Closed OswaldoBornemann closed 4 years ago
try without forward attention but location sensitive attention. 2nd one looks fine btw. Reopen if you need it.
@erogol But how could you successfully used forward attention
with LJSpeech
dataset ?
I don'rt remember how I trained it before but currently I sue it for inference only.
@erogol thanks a lot ! And happy new year ~
I am trying Tacotron2 based on LJSpeech dataset and try to reproduced the result that
erogol
did. But both two experiments did not work well, both of two seem to have high stop loss.The first experiment main config is below
The results are below
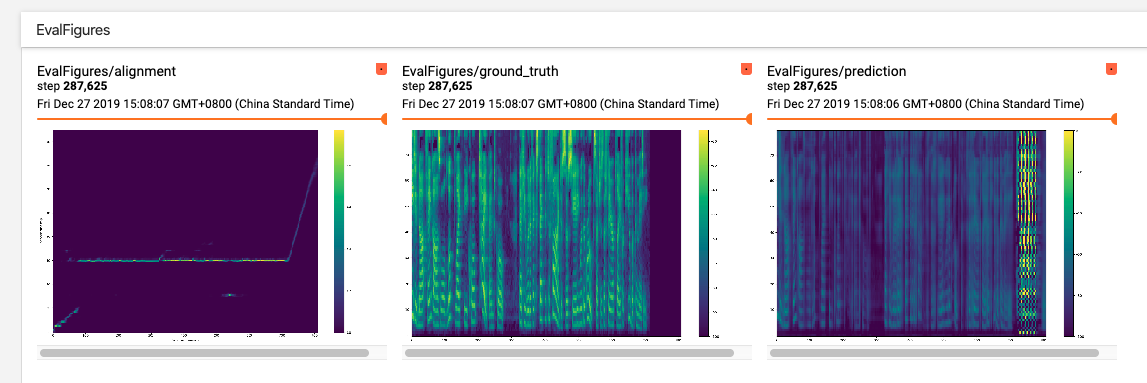
The second experiment main config is below
Both two experiments have been trained almost 10 days.