Open notasadsong opened 2 years ago
Hi, I recommend cloning the Colab notebook again and re-running it. I just re-ran it in Colab and my result looks very different from yours. Did you make changes to the notebook?
I just cloned the github code again and ran it with the command in Colab notebook. I'm sure I haven't change anything but I got really different results.
The grayscale image is as follow, without any segmentation:
Maybe there is someting wrong with it?
Hi @notasadsong, could you please post screenshots and copy the text output of all of the cells here, from the Colab session you are running? (from a fresh copy of the Colab)?
Hi @johnwlambert, I didn't run the code in the Colab. Instead I ran it in the command window. I got the text output as follow:
~/桌面/mseg-se/mseg-semantic$ python3 -u mseg_semantic/tool/universal_demo.py --config=mseg_semantic/config/test/default_config_360_ms.yaml model_name mseg-3m model_path mseg-3m.pth input_file dirtroad10.jpg Namespace(config='mseg_semantic/config/test/default_config_360_ms.yaml', file_save='default', opts=['model_name', 'mseg-3m', 'model_path', 'mseg-3m.pth', 'input_file', 'dirtroad10.jpg']) arch: hrnet base_size: 360 batch_size_val: 1 dataset: dirtroad10 has_prediction: False ignore_label: 255 img_name_unique: False index_start: 0 index_step: 0 input_file: dirtroad10.jpg layers: 50 model_name: mseg-3m model_path: mseg-3m.pth network_name: None save_folder: default scales: [0.5, 0.75, 1.0, 1.25, 1.5, 1.75] small: True split: val test_gpu: [0] test_h: 713 test_w: 713 version: 4.0 vis_freq: 20 workers: 16 zoom_factor: 8 [2022-02-26 19:16:13,716 INFO universal_demo.py line 50 1110] arch: hrnet base_size: 360 batch_size_val: 1 dataset: dirtroad10 has_prediction: False ignore_label: 255 img_name_unique: True index_start: 0 index_step: 0 input_file: dirtroad10.jpg layers: 50 model_name: mseg-3m model_path: mseg-3m.pth network_name: None print_freq: 10 save_folder: default scales: [0.5, 0.75, 1.0, 1.25, 1.5, 1.75] small: True split: test test_gpu: [0] test_h: 713 test_w: 713 u_classes: ['backpack', 'umbrella', 'bag', 'tie', 'suitcase', 'case', 'bird', 'cat', 'dog', 'horse', 'sheep', 'cow', 'elephant', 'bear', 'zebra', 'giraffe', 'animal_other', 'microwave', 'radiator', 'oven', 'toaster', 'storage_tank', 'conveyor_belt', 'sink', 'refrigerator', 'washer_dryer', 'fan', 'dishwasher', 'toilet', 'bathtub', 'shower', 'tunnel', 'bridge', 'pier_wharf', 'tent', 'building', 'ceiling', 'laptop', 'keyboard', 'mouse', 'remote', 'cell phone', 'television', 'floor', 'stage', 'banana', 'apple', 'sandwich', 'orange', 'broccoli', 'carrot', 'hot_dog', 'pizza', 'donut', 'cake', 'fruit_other', 'food_other', 'chair_other', 'armchair', 'swivel_chair', 'stool', 'seat', 'couch', 'trash_can', 'potted_plant', 'nightstand', 'bed', 'table', 'pool_table', 'barrel', 'desk', 'ottoman', 'wardrobe', 'crib', 'basket', 'chest_of_drawers', 'bookshelf', 'counter_other', 'bathroom_counter', 'kitchen_island', 'door', 'light_other', 'lamp', 'sconce', 'chandelier', 'mirror', 'whiteboard', 'shelf', 'stairs', 'escalator', 'cabinet', 'fireplace', 'stove', 'arcade_machine', 'gravel', 'platform', 'playingfield', 'railroad', 'road', 'snow', 'sidewalk_pavement', 'runway', 'terrain', 'book', 'box', 'clock', 'vase', 'scissors', 'plaything_other', 'teddy_bear', 'hair_dryer', 'toothbrush', 'painting', 'poster', 'bulletin_board', 'bottle', 'cup', 'wine_glass', 'knife', 'fork', 'spoon', 'bowl', 'tray', 'range_hood', 'plate', 'person', 'rider_other', 'bicyclist', 'motorcyclist', 'paper', 'streetlight', 'road_barrier', 'mailbox', 'cctv_camera', 'junction_box', 'traffic_sign', 'traffic_light', 'fire_hydrant', 'parking_meter', 'bench', 'bike_rack', 'billboard', 'sky', 'pole', 'fence', 'railing_banister', 'guard_rail', 'mountain_hill', 'rock', 'frisbee', 'skis', 'snowboard', 'sports_ball', 'kite', 'baseball_bat', 'baseball_glove', 'skateboard', 'surfboard', 'tennis_racket', 'net', 'base', 'sculpture', 'column', 'fountain', 'awning', 'apparel', 'banner', 'flag', 'blanket', 'curtain_other', 'shower_curtain', 'pillow', 'towel', 'rug_floormat', 'vegetation', 'bicycle', 'car', 'autorickshaw', 'motorcycle', 'airplane', 'bus', 'train', 'truck', 'trailer', 'boat_ship', 'slow_wheeled_object', 'river_lake', 'sea', 'water_other', 'swimming_pool', 'waterfall', 'wall', 'window', 'window_blind'] version: 4.0 vis_freq: 20 workers: 16 zoom_factor: 8 [2022-02-26 19:16:13,716 INFO universal_demo.py line 51 1110] => creating model ... [2022-02-26 19:16:15,910 INFO inference_task.py line 284 1110] => loading checkpoint 'mseg-3m.pth' [2022-02-26 19:16:42,545 INFO inference_task.py line 290 1110] => loaded checkpoint 'mseg-3m.pth' [2022-02-26 19:16:42,549 INFO inference_task.py line 302 1110] >>>>>>>>>>>>>> Start inference task >>>>>>>>>>>>> [2022-02-26 19:16:42,551 INFO inference_task.py line 337 1110] Write image prediction to dirtroad10_overlaid_classes.jpg /home/zwh/anaconda3/lib/python3.9/site-packages/torch/nn/functional.py:3631: UserWarning: Default upsampling behavior when mode=bilinear is changed to align_corners=False since 0.4.0. Please specify align_corners=True if the old behavior is desired. See the documentation of nn.Upsample for details. warnings.warn( /home/zwh/anaconda3/lib/python3.9/site-packages/torch/nn/functional.py:3509: UserWarning: nn.functional.upsample is deprecated. Use nn.functional.interpolate instead. warnings.warn("nn.functional.upsample is deprecated. Use nn.functional.interpolate instead.") [2022-02-26 19:21:20,020 INFO inference_task.py line 330 1110] <<<<<<<<<<< Inference task completed <<<<<<<<<<<<<<
I see. If you run it in Colab, what is the result you see? Can you quickly open up this Colab link, run all the cells (will take no more than 5 min), and share your Colab result here?
If you can copy the lines verbatim from the Colab to a bash script on your local machine, the result should be identical : - ) If not, can you please share your OS, python versions, versions of every library from here: https://github.com/mseg-dataset/mseg-semantic/blob/master/requirements.txt#L1, the exact commands you are running, and the bash script you are using to execute this?
I ran it in Colab and I got the expected result which looks so great. Still I didn't see identical result when I ran it on local machine. I use ubuntu18.04, python3.9.7, and the version of the libraries(only contains the libraries with version infomation) are as follow:
hydra-core==1.1.1 opencv-python==4.5.5.62 pandas==1.3.4 Pillow==8.4.0 PyYAML==6.0 sklearn==0.0 torch==1.10.2
Hi! I am trying to use the pretrained models to process images from KITTI Odometry and changed nothing of the code. But I got some invalid segmentations. Then I tested in the sample image4 in here .The output is as follow: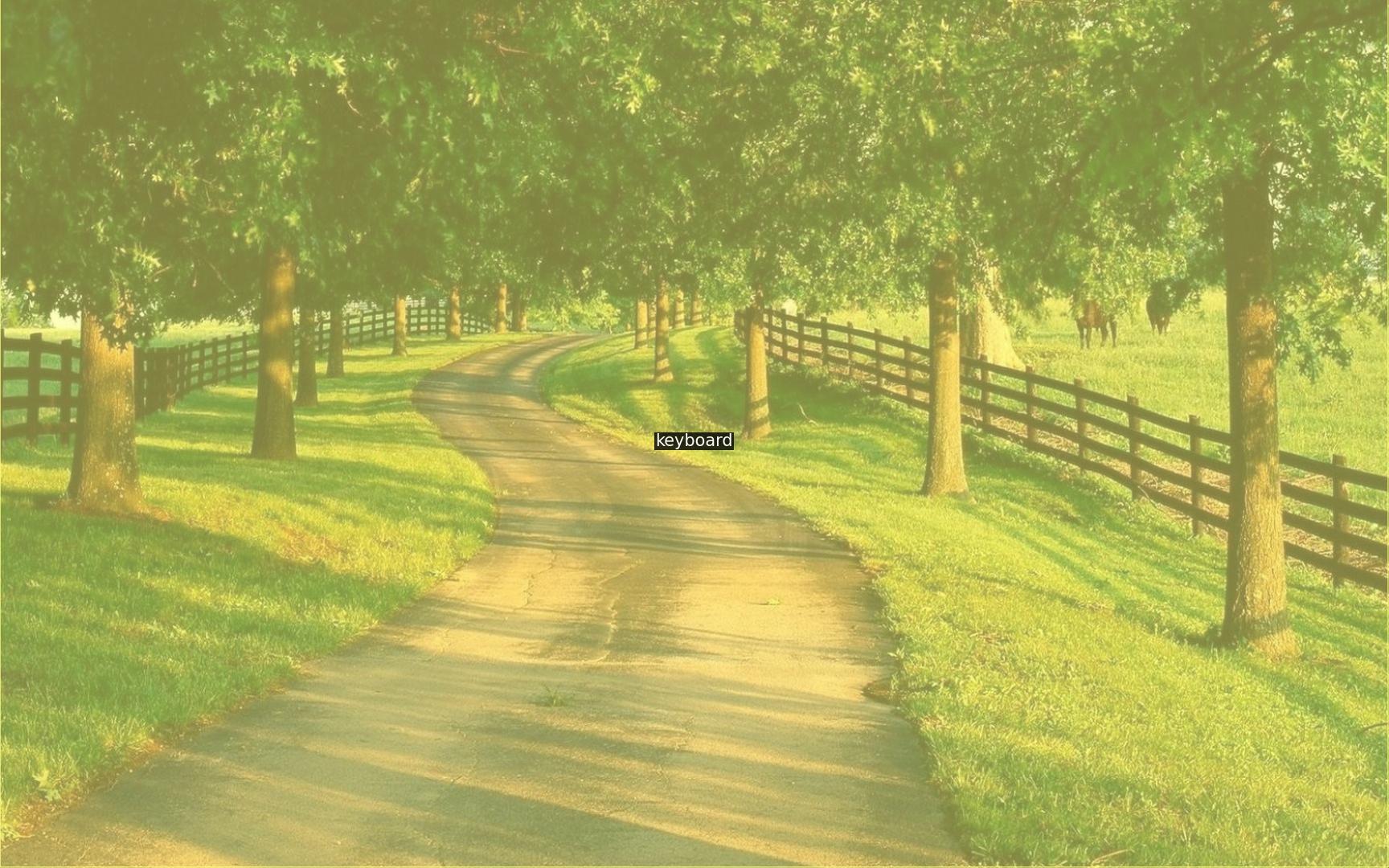
The config is: python3 -u mseg_semantic/tool/universal_demo.py --config=mseg_semantic/config/test/default_config_360_ms.yaml model_name mseg-3m model_path mseg-3m.pth input_file dirtroad10.jpg
Could you please tell me where the problem is? Thanks!