134679/45865924-a29f2580-bdb1-11e8-9a03-f61b328b2d87.png
Note that this is real_samples.png!!! [image: real_samples] https://user-images.githubusercontent.com/16134679/45865928-a468e900-bdb1-11e8-8c26-5eca7b41bdfa.png
— You are receiving this because you are subscribed to this thread. Reply to this email directly, view it on GitHub https://github.com/martinarjovsky/WassersteinGAN/issues/62, or mute the thread https://github.com/notifications/unsubscribe-auth/AFB0kjsndk_WrLq8waluc-aO9ktV8o1Rks5udJQEgaJpZM4Wzl0Y .
The running of the code has finished !
@martinarjovsky
fake_samples_25000.png
loss log
[167/1000][555/782][24987] Loss_D: -0.772816 Loss_G: -0.022373 Loss_D_real: -0.193230 Loss_D_fake 0.579586
[167/1000][560/782][24988] Loss_D: -0.800285 Loss_G: 0.586990 Loss_D_real: -0.633111 Loss_D_fake 0.167174
[167/1000][565/782][24989] Loss_D: -0.585662 Loss_G: 0.091040 Loss_D_real: -0.018860 Loss_D_fake 0.566802
[167/1000][570/782][24990] Loss_D: -0.930666 Loss_G: 0.580418 Loss_D_real: -0.650177 Loss_D_fake 0.280490
[167/1000][575/782][24991] Loss_D: -0.745919 Loss_G: 0.111690 Loss_D_real: -0.156507 Loss_D_fake 0.589412
[167/1000][580/782][24992] Loss_D: -0.981289 Loss_G: 0.589674 Loss_D_real: -0.631602 Loss_D_fake 0.349687
[167/1000][585/782][24993] Loss_D: -0.933379 Loss_G: 0.301309 Loss_D_real: -0.388805 Loss_D_fake 0.544573
[167/1000][590/782][24994] Loss_D: -1.077024 Loss_G: 0.548679 Loss_D_real: -0.589278 Loss_D_fake 0.487745
[167/1000][595/782][24995] Loss_D: -0.914252 Loss_G: 0.511773 Loss_D_real: -0.556782 Loss_D_fake 0.357470
[167/1000][600/782][24996] Loss_D: -1.090694 Loss_G: 0.532181 Loss_D_real: -0.572747 Loss_D_fake 0.517948
[167/1000][605/782][24997] Loss_D: -0.898265 Loss_G: 0.501241 Loss_D_real: -0.532952 Loss_D_fake 0.365313
[167/1000][610/782][24998] Loss_D: -0.943638 Loss_G: 0.485056 Loss_D_real: -0.502485 Loss_D_fake 0.441153
[167/1000][615/782][24999] Loss_D: -0.991872 Loss_G: 0.501545 Loss_D_real: -0.539097 Loss_D_fake 0.452775
[167/1000][620/782][25000] Loss_D: -1.001911 Loss_G: 0.499365 Loss_D_real: -0.527643 Loss_D_fake 0.474269
I train it 25000 iters, but the result seems still not right. Could you help me find out what's wrong with it?
I have change the model into 256 image size(Input image size 64 to 256 size).
then run this code 41600 iteration (800 epochs and 270 iterations at Batchsize 32).
I have use 9000 face image data set.
but over result is not generated good.
/home/mnit/PycharmProjects/ICB2019/WGAN_Pytorch_Clf256/samples/fake_samples_41600.png
Random Seed: 6408
G True
G True
DCGAN_G_nobn(
(main): Sequential(
(initial.100-512.convt): ConvTranspose2d(100, 512, kernel_size=(4, 4), stride=(1, 1), bias=False)
(initial.512.relu): ReLU(inplace)
(pyramid.512-256.convt): ConvTranspose2d(512, 256, kernel_size=(4, 4), stride=(2, 2), padding=(1, 1), bias=False)
(pyramid.256.relu): ReLU(inplace)
(pyramid.256-128.convt): ConvTranspose2d(256, 128, kernel_size=(4, 4), stride=(2, 2), padding=(1, 1), bias=False)
(pyramid.128.relu): ReLU(inplace)
(pyramid.128-64.convt): ConvTranspose2d(128, 64, kernel_size=(4, 4), stride=(2, 2), padding=(1, 1), bias=False)
(pyramid.64.relu): ReLU(inplace)
(pyramid.64-32.convt): ConvTranspose2d(64, 32, kernel_size=(4, 4), stride=(2, 2), padding=(1, 1), bias=False)
(pyramid.32.relu): ReLU(inplace)
(pyramid.32-16.convt): ConvTranspose2d(32, 16, kernel_size=(4, 4), stride=(2, 2), padding=(1, 1), bias=False)
(pyramid.16.relu): ReLU(inplace)
(final.16-3.convt): ConvTranspose2d(16, 3, kernel_size=(4, 4), stride=(2, 2), padding=(1, 1), bias=False)
(final.3.tanh): Tanh()
)
)
D True
('initial WGAN Dis: ndf csize ndf', 16, 128, 16)
('Input Feature', 16, 'output feature', 32)
('WGAN Dis: size csize ndf', 256, 64, 32)
('Input Feature', 32, 'output feature', 64)
('WGAN Dis: size csize ndf', 256, 32, 64)
('Input Feature', 64, 'output feature', 128)
('WGAN Dis: size csize ndf', 256, 16, 128)
('Input Feature', 128, 'output feature', 256)
('WGAN Dis: size csize ndf', 256, 8, 256)
('Input Feature', 256, 'output feature', 512)
('WGAN Dis: size csize ndf', 256, 4, 512)
DCGAN_D(
(main): Sequential(
(initial.conv.3-16): Conv2d(3, 16, kernel_size=(4, 4), stride=(2, 2), padding=(1, 1), bias=False)
(initial.relu.16): LeakyReLU(0.2, inplace)
(pyramid.16-32.conv): Conv2d(16, 32, kernel_size=(4, 4), stride=(2, 2), padding=(1, 1), bias=False)
(pyramid.32.batchnorm): BatchNorm2d(32, eps=1e-05, momentum=0.1, affine=True)
(pyramid.32.relu): LeakyReLU(0.2, inplace)
(pyramid.32-64.conv): Conv2d(32, 64, kernel_size=(4, 4), stride=(2, 2), padding=(1, 1), bias=False)
(pyramid.64.batchnorm): BatchNorm2d(64, eps=1e-05, momentum=0.1, affine=True)
(pyramid.64.relu): LeakyReLU(0.2, inplace)
(pyramid.64-128.conv): Conv2d(64, 128, kernel_size=(4, 4), stride=(2, 2), padding=(1, 1), bias=False)
(pyramid.128.batchnorm): BatchNorm2d(128, eps=1e-05, momentum=0.1, affine=True)
(pyramid.128.relu): LeakyReLU(0.2, inplace)
(pyramid.128-256.conv): Conv2d(128, 256, kernel_size=(4, 4), stride=(2, 2), padding=(1, 1), bias=False)
(pyramid.256.batchnorm): BatchNorm2d(256, eps=1e-05, momentum=0.1, affine=True)
(pyramid.256.relu): LeakyReLU(0.2, inplace)
(pyramid.256-512.conv): Conv2d(256, 512, kernel_size=(4, 4), stride=(2, 2), padding=(1, 1), bias=False)
(pyramid.512.batchnorm): BatchNorm2d(512, eps=1e-05, momentum=0.1, affine=True)
(pyramid.512.relu): LeakyReLU(0.2, inplace)
(final.512-1.conv): Conv2d(512, 1, kernel_size=(4, 4), stride=(1, 1), bias=False)
)
)
Loss_D: -1.515402 Loss_G: 0.700609 Loss_D_real: -0.823006 Loss_D_fake 0.692396 Loss_D: -1.515402 Loss_G: 0.700609 Loss_D_real: -0.823006 Loss_D_fake 0.692396 Loss_D: -1.515402 Loss_G: 0.700609 Loss_D_real: -0.823006 Loss_D_fake 0.692396 loss fuction is not change at 41600 iteration
@praveenkumarchandaliya
I think you can try small images such as 3232 or 6464. The method work well in all dataset with small image size in my experiment.
good luck.
@zyoohv Have you got good results for CIFAR10 data with default parameter settings? How many epochs have you run? Thanks!
I haven't run the code in cifar 10. You may want to take a look at https://github.com/igul222/improved_wgan_training where we provide a very good cifar10 model.
Cheers :) Martin
I run your code in cifar10, but the result seems not as good as our expected.
system: debian 8
python: python2 pytorch: torch==0.3.1
fake_samples_500.png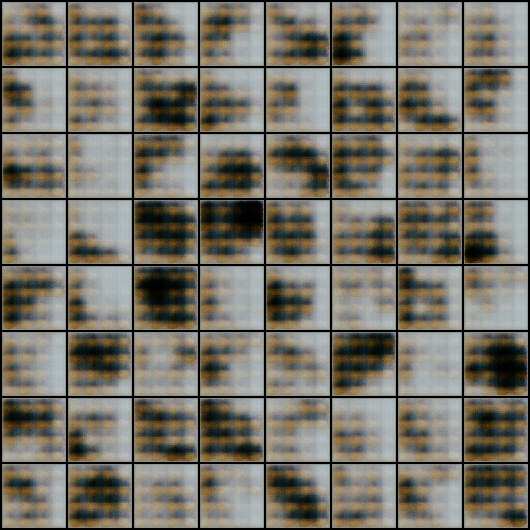
fake_samples_1000.png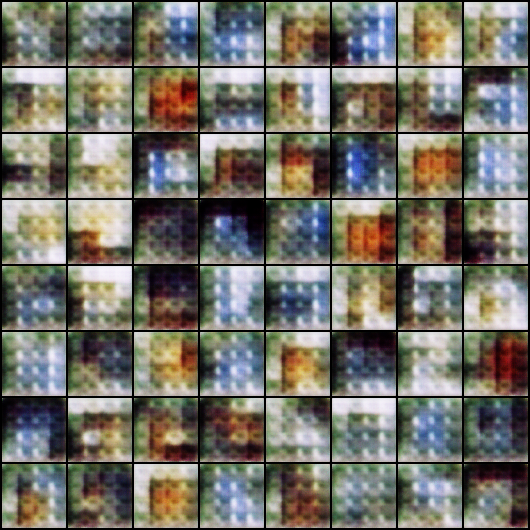
fake_samples_1500.png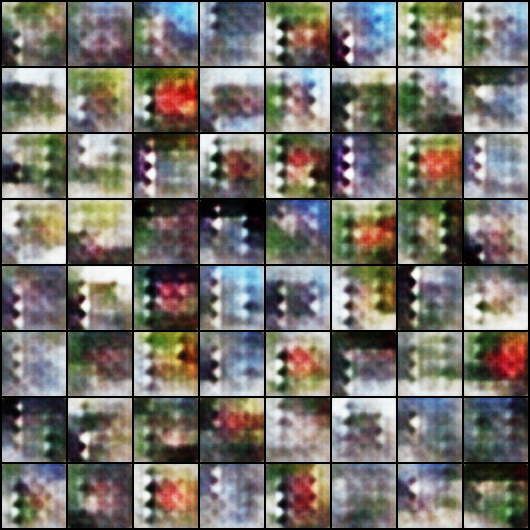
fake_samples_2000.png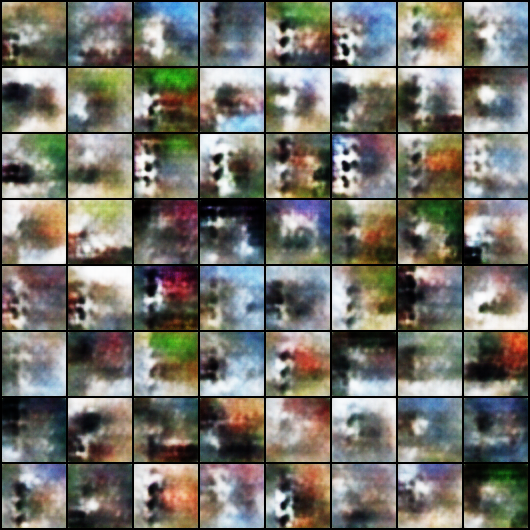
fake_samples_2500.png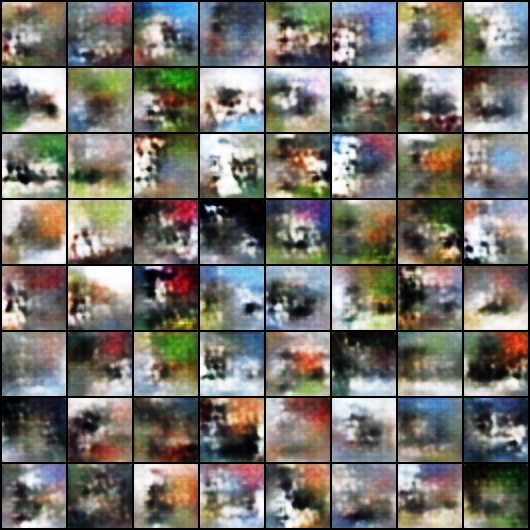
fake_samples_3000.png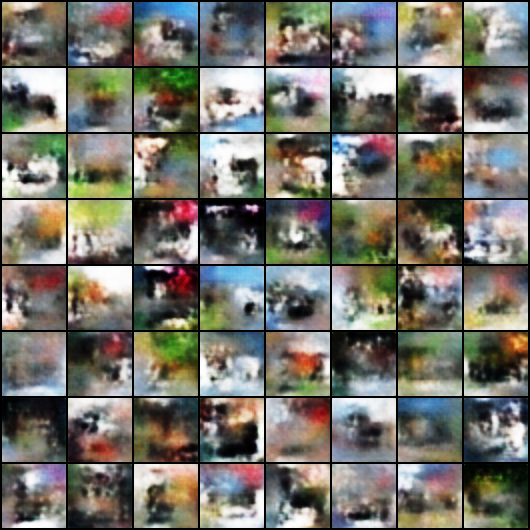
Note that this is real_samples.png!!!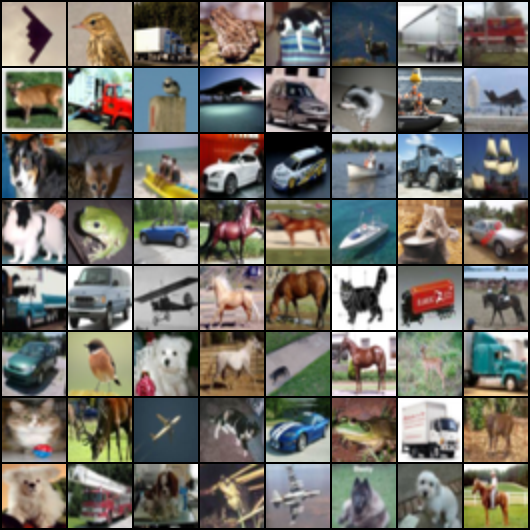