Open lijoe123 opened 2 years ago
And the detail is:
2022-06-14 12:32:30,844 - mmdet - INFO - Environment info:
------------------------------------------------------------
sys.platform: linux
Python: 3.7.13 (default, Mar 29 2022, 02:18:16) [GCC 7.5.0]
CUDA available: True
GPU 0: NVIDIA GeForce RTX 2080 Ti
CUDA_HOME: /usr
NVCC: Cuda compilation tools, release 10.1, V10.1.24
GCC: gcc (Ubuntu 9.3.0-17ubuntu1~20.04) 9.3.0
PyTorch: 1.11.0
PyTorch compiling details: PyTorch built with:
- GCC 7.3
- C++ Version: 201402
- Intel(R) oneAPI Math Kernel Library Version 2021.4-Product Build 20210904 for Intel(R) 64 architecture applications
- Intel(R) MKL-DNN v2.5.2 (Git Hash a9302535553c73243c632ad3c4c80beec3d19a1e)
- OpenMP 201511 (a.k.a. OpenMP 4.5)
- LAPACK is enabled (usually provided by MKL)
- NNPACK is enabled
- CPU capability usage: AVX2
- CUDA Runtime 11.3
- NVCC architecture flags: -gencode;arch=compute_37,code=sm_37;-gencode;arch=compute_50,code=sm_50;-gencode;arch=compute_60,code=sm_60;-gencode;arch=compute_61,code=sm_61;-gencode;arch=compute_70,code=sm_70;-gencode;arch=compute_75,code=sm_75;-gencode;arch=compute_80,code=sm_80;-gencode;arch=compute_86,code=sm_86;-gencode;arch=compute_37,code=compute_37
- CuDNN 8.2
- Magma 2.5.2
- Build settings: BLAS_INFO=mkl, BUILD_TYPE=Release, CUDA_VERSION=11.3, CUDNN_VERSION=8.2.0, CXX_COMPILER=/opt/rh/devtoolset-7/root/usr/bin/c++, CXX_FLAGS= -Wno-deprecated -fvisibility-inlines-hidden -DUSE_PTHREADPOOL -fopenmp -DNDEBUG -DUSE_KINETO -DUSE_FBGEMM -DUSE_QNNPACK -DUSE_PYTORCH_QNNPACK -DUSE_XNNPACK -DSYMBOLICATE_MOBILE_DEBUG_HANDLE -DEDGE_PROFILER_USE_KINETO -O2 -fPIC -Wno-narrowing -Wall -Wextra -Werror=return-type -Wno-missing-field-initializers -Wno-type-limits -Wno-array-bounds -Wno-unknown-pragmas -Wno-sign-compare -Wno-unused-parameter -Wno-unused-function -Wno-unused-result -Wno-unused-local-typedefs -Wno-strict-overflow -Wno-strict-aliasing -Wno-error=deprecated-declarations -Wno-stringop-overflow -Wno-psabi -Wno-error=pedantic -Wno-error=redundant-decls -Wno-error=old-style-cast -fdiagnostics-color=always -faligned-new -Wno-unused-but-set-variable -Wno-maybe-uninitialized -fno-math-errno -fno-trapping-math -Werror=format -Wno-stringop-overflow, LAPACK_INFO=mkl, PERF_WITH_AVX=1, PERF_WITH_AVX2=1, PERF_WITH_AVX512=1, TORCH_VERSION=1.11.0, USE_CUDA=ON, USE_CUDNN=ON, USE_EXCEPTION_PTR=1, USE_GFLAGS=OFF, USE_GLOG=OFF, USE_MKL=ON, USE_MKLDNN=OFF, USE_MPI=OFF, USE_NCCL=ON, USE_NNPACK=ON, USE_OPENMP=ON, USE_ROCM=OFF,
TorchVision: 0.12.0
OpenCV: 4.5.5
MMCV: 1.5.2
MMCV Compiler: GCC 7.3
MMCV CUDA Compiler: 11.3
MMDetection: 2.25.0+
------------------------------------------------------------
2022-06-14 12:32:32,466 - mmdet - INFO - Distributed training: False
2022-06-14 12:32:34,057 - mmdet - INFO - Config:
dataset_type = 'CocoDataset'
data_root = '/home/student/Downloads/mmdetection-master2.25/data/coco/'
img_norm_cfg = dict(
mean=[123.675, 116.28, 103.53], std=[58.395, 57.12, 57.375], to_rgb=True)
train_pipeline = [
dict(type='LoadImageFromFile'),
dict(type='LoadAnnotations', with_bbox=True),
dict(type='RandomFlip', flip_ratio=0.5),
dict(
type='AutoAugment',
policies=[[{
'type':
'Resize',
'img_scale': [(480, 1333), (512, 1333), (544, 1333), (576, 1333),
(608, 1333), (640, 1333), (672, 1333), (704, 1333),
(736, 1333), (768, 1333), (800, 1333)],
'multiscale_mode':
'value',
'keep_ratio':
True
}],
[{
'type': 'Resize',
'img_scale': [(400, 1333), (500, 1333), (600, 1333)],
'multiscale_mode': 'value',
'keep_ratio': True
}, {
'type': 'RandomCrop',
'crop_type': 'absolute_range',
'crop_size': (384, 600),
'allow_negative_crop': True
}, {
'type':
'Resize',
'img_scale': [(480, 1333), (512, 1333), (544, 1333),
(576, 1333), (608, 1333), (640, 1333),
(672, 1333), (704, 1333), (736, 1333),
(768, 1333), (800, 1333)],
'multiscale_mode':
'value',
'override':
True,
'keep_ratio':
True
}]]),
dict(
type='Normalize',
mean=[123.675, 116.28, 103.53],
std=[58.395, 57.12, 57.375],
to_rgb=True),
dict(type='Pad', size_divisor=1),
dict(type='DefaultFormatBundle'),
dict(type='Collect', keys=['img', 'gt_bboxes', 'gt_labels'])
]
test_pipeline = [
dict(type='LoadImageFromFile'),
dict(
type='MultiScaleFlipAug',
img_scale=(1333, 800),
flip=False,
transforms=[
dict(type='Resize', keep_ratio=True),
dict(type='RandomFlip'),
dict(
type='Normalize',
mean=[123.675, 116.28, 103.53],
std=[58.395, 57.12, 57.375],
to_rgb=True),
dict(type='Pad', size_divisor=1),
dict(type='ImageToTensor', keys=['img']),
dict(type='Collect', keys=['img'])
])
]
data = dict(
samples_per_gpu=2,
workers_per_gpu=2,
train=dict(
type='CocoDataset',
ann_file=
'/home/student/Downloads/mmdetection-master2.25/data/coco/annotations/train.json',
img_prefix=
'/home/student/Downloads/mmdetection-master2.25/data/coco/train2023/',
pipeline=[
dict(type='LoadImageFromFile'),
dict(type='LoadAnnotations', with_bbox=True),
dict(type='RandomFlip', flip_ratio=0.5),
dict(
type='AutoAugment',
policies=[[{
'type':
'Resize',
'img_scale': [(480, 1333), (512, 1333), (544, 1333),
(576, 1333), (608, 1333), (640, 1333),
(672, 1333), (704, 1333), (736, 1333),
(768, 1333), (800, 1333)],
'multiscale_mode':
'value',
'keep_ratio':
True
}],
[{
'type': 'Resize',
'img_scale': [(400, 1333), (500, 1333),
(600, 1333)],
'multiscale_mode': 'value',
'keep_ratio': True
}, {
'type': 'RandomCrop',
'crop_type': 'absolute_range',
'crop_size': (384, 600),
'allow_negative_crop': True
}, {
'type':
'Resize',
'img_scale': [(480, 1333), (512, 1333),
(544, 1333), (576, 1333),
(608, 1333), (640, 1333),
(672, 1333), (704, 1333),
(736, 1333), (768, 1333),
(800, 1333)],
'multiscale_mode':
'value',
'override':
True,
'keep_ratio':
True
}]]),
dict(
type='Normalize',
mean=[123.675, 116.28, 103.53],
std=[58.395, 57.12, 57.375],
to_rgb=True),
dict(type='Pad', size_divisor=1),
dict(type='DefaultFormatBundle'),
dict(type='Collect', keys=['img', 'gt_bboxes', 'gt_labels'])
]),
val=dict(
type='CocoDataset',
ann_file=
'/home/student/Downloads/mmdetection-master2.25/data/coco/annotations/val.json',
img_prefix=
'/home/student/Downloads/mmdetection-master2.25/data/coco/val2023/',
pipeline=[
dict(type='LoadImageFromFile'),
dict(
type='MultiScaleFlipAug',
img_scale=(1333, 800),
flip=False,
transforms=[
dict(type='Resize', keep_ratio=True),
dict(type='RandomFlip'),
dict(
type='Normalize',
mean=[123.675, 116.28, 103.53],
std=[58.395, 57.12, 57.375],
to_rgb=True),
dict(type='Pad', size_divisor=1),
dict(type='ImageToTensor', keys=['img']),
dict(type='Collect', keys=['img'])
])
]),
test=dict(
type='CocoDataset',
ann_file=
'/home/student/Downloads/mmdetection-master2.25/data/coco/annotations/val.json',
img_prefix=
'/home/student/Downloads/mmdetection-master2.25/data/coco/val2023/',
pipeline=[
dict(type='LoadImageFromFile'),
dict(
type='MultiScaleFlipAug',
img_scale=(1333, 800),
flip=False,
transforms=[
dict(type='Resize', keep_ratio=True),
dict(type='RandomFlip'),
dict(
type='Normalize',
mean=[123.675, 116.28, 103.53],
std=[58.395, 57.12, 57.375],
to_rgb=True),
dict(type='Pad', size_divisor=1),
dict(type='ImageToTensor', keys=['img']),
dict(type='Collect', keys=['img'])
])
]))
evaluation = dict(interval=1, metric='bbox', classwise=True)
checkpoint_config = dict(interval=1)
log_config = dict(interval=50, hooks=[dict(type='TextLoggerHook')])
custom_hooks = [dict(type='NumClassCheckHook')]
dist_params = dict(backend='nccl')
log_level = 'INFO'
load_from = None
resume_from = None
workflow = [('train', 1)]
opencv_num_threads = 0
mp_start_method = 'fork'
auto_scale_lr = dict(enable=False, base_batch_size=16)
model = dict(
type='DETR',
backbone=dict(
type='ResNet',
depth=50,
num_stages=4,
out_indices=(3, ),
frozen_stages=1,
norm_cfg=dict(type='BN', requires_grad=False),
norm_eval=True,
style='pytorch',
init_cfg=dict(type='Pretrained', checkpoint='torchvision://resnet50')),
bbox_head=dict(
type='DETRHead',
num_classes=6,
in_channels=2048,
transformer=dict(
type='Transformer',
encoder=dict(
type='DetrTransformerEncoder',
num_layers=6,
transformerlayers=dict(
type='BaseTransformerLayer',
attn_cfgs=[
dict(
type='MultiheadAttention',
embed_dims=256,
num_heads=8,
dropout=0.1)
],
feedforward_channels=2048,
ffn_dropout=0.1,
operation_order=('self_attn', 'norm', 'ffn', 'norm'))),
decoder=dict(
type='DetrTransformerDecoder',
return_intermediate=True,
num_layers=6,
transformerlayers=dict(
type='DetrTransformerDecoderLayer',
attn_cfgs=dict(
type='MultiheadAttention',
embed_dims=256,
num_heads=8,
dropout=0.1),
feedforward_channels=2048,
ffn_dropout=0.1,
operation_order=('self_attn', 'norm', 'cross_attn', 'norm',
'ffn', 'norm')))),
positional_encoding=dict(
type='SinePositionalEncoding', num_feats=128, normalize=True),
loss_cls=dict(
type='CrossEntropyLoss',
bg_cls_weight=0.1,
use_sigmoid=False,
loss_weight=1.0,
class_weight=1.0),
loss_bbox=dict(type='L1Loss', loss_weight=5.0),
loss_iou=dict(type='GIoULoss', loss_weight=2.0)),
train_cfg=dict(
assigner=dict(
type='HungarianAssigner',
cls_cost=dict(type='ClassificationCost', weight=1.0),
reg_cost=dict(type='BBoxL1Cost', weight=5.0, box_format='xywh'),
iou_cost=dict(type='IoUCost', iou_mode='giou', weight=2.0))),
test_cfg=dict(max_per_img=100))
optimizer = dict(
type='AdamW',
lr=0.0025,
weight_decay=0.0001,
paramwise_cfg=dict(
custom_keys=dict(backbone=dict(lr_mult=0.1, decay_mult=1.0))))
optimizer_config = dict(grad_clip=dict(max_norm=0.1, norm_type=2))
lr_config = dict(policy='step', step=[100])
runner = dict(type='EpochBasedRunner', max_epochs=150)
work_dir = 'work_dirs/detr'
auto_resume = False
gpu_ids = [0]
Did you make any modifications to the coco dataset?
Did you make any modifications to the coco dataset?
No!!!! I use faster rcnn to train my own dataset, i can get the good mAP.
@Czm369
+1,I got the same problem when I train detr on visdrone dataset
On custom dataset, I also get the same issue, any update regarding this ?
I am facing the same issue with mmdet 3.0.0 and 3.1.0 and the config file detr_r50_8xb2-150e_coco.py.
Training the DETR architecture as specified by the config file and all AP values stay at 0.000 even after 100 epochs and a grid search over the learning rate. Other models can be trained using mmdet without any issues on my dataset:
Method | AP | AP 50 | AP 75 |
---|---|---|---|
DETR | 0.000 | 0.000 | 0.000 |
Faster R-CNN | 0.709 | 0.943 | 0.815 |
RetinaNet | 0.704 | 0.949 | 0.807 |
Has the DETR architecture been trained recently by anyone with a successful outcome?
Same here. I'm currently training DETR on COCO for 8epochs and its AP is still 0 :c I see many issues related to DETR AP=0, how is everyone dealing with this problem ?
@jinlovespho I am using other DETR based models from MMDetection instead:
No issues training those architectures on custom datasets so far. However the DETR problem remains unsolved for me.
I have the same issues on my custom datasets, while other models like SSD, YOLO, RetinaNet, even DAB DETR, DINO, work well.
请问您解决了吗,我在dab detr上遇到了相同的问题
When i train the detr_r50_8*2_150e_coco.py on my own coco dataset, but the ap=0?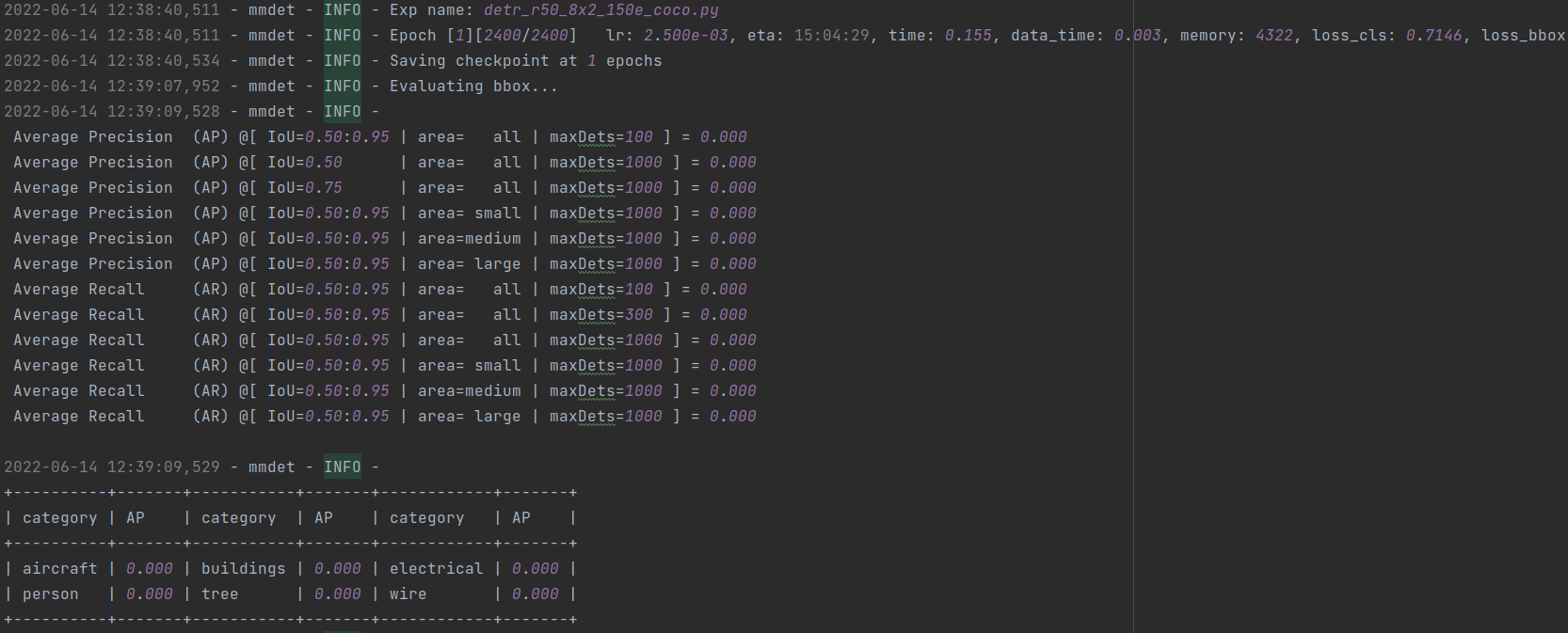