Closed mratsim closed 4 years ago
Some more readings:
A Unified Scheduler for Recursive and TaskDataflow Parallelism
http://www.cs.qub.ac.uk/~H.Vandierendonck/papers/PACT11_unified.pdf
Nabbit, Executing Dynamic Task Graph Using Work-Stealing
https://pdfs.semanticscholar.org/7c1d/5701d6f8f1a311b99e96f25c501ae70e7b58.pdf
Combining Dataflow programming with Polyhedral Optimization
Also it seems like Cholesky decomposition would be a good candidate to test dataflow dependencies
The generated code of the following nested loop:
proc main4() =
init(Weave)
expandMacros:
parallelForStrided i in 0 ..< 100, stride = 30:
parallelForStrided j in 0 ..< 200, stride = 60:
captures: {i}
log("Matrix[%d, %d] (thread %d)\n", i, j, myID())
exit(Weave)
echo "\n\nStrided Nested loops"
echo "-------------------------"
main4()
echo "-------------------------"
is:
proc parallelForSection() {.nimcall, gcsafe.} =
let this`gensym453091 = localCtx.worker.currentTask
var i = this`gensym453091.start
inc this`gensym453091.cur, 1
while i < this`gensym453091.stop:
type
CapturedTy = (typeof(i))
discard
proc parallelForSection(i: typeof(i)) {.nimcall, gcsafe.} =
let this`gensym453805 = localCtx.worker.currentTask
var j = this`gensym453805.start
inc this`gensym453805.cur, 1
while j < this`gensym453805.stop:
discard "captures:\n {i}"
c_printf("Matrix[%d, %d] (thread %d)\n", i, j, localCtx.worker.ID)
flushFile(stdout)
j += this`gensym453805.stride
this`gensym453805.cur += this`gensym453805.stride
loadBalance(Weave)
proc weaveParallelFor(param`gensym453408: pointer) {.nimcall, gcsafe.} =
let this`gensym453409 = localCtx.worker.currentTask
const
expr`gensym454268 = "not isRootTask(this`gensym453409)"
let data = cast[ptr CapturedTy](param`gensym453408)
parallelForSection(data[])
let task`gensym453410 = newTaskFromCache()
task`gensym453410.parent = localCtx.worker.currentTask
task`gensym453410.fn = weaveParallelFor
task`gensym453410.isLoop = true
task`gensym453410.start = 0
task`gensym453410.cur = 0
task`gensym453410.stop = 200
task`gensym453410.stride = 60
cast[ptr CapturedTy](addr(task`gensym453410.data))[] = i
schedule(task`gensym453410)
i += this`gensym453091.stride
this`gensym453091.cur += this`gensym453091.stride
loadBalance(Weave)
proc weaveParallelFor(param`gensym453086: pointer) {.nimcall, gcsafe.} =
let this`gensym453087 = localCtx.worker.currentTask
const
expr`gensym454834 = "not isRootTask(this`gensym453087)"
parallelForSection()
let task`gensym453088 = newTaskFromCache()
task`gensym453088.parent = localCtx.worker.currentTask
task`gensym453088.fn = weaveParallelFor
task`gensym453088.isLoop = true
task`gensym453088.start = 0
task`gensym453088.cur = 0
task`gensym453088.stop = 100
task`gensym453088.stride = 30
schedule(task`gensym453088)
It should be possible to create a waitable dummy future for each iteration of the outer loop and sync on it in the inner loop.
Some more reflexion on nestable barriers (#41).
It doesn't seem possible in the current design or compatible with work-stealing in general.
What can be done is something similar to #omp taskwait
or Cilk sync
which is to await for all child task to be finished.
However a sync barrier for all threads in a nested context cannot be done because there is no guarantee that all threads will actually get the task as they are lazily splitted, so that will lead to a deadlock.
A taskgraph approach is possible, however lots of care must be given to reduce the overhead. Note that the main motivation behind nestable barriers, being able to implement state-of-the-art GEMM is partially solved by #66.
However in #66 the following constructs prevent weave-based GEMM from being nestable to build an im2col + GEMM based convolution parallelized at both the batch level and the GEMM level. https://github.com/mratsim/weave/blob/789fa95f9153c353abc7430c0dc95724e839e885/benchmarks/matmul_gemm_blas/gemm_pure_nim/gemm_weave.nim#L152-L182
More support for abandoning full nestable barriers: http://www.open-std.org/jtc1/sc22/wg21/docs/papers/2012/n3409.pdf and use the Cilk "fully strict" semantics "only await the children spawns" so that the barrier is composable.
That does not remove the need for task dependency graphs (implemented as message passing!)
X10 introduces the "terminally-strict where a task can await any of its descendants and not just its direct children. Weave can actually do that. One interesting thing is that X10 decouples function scope with sync/awaitable scope. See paper: https://www.semanticscholar.org/paper/Work-First-and-Help-First-Scheduling-Policies-for-Barik-Guo/2cb7ac2c3487aae8302cd205446710ea30f9c016
Closely-related to our need the following paper presents Data-Driven Futures to express complex dependencies. This is equivalent to "andThen" continuations in async/await https://www.cs.rice.edu/~vs3/PDF/DDT-ICPP2011.pdf. The model seems compatible with channel-based work-stealing. From the paper their Cholesky decomposition was better performing than the publication time state-of-the-art which is interesting as Cholesky is array-based.
Slides of Data-Driven Futures: http://cnc11.hpcgarage.org/slides/Tasirlar.pdf
Cholesky with Data-Driven Futures
This can be benched against either LAPACK Cholesky or a lower-level OpenMP task dependency based Cholesky from http://www.netlib.org/utk/people/JackDongarra/PAPERS/task-based-cholesky.pdf
Here is LU Decomposition and Cholesky Decomposition in pseudo code and dependency graph from http://www.cs.ucy.ac.cy/dfmworkshop/wp-content/uploads/2014/07/Exploring-HPC-Parallelism-with-Data-Driven-Multithreating.pdf
Data-driven futures seem suitable to express pipeline and graph parallelism
Besides the distributed computing scenario another thing to keep in my mind is if that helps or hinders heterogeneous (distributed?) CPU + GPU computing
However this is not sufficient to allow nesting of matrix multiplications in parallel regions (for example in a batched matrix multiplication or convolution or LU/QR/Cholesky factorization)
So we really need to be able to express data-dependences (while currently we express control-flow dependencies via spawn/sync).
Looking into other runtimes (TBB Flowgraph, XKaapi/libkomp, Cpp-Taskflow, Nabbit, ...) most use a concurrent hash table which doesn't map well with message passing, distributed computing or GPU computing.
StarPU (nice high level overview, histoy and challenges here On Runtime Systems for Task-based Programming on Heterogeneous Platforms might provide a way forward.
More Cholesky representations from the StarPU paper:
We could add the support of delayed tasks and promises, i.e. each worker has a separate queue of delayed tasks, they are added to the normal work-stealing scheduler only when a promised is fulfilled.
Promises can be implemented as a channel + a delivered
/fulfilled
boolean (as a channel read consumes a message). They probably need to be threadsafe ref-counted object as a thread may allocate a promise, declare a deferred task and return and threads working on the promise and then triggering the declared task is not the same.
For example
type Promise = ptr object
fulfiller: Channel[Dummy]
refCount: Atomic[int32]
isDelivered: Atomic[bool]
A task dependent on a promise would check regularly if it was delivered and if not query the fulfiller channel. If query is successful, isDelivered is changed to true (so that multiple tasks can dependent on the same promise) and the task is scheduled.
Supporting for-loop might requires creating a matrix of 1000x1000 promises if we want to express dependency at the cell-level for a 1000x1000 matrix. Obviously this is a worst-case and:
Nonetheless, there might be more efficient data-structure like interval trees or segment trees. The channel could be replaced by a MPSC channel a consumer would check the interval data structure to see if its dependency is fulfilled, if not it takes a lock, drain the channel and update the interval data structure with all received chunks. This unfortunately would add another synchronization data structure beyond channels and event notifiers.
Distributed runtimes are the most relevant here as they have to use message-passing across a cluster.
Kokkos seems to use an array of dependencies:
https://www.osti.gov/servlets/purl/1562647
StarPU and HPX to be checked.
If we add support for delayed tasks, a thread might create a lot of delayed tasks all sitting in its delayed task queue. The proposed solution only add them to the normal runtime once their dependencies are fulfilled meaning, a thread might have all tasks. This is similar to the load imbalance the single-task-producer benchmark is measuring. It should be solved by adaptative steal half.
In the proposed scheme, fulfilling a promise is done by user code so the runtime has no knowledge of tasks that are actually blocking further work. AST analysis could be done to locate such task and place them in a "priority" deque (it wouldn't support priority, they would just be ran or stolen from first)
Another extension to Cilk to add dataflow graph aware scheduling: Swan
In terms of benchmark, besides Cholesky a lot of publications are referencing the Smith-Waterman algorithm for DNA and protein sequence alignment and a computational biology benchmark would be a nice change of pace.
See: https://uh-ir.tdl.org/bitstream/handle/10657/558/Thesis12.pdf?sequence=1&isAllowed=y
and https://www.cs.colostate.edu/~pouchet/doc/lcpc-article.15.pdf (polyhedral compiler generated)
Dataflow graph parallelism / Stream parallelism / Pipeline parallelism / Data-driven task parallelism (AFAIK it's all the same) is able to express computations dependent on data readiness while plain task parallelism and data parallelism are only aware of control flow.
This is needed for:
Parallel video encoding/decoding when a lot of computations are dependent on a video frame being decompressed/computed: https://hal.inria.fr/hal-01289532/document (Adding detaflow to FFMpeg)
This does not take into account intra-frame parallelism, resulting speedup compared to FFMpeg are in the order of 50%~80%
All the Polybench iterative stencil benchmarks for benchmarking polyhedral compilers are relevant for benchmarking polyhedral dataflow. Writing a video encoder for benchmarking is probably a bit difficult but who knows
From commits:
I am almost there to port a state-of-the-art BLAS to Weave and compete with OpenBLAS and MKL, however parallelizing 2 nested loops with read-after-writes dependencies causes issues.
Analysis
The current barrier is Master thread only and is only suitable for the root task
https://github.com/mratsim/weave/blob/7802daf62652754c4e0815e139842fe820600870/weave/runtime.nim#L88-L96
Unfortunately in nested parallel loops normal workers could also reach it and will not be stopped and they will create more tasks or continue on their current one even though dependencies are not resolved.
Potential solutions
Extending the barrier
Extend the current barrier to work with worker threads in nested situations. An implementation of typical workload with nested barriers should be added to the bench suite to test behaviour in with a known testable workload.
Providing static loop scheduling
Providing static loop scheduling, by eagerly splitting the work may (?) prevent one thread running away creating tasks when their dependencies were not resolved. Need more thinking as I have trouble considering all the scenarios.
Create a waitable nested-for loops iterations
Weave already provides very-fine grained synchronization primitives with futures, we do not need to wait for threads but just for the iteration that does the packing work we depend on to. A potential syntax would be:
This would create a dummy future called
icForLoop
that could waited on by calls that are further nested.Unknowns:
Task graphs
While a couple of other frameworks are expressing such as task graphs:
The syntax of make_edge/precede is verbose, it doesn't seem to address the issues of fine-grained loop.
I believe it's better to express emerging dependencies via data dependencies, i.e. futures and waitable ranges
OpenMP tasks dependencies approach could be suitable and made cleaner, from OpenMP 4.5 doc: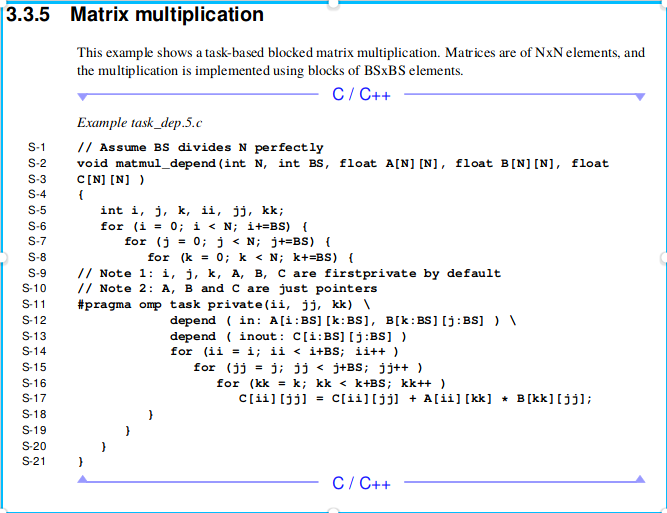
Or CppSs (, https://gitlab.com/szs/CppSs, https://www.thinkmind.org/download.php?articleid=infocomp_2013_2_30_10112, https://arxiv.org/abs/1502.07608)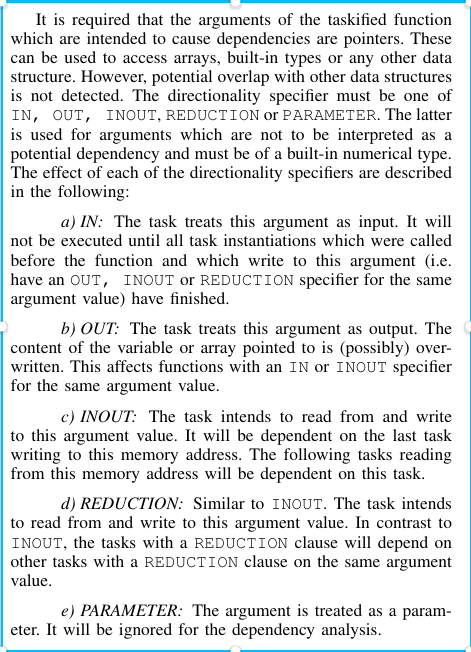
Or Kaapi (https://tel.archives-ouvertes.fr/tel-01151787v1/document)
Create a polyhedral compiler
Well, the whole point of Weave is easing creating a linear algebra compiler so ...
Code explanation
The way the code is structured is the following (from BLIS paper [2]):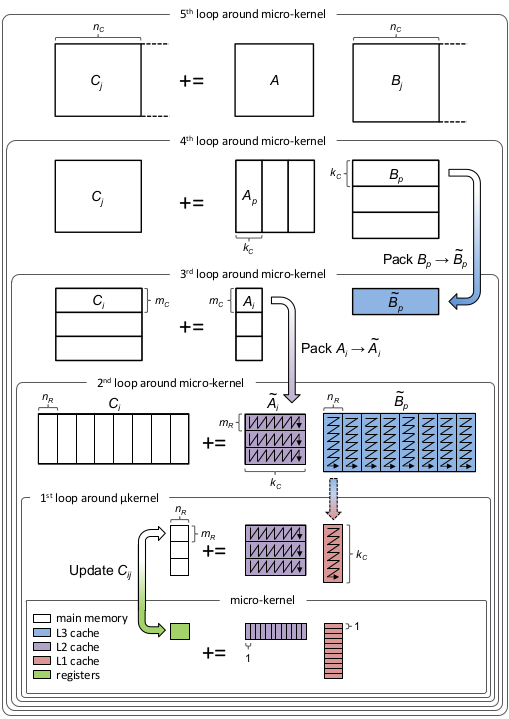
For MxN = MxK KN Assuming a shared memory arch with priavate L1-L2 cache per core and shared L3, Tiling for CPU caches and register blocking is done the following way:
mc*kc/(2mc+2kc) with mc*kc < K
Loop: Difficult to parallelize
as K is the reduction dimension
so parallelizing K
requires handling conflicting writes
Normally parallelized but missing a way to express dependency or a worker barrier
References
[1] Anatomy of High-Performance Matrix Multiplication (Revised) Kazushige Goto, Robert A. Van de Geijn
http://www.cs.utexas.edu/~flame/pubs/GotoTOMS_revision.pdf
[2] Anatomy of High-Performance Many-Threaded Matrix Multiplication Smith et al
http://www.cs.utexas.edu/users/flame/pubs/blis3_ipdps14.pdf
[3] Automating the Last-Mile for High Performance Dense Linear Algebra Veras et al
https://arxiv.org/pdf/1611.08035.pdf
[4] GEMM: From Pure C to SSE Optimized Micro Kernels Michael Lehn
http://apfel.mathematik.uni-ulm.de/~lehn/sghpc/gemm/index.html
Laser wiki - GEMM optimization resources