Open Soumennn opened 5 years ago
Same error
Is it possible for you to share the traceback for this error?
when you run that command plz try this one python2 convfeatures.py --data_path Dataset/flickr30k-images/ --inception_path ConvNets/inception_v4.pb i hope this will work fine. thank you
when you run that command plz try this one python2 convfeatures.py --data_path Dataset/flickr30k-images/ --inception_path ConvNets/inception_v4.pb i hope this will work fine. thank you
Thank you ! I found there was a '/' lost behind data_path , but still an IndexError in main.py --mode train
Traceback (most recent call last):
File "main.py", line 66, in
could you please send me the lines from 62 to 71 Sreenu.Padidapu, Jr,Software Engineer, Aadhya Analytics, 2-39,Old SBI Road, Sri nagar colony, Gannavaram-521101.
On Wed, May 22, 2019 at 10:05 AM dljzx notifications@github.com wrote:
when you run that command plz try this one python2 convfeatures.py --data_path Dataset/flickr30k-images/ --inception_path ConvNets/inception_v4.pb i hope this will work fine. thank you
Thank you ! I found there was a '/' lost behind data_path , but still an IndexError in main.py --mode train Traceback (most recent call last): File "main.py", line 66, in features, captions = training_data[:, 0], training_data[:, 1] IndexError: too many indices for array Could you help me solve that question? Thanks a lot.
— You are receiving this because you commented. Reply to this email directly, view it on GitHub https://github.com/neural-nuts/image-caption-generator/issues/38?email_source=notifications&email_token=AKDKCK3XIQGJAXAU7AXZVGLPWTERXA5CNFSM4HEPBIZ2YY3PNVWWK3TUL52HS4DFVREXG43VMVBW63LNMVXHJKTDN5WW2ZLOORPWSZGODV5323Y#issuecomment-494648687, or mute the thread https://github.com/notifications/unsubscribe-auth/AKDKCK7WAW6H5II7KN547GTPWTERXANCNFSM4HEPBIZQ .
could you please send me the lines from 62 to 71 Sreenu.Padidapu, Jr,Software Engineer, Aadhya Analytics, 2-39,Old SBI Road, Sri nagar colony, Gannavaram-521101. … On Wed, May 22, 2019 at 10:05 AM dljzx @.***> wrote: when you run that command plz try this one python2 convfeatures.py --data_path Dataset/flickr30k-images/ --inception_path ConvNets/inception_v4.pb i hope this will work fine. thank you Thank you ! I found there was a '/' lost behind data_path , but still an IndexError in main.py --mode train Traceback (most recent call last): File "main.py", line 66, in features, captions = training_data[:, 0], training_data[:, 1] IndexError: too many indices for array Could you help me solve that question? Thanks a lot. — You are receiving this because you commented. Reply to this email directly, view it on GitHub <#38?email_source=notifications&email_token=AKDKCK3XIQGJAXAU7AXZVGLPWTERXA5CNFSM4HEPBIZ2YY3PNVWWK3TUL52HS4DFVREXG43VMVBW63LNMVXHJKTDN5WW2ZLOORPWSZGODV5323Y#issuecomment-494648687>, or mute the thread https://github.com/notifications/unsubscribe-auth/AKDKCK7WAW6H5II7KN547GTPWTERXANCNFSM4HEPBIZQ .
if config.mode == "train": vocab, wtoidx, training_data = generate_captions( config.word_threshold, config.max_len, args.caption_path, args.feature_path, config.data_is_coco) features, captions = training_data[:, 0], training_data[:, 1] features = np.array([feat.astype(float) for feat in features]) data = (vocab.tolist(), wtoidx.tolist(), features, captions) model = Caption_Generator(config, data=data) loss, inp_dict = model.build_train_graph() model.train(loss, inp_dict)
These are the lines you need. It is so kind of you to respond me so quick.
i think all are correct here could you send me the caption_generator.py file it will more helpful for me. Thanks, Sreenu.Padidapu, Jr,Software Engineer, Aadhya Analytics, 2-39,Old SBI Road, Sri nagar colony, Gannavaram-521101.
On Wed, May 22, 2019 at 10:17 AM dljzx notifications@github.com wrote:
could you please send me the lines from 62 to 71 Sreenu.Padidapu, Jr,Software Engineer, Aadhya Analytics, 2-39,Old SBI Road, Sri nagar colony, Gannavaram-521101. … <#m7082800599257854298> On Wed, May 22, 2019 at 10:05 AM dljzx @.***> wrote: when you run that command plz try this one python2 convfeatures.py --data_path Dataset/flickr30k-images/ --inception_path ConvNets/inception_v4.pb i hope this will work fine. thank you Thank you ! I found there was a '/' lost behind data_path , but still an IndexError in main.py --mode train Traceback (most recent call last): File "main.py", line 66, in features, captions = training_data[:, 0], training_data[:, 1] IndexError: too many indices for array Could you help me solve that question? Thanks a lot. — You are receiving this because you commented. Reply to this email directly, view it on GitHub <#38 https://github.com/neural-nuts/image-caption-generator/issues/38?email_source=notifications&email_token=AKDKCK3XIQGJAXAU7AXZVGLPWTERXA5CNFSM4HEPBIZ2YY3PNVWWK3TUL52HS4DFVREXG43VMVBW63LNMVXHJKTDN5WW2ZLOORPWSZGODV5323Y#issuecomment-494648687>, or mute the thread https://github.com/notifications/unsubscribe-auth/AKDKCK7WAW6H5II7KN547GTPWTERXANCNFSM4HEPBIZQ .
if config.mode == "train": vocab, wtoidx, training_data = generate_captions( config.word_threshold, config.max_len, args.caption_path, args.feature_path, config.data_is_coco) features, captions = training_data[:, 0], training_data[:, 1] features = np.array([feat.astype(float) for feat in features]) data = (vocab.tolist(), wtoidx.tolist(), features, captions) model = Caption_Generator(config, data=data) loss, inp_dict = model.build_train_graph() model.train(loss, inp_dict)
These are the lines you need. It is so kind of you to respond me so quick.
— You are receiving this because you commented. Reply to this email directly, view it on GitHub https://github.com/neural-nuts/image-caption-generator/issues/38?email_source=notifications&email_token=AKDKCK5EDPVKJIRICLRK4Q3PWTF5JA5CNFSM4HEPBIZ2YY3PNVWWK3TUL52HS4DFVREXG43VMVBW63LNMVXHJKTDN5WW2ZLOORPWSZGODV54JKI#issuecomment-494650537, or mute the thread https://github.com/notifications/unsubscribe-auth/AKDKCK2GBKH4PMLSW6XFE4DPWTF5JANCNFSM4HEPBIZQ .
i think all are correct here could you send me the caption_generator.py file it will more helpful for me. Thanks, Sreenu.Padidapu, Jr,Software Engineer, Aadhya Analytics, 2-39,Old SBI Road, Sri nagar colony, Gannavaram-521101. … On Wed, May 22, 2019 at 10:17 AM dljzx @.> wrote: could you please send me the lines from 62 to 71 Sreenu.Padidapu, Jr,Software Engineer, Aadhya Analytics, 2-39,Old SBI Road, Sri nagar colony, Gannavaram-521101. … <#m7082800599257854298> On Wed, May 22, 2019 at 10:05 AM dljzx @.> wrote: when you run that command plz try this one python2 convfeatures.py --data_path Dataset/flickr30k-images/ --inception_path ConvNets/inception_v4.pb i hope this will work fine. thank you Thank you ! I found there was a '/' lost behind data_path , but still an IndexError in main.py --mode train Traceback (most recent call last): File "main.py", line 66, in features, captions = training_data[:, 0], training_data[:, 1] IndexError: too many indices for array Could you help me solve that question? Thanks a lot. — You are receiving this because you commented. Reply to this email directly, view it on GitHub <#38 <#38>?email_source=notifications&email_token=AKDKCK3XIQGJAXAU7AXZVGLPWTERXA5CNFSM4HEPBIZ2YY3PNVWWK3TUL52HS4DFVREXG43VMVBW63LNMVXHJKTDN5WW2ZLOORPWSZGODV5323Y#issuecomment-494648687>, or mute the thread https://github.com/notifications/unsubscribe-auth/AKDKCK7WAW6H5II7KN547GTPWTERXANCNFSM4HEPBIZQ . if config.mode == "train": vocab, wtoidx, training_data = generate_captions( config.word_threshold, config.max_len, args.caption_path, args.feature_path, config.data_is_coco) features, captions = training_data[:, 0], training_data[:, 1] features = np.array([feat.astype(float) for feat in features]) data = (vocab.tolist(), wtoidx.tolist(), features, captions) model = Caption_Generator(config, data=data) loss, inp_dict = model.build_train_graph() model.train(loss, inp_dict) These are the lines you need. It is so kind of you to respond me so quick. — You are receiving this because you commented. Reply to this email directly, view it on GitHub <#38?email_source=notifications&email_token=AKDKCK5EDPVKJIRICLRK4Q3PWTF5JA5CNFSM4HEPBIZ2YY3PNVWWK3TUL52HS4DFVREXG43VMVBW63LNMVXHJKTDN5WW2ZLOORPWSZGODV54JKI#issuecomment-494650537>, or mute the thread https://github.com/notifications/unsubscribe-auth/AKDKCK2GBKH4PMLSW6XFE4DPWTF5JANCNFSM4HEPBIZQ .
caption_generator.zip Here is caption_generator.py
instead of that file plz replace this one
import matplotlib.pyplot as plt from random import shuffle from convfeatures import * import tensorflow as tf from PIL import Image import numpy as np import pickle import sys import os
class Caption_Generator():
def __init__(self, config, data=None):
self.dim_imgft = np.int(config.dim_imgft)
self.embedding_size = np.int(config.embedding_size)
self.num_hidden = np.int(config.num_hidden)
self.batch_size = np.int(config.batch_size)
self.num_timesteps = np.int(config.num_timesteps)
self.max_len = config.max_len
self.word_threshold = config.word_threshold
self.bias_init = config.bias_init
self.xavier_init = config.xavier_init
self.dropout = config.dropout
self.lstm_keep_prob = config.lstm_keep_prob
self.beta = config.beta_l2
self.mode = config.mode
self.batch_decode = config.batch_decode
self.learning_rate = config.learning_rate
self.resume = config.resume
self.savedecoder = config.savedecoder
self.saveencoder = config.saveencoder
if self.mode == 'train':
self.vocab, self.wtoidx, self.features, self.captions = data
self.num_batch = int(self.features.shape[0]) / self.batch_size
print("Converting Captions to IDs")
self.captions = self.Words_to_IDs(self.wtoidx, self.captions)
if self.resume == 1:
self.vocab = np.load("Dataset/vocab.npy").tolist()
self.wtoidx = np.load("Dataset/wordmap.npy").tolist()
self.current_epoch = 0
self.current_step = 0
if self.resume is 1 or self.mode == 'test':
if os.path.isfile('model/save.npy'):
self.current_epoch, self.current_step = np.load(
"model/save.npy")
else:
print("No Checkpoints, Restarting Training..")
self.resume = 0
self.nb_epochs = config.nb_epochs
if self.mode == 'test':
self.vocab = np.load("Dataset/vocab.npy").tolist()
self.wtoidx = np.load("Dataset/wordmap.npy").tolist()
self.max_words = np.int(len(self.wtoidx))
self.idxtow = dict(zip(self.wtoidx.values(),
self.wtoidx.keys())) self.model() self.image_features, self.IDs = self.build_decode_graph() self.load_image=config.load_image if not self.batch_decode: self.io = build_prepro_graph(config.inception_path) self.sess = self.init_decode() return
self.max_words = np.int(len(self.wtoidx))
self.idxtow = dict(zip(self.wtoidx.values(), self.wtoidx.keys()))
self.model()
def Words_to_IDs(self, wtoidx, caption_batch):
for i, caption in enumerate(caption_batch):
cap = []
for word in caption.split():
try:
cap.append(wtoidx[word])
except KeyError:
cap.append(wtoidx["<UNK>"])
caption_batch[i] = np.array(cap)
return np.vstack(caption_batch)
def IDs_to_Words(self, idxtow, ID_batch):
return [idxtow[word] for IDs in ID_batch for word in IDs]
def generate_mask(self, ID_batch, wtoidx):
nonpadded = map(lambda x: len(
ID_batch[0]) - x.count(wtoidx["<PAD>"]), ID_batch.tolist())
ID_batch = np.zeros((ID_batch.shape[0], self.max_len + 2))
for ind, row in enumerate(ID_batch):
row[:nonpadded[ind]] = 1
return ID_batch
def get_next_batch(self):
batch_size = self.batch_size
for batch_idx in range(0, len(self.features), batch_size):
images_batch = self.features[batch_idx:batch_idx + batch_size]
caption_batch = self.captions[batch_idx:batch_idx + batch_size]
# print caption_batch
yield images_batch, caption_batch
# From NeuralTalk by Andrej Karpathy
def init_bias(self):
bias_init_vector = np.array(
[1.0 * self.vocab[self.idxtow[i]] for i in self.idxtow])
bias_init_vector /= np.sum(bias_init_vector)
bias_init_vector = np.log(bias_init_vector)
bias_init_vector -= np.max(bias_init_vector)
return bias_init_vector
def assign_weights(self, dim1, dim2=None, name=None, Xavier=False):
if Xavier:
weight_initializer = tf.contrib.layers.xavier_initializer()
return tf.get_variable(
name, [dim1, dim2], initializer=weight_initializer)
return tf.Variable(tf.truncated_normal([dim1, dim2]),
name=name)
def assign_biases(self, dim, name, bias_init=False):
if bias_init:
return tf.Variable(self.init_bias().astype(np.float32),
name=name) return tf.Variable(tf.zeros([dim]), name=name)
def model(self):
self.word_embedding = {
"weights": self.assign_weights(
self.max_words,
self.embedding_size,
'Weight_emb'),
"biases": self.assign_biases(
self.embedding_size,
"Bias_emb")}
self.image_embedding = {
"weights": self.assign_weights(
self.dim_imgft,
self.embedding_size,
'Weight_img_emb'),
"biases": self.assign_biases(
self.embedding_size,
'Bias_img_emb')}
self.target_word = {
"weights": self.assign_weights(
self.embedding_size,
self.max_words,
'Weight_target'),
"biases": self.assign_biases(
self.max_words,
'Bias_target', bias_init=self.bias_init)}
self.lstm_cell = tf.contrib.rnn.BasicLSTMCell(self.num_hidden)
if self.dropout:
self.lstm_cell = tf.contrib.rnn.DropoutWrapper(
self.lstm_cell, self.lstm_keep_prob, self.lstm_keep_prob)
self.inp_dict = {
"features": tf.placeholder(
tf.float32, [self.batch_size, self.dim_imgft],
name="Train_Features"), "captions": tf.placeholder( tf.int32, [self.batch_size, self.num_timesteps], name="Train_Captions"), "mask": tf.placeholder( tf.float32, [self.batch_size, self.num_timesteps], name="Train_Mask") }
def create_feed_dict(self, Ids, features, mask, mode="train"):
feed_dict = {}
feed_dict[self.inp_dict['captions']] = Ids
feed_dict[self.inp_dict['features']] = features
feed_dict[self.inp_dict['mask']] = mask
return feed_dict
def build_train_graph(self):
init_c = tf.zeros([self.batch_size, self.lstm_cell.state_size[0]])
init_h = tf.zeros([self.batch_size, self.lstm_cell.state_size[1]])
initial_state = (init_c, init_h)
image_emb = tf.matmul(self.inp_dict["features"],
self.image_embedding[ 'weights']) + self.image_embedding['biases'] with tf.variable_scope("LSTM"): output, state = self.lstm_cell(image_emb, initial_state) loss = 0.0 for i in range(1, self.num_timesteps): batch_embed = tf.nn.embedding_lookup( self.word_embedding['weights'], self.inp_dict['captions'][ :, i - 1]) + self.word_embedding['biases'] tf.get_variable_scope().reuse_variables() output, state = self.lstm_cell(batch_embed, state) words = tf.reshape(self.inp_dict['captions'][ :, i], shape=[self.batch_size, 1]) onehot_encoded = tf.one_hot(indices=words, depth=len( self.wtoidx), on_value=1, off_value=0, axis=-1) onehot_encoded = tf.reshape(onehot_encoded, shape=[ self.batch_size, self.max_words]) target_logit = tf.matmul( output, self.target_word['weights']) + self.target_word['biases'] cross_entropy = tf.nn.softmax_cross_entropy_with_logits( logits=target_logit, labels=onehot_encoded) cross_entropy = cross_entropy * self.inp_dict["mask"][:, i] current_loss = tf.reduce_sum(cross_entropy) loss = loss + current_loss loss = loss / tf.reduce_sum(self.inp_dict["mask"][:, 1:])
# self.beta=0
#l2_loss = self.beta * sum([tf.nn.l2_loss(tf_var) for tf_var in
tf.trainable_variables() if not "Bias" in tf_var.name])
return loss, self.inp_dict
def build_decode_graph(self):
image_features = tf.placeholder(
tf.float32, [1, self.dim_imgft], name='Input_Features')
image_emb = tf.matmul(image_features, self.image_embedding[
'weights']) + self.image_embedding['biases']
init_c = tf.zeros([1, self.lstm_cell.state_size[0]])
init_h = tf.zeros([1, self.lstm_cell.state_size[1]])
initial_state = (init_c, init_h)
IDs = []
with tf.variable_scope("LSTM"):
output, state = self.lstm_cell(image_emb, initial_state)
pred_ID = tf.nn.embedding_lookup(
self.word_embedding['weights'], [
self.wtoidx["<S>"]]) + self.word_embedding['biases']
for i in range(self.num_timesteps):
tf.get_variable_scope().reuse_variables()
output, state = self.lstm_cell(pred_ID, state)
logits = tf.matmul(output, self.target_word[
"weights"]) + self.target_word["biases"]
predicted_next_idx = tf.argmax(logits, axis=1)
pred_ID = tf.nn.embedding_lookup(
self.word_embedding['weights'], predicted_next_idx)
pred_ID = pred_ID + self.word_embedding['biases']
predicted_next_idx = tf.cast(predicted_next_idx, tf.int32,
name="word_"+str(i)) IDs.append(predicted_next_idx)
with open("model/Decoder/DecoderOutputs.txt", 'w') as f:
for name in IDs:
f.write(name.name.split(":0")[0] + "\n")
return image_features, IDs
def train(self, loss, inp_dict):
self.loss = loss
self.inp_dict = inp_dict
saver = tf.train.Saver(max_to_keep=10)
global_step = tf.Variable(
self.current_step,
name='global_step')
starter_learning_rate = self.learning_rate
learning_rate = tf.train.exponential_decay(
starter_learning_rate, global_step, 100000, 0.95,
staircase=True) optimizer = tf.train.AdamOptimizer(learning_rate).minimize( self.loss, global_step=global_step) tf.summary.scalar("loss", self.loss) tf.summary.scalar("learning_rate", learning_rate) summary_op = tf.summary.merge_all()
with tf.Session() as sess:
print("Initializing Training")
init = tf.global_variables_initializer()
sess.run(init)
if self.resume is 1:
print("Loading Previously Trained Model")
print(self.current_epoch, "Out of", self.nb_epochs,
"Completed in previous run.") try: ckpt_file = "./model/model.ckpt-" + str(self.current_step) saver.restore(sess, ckpt_file) print("Resuming Training") except Exception as e: print(str(e).split('\n')[0]) print("Checkpoints not found") sys.exit(0) writer = tf.summary.FileWriter( "model/log_dir/", graph=tf.get_default_graph())
for epoch in range(self.current_epoch, self.nb_epochs):
loss=[]
idx = np.random.permutation(self.features.shape[0])
self.captions = self.captions[idx]
self.features = self.features[idx]
batch_iter = self.get_next_batch()
for batch_idx in xrange(self.num_batch):
batch_features, batch_Ids = batch_iter.next()
batch_mask = self.generate_mask(batch_Ids, self.wtoidx)
run = [global_step, optimizer, self.loss, summary_op]
feed_dict = self.create_feed_dict(
batch_Ids, batch_features, batch_mask)
step, _, current_loss, summary = sess.run(
run, feed_dict=feed_dict)
writer.add_summary(summary, step)
if step % 100 == 0:
print(epoch, ": Global Step:", step, "\tLoss: ",
current_loss) loss.append(current_loss) print() print("Epoch: ", epoch, "\tAverage Loss: ", np.mean(loss)) print("\nSaving Model..\n") saver.save(sess, "./model/model.ckpt", global_step=global_step) np.save("model/save", (epoch, step))
def init_decode(self):
saver = tf.train.Saver()
ckpt_file = "./model/model.ckpt-" + str(self.current_step)
sess = tf.Session()
init = tf.global_variables_initializer()
sess.run(init)
saver.restore(sess, ckpt_file)
return sess
def decode(self, path):
features = get_features(self.sess, self.io, path, self.saveencoder)
caption_IDs = self.sess.run(
self.IDs, feed_dict={
self.image_features: features})
sentence = " ".join(self.IDs_to_Words(self.idxtow, caption_IDs))
sentence = sentence.split("</S>")[0]
if self.load_image:
plt.imshow(Image.open(path))
plt.axis("off")
plt.title(sentence, fontsize='10', loc='left')
name=path.split("/")[-1]
plt.savefig("./results/"+"gen_"+name)
plt.show()
else:
print(sentence)
if self.savedecoder:
saver = tf.train.Saver()
saver.save(self.sess, "model/Decoder/model.ckpt")
#return path, sentence
def batch_decoder(self, filenames, features):
saver = tf.train.Saver()
ckpt_file = "./model/model.ckpt-" + str(self.current_step)
sentences = []
filenames = np.unique(filenames)
with open("model/Decoder/Generated_Captions.txt", 'w') as f:
with tf.Session() as sess:
init = tf.global_variables_initializer()
sess.run(init)
saver.restore(sess, ckpt_file)
for i, feat in enumerate(features):
feat = np.reshape(feat, newshape=(1, 1536))
caption_IDs = sess.run(
self.IDs, feed_dict={
self.image_features: feat})
sentence = " ".join(
self.IDs_to_Words(
self.idxtow, caption_IDs))
sentence = sentence.split("</S>")[0]
if i % 1000 == 0:
print("Progress", i, "out of", features.shape[0])
f.write(filenames[i] + "\t" + sentence + "\n")
Sreenu.Padidapu, Jr,Software Engineer, Aadhya Analytics, 2-39,Old SBI Road, Sri nagar colony, Gannavaram-521101.
On Wed, May 22, 2019 at 10:31 AM dljzx notifications@github.com wrote:
i think all are correct here could you send me the captiongenerator.py file it will more helpful for me. Thanks, Sreenu.Padidapu, Jr,Software Engineer, Aadhya Analytics, 2-39,Old SBI Road, Sri nagar colony, Gannavaram-521101. … <#m-5093071406617138178_> On Wed, May 22, 2019 at 10:17 AM dljzx @.> wrote: could you please send me the lines from 62 to 71 Sreenu.Padidapu, Jr,Software Engineer, Aadhya Analytics, 2-39,Old SBI Road, Sri nagar colony, Gannavaram-521101. … <#m7082800599257854298> On Wed, May 22, 2019 at 10:05 AM dljzx @.> wrote: when you run that command plz try this one python2 convfeatures.py --data_path Dataset/flickr30k-images/ --inception_path ConvNets/inception_v4.pb i hope this will work fine. thank you Thank you ! I found there was a '/' lost behind data_path , but still an IndexError in main.py --mode train Traceback (most recent call last): File "main.py", line 66, in features, captions = training_data[:, 0], training_data[:, 1] IndexError: too many indices for array Could you help me solve that question? Thanks a lot. — You are receiving this because you commented. Reply to this email directly, view it on GitHub <#38 https://github.com/neural-nuts/image-caption-generator/issues/38 <#38 https://github.com/neural-nuts/image-caption-generator/issues/38>?email_source=notifications&email_token=AKDKCK3XIQGJAXAU7AXZVGLPWTERXA5CNFSM4HEPBIZ2YY3PNVWWK3TUL52HS4DFVREXG43VMVBW63LNMVXHJKTDN5WW2ZLOORPWSZGODV5323Y#issuecomment-494648687>, or mute the thread https://github.com/notifications/unsubscribe-auth/AKDKCK7WAW6H5II7KN547GTPWTERXANCNFSM4HEPBIZQ . if config.mode == "train": vocab, wtoidx, training_data = generate_captions( config.word_threshold, config.max_len, args.caption_path, args.feature_path, config.data_is_coco) features, captions = training_data[:, 0], training_data[:, 1] features = np.array([feat.astype(float) for feat in features]) data = (vocab.tolist(), wtoidx.tolist(), features, captions) model = Caption_Generator(config, data=data) loss, inp_dict = model.build_train_graph() model.train(loss, inp_dict) These are the lines you need. It is so kind of you to respond me so quick. — You are receiving this because you commented. Reply to this email directly, view it on GitHub <#38 https://github.com/neural-nuts/image-caption-generator/issues/38?email_source=notifications&email_token=AKDKCK5EDPVKJIRICLRK4Q3PWTF5JA5CNFSM4HEPBIZ2YY3PNVWWK3TUL52HS4DFVREXG43VMVBW63LNMVXHJKTDN5WW2ZLOORPWSZGODV54JKI#issuecomment-494650537>, or mute the thread https://github.com/notifications/unsubscribe-auth/AKDKCK2GBKH4PMLSW6XFE4DPWTF5JANCNFSM4HEPBIZQ .
caption_generator.zip https://github.com/neural-nuts/image-caption-generator/files/3205684/caption_generator.zip Here is caption_generator.py
— You are receiving this because you commented. Reply to this email directly, view it on GitHub https://github.com/neural-nuts/image-caption-generator/issues/38?email_source=notifications&email_token=AKDKCK3YI64O5XJXNHCNUYLPWTHRVA5CNFSM4HEPBIZ2YY3PNVWWK3TUL52HS4DFVREXG43VMVBW63LNMVXHJKTDN5WW2ZLOORPWSZGODV543QI#issuecomment-494652865, or mute the thread https://github.com/notifications/unsubscribe-auth/AKDKCK36K6PMUAQFDX3QJOTPWTHRVANCNFSM4HEPBIZQ .
instead of that file plz replace this one ...
Thank you for your effort. I have tried your code but still the same error...
when you run that command plz try this one python2 convfeatures.py --data_path Dataset/flickr30k-images/ --inception_path ConvNets/inception_v4.pb i hope this will work fine. thank you
Thank you ! I found there was a '/' lost behind data_path , but still an IndexError in main.py --mode train Traceback (most recent call last): File "main.py", line 66, in features, captions = training_data[:, 0], training_data[:, 1] IndexError: too many indices for array Could you help me solve that question? Thanks a lot.
It appears that training_data
is only one-dimensional. Although, I am not sure why that should be the case.
You should try verifying the shape of training_data
, and whether features
and captions
are being assigned correct values.
NotFoundError (see above for traceback): flickr30k_images/ff1000092795.jpg; No such file or directory [[node ReadFile (defined at convfeatures.py:24) ]] [[node DecodeJpeg (defined at convfeatures.py:25) ]]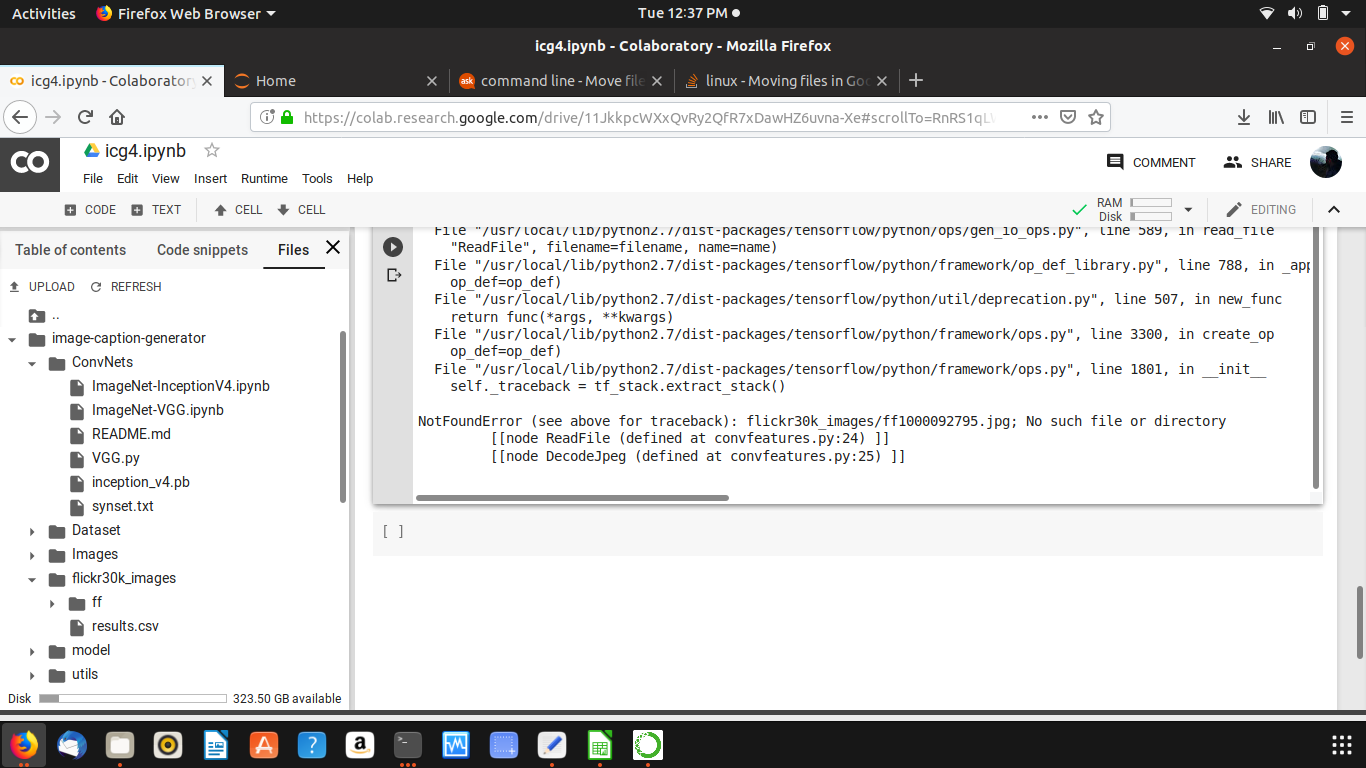
please help me with this issue as i am trying to make my college project with this. Also a python3 version migration would have helped a lot.