Closed monocongo closed 4 years ago
Here's are some "Car" images:
Dear @monocongo, We do not have any feedback loop for this sort of feedback. However, some of these are correct according to our definition:
Thanks for your response, @jponttuset.
Before including images from OpenImages in my dataset I first filter out all images with these attributes marked as true so none of the above images should be marked as such:
# filter out images that are occluded, truncated, group, depiction, inside, etc.
for reject_field in ("IsOccluded", "IsTruncated", "IsGroupOf", "IsDepiction", "IsInside"):
df_images = df_images[df_images[reject_field] == 0]
I realize that this dataset is free and you get what you pay for, and my thoughts were to help with the quality control as a small contriibution to the project. I don't get the impression from your response that this is of much interest, nor is there a mecahnism in place to facilitate improvements when issues are detected. If this changes or if I'm mistaken then please contact me if I can help. I have lists of images from the dataset that are problematic that I use in my own work as an exclusion filter, in case that would be useful to others. I have not been able to go through more than a couple thousand images so far but I have found roughly 10% of the images to be problematic, so it appears that the dataset could benefit from additional attention to quality control.
Hi @monocongo,
I don't get the impression from your response that this is of much interest, nor is there a mecahnism in place to facilitate improvements when issues are detected.
At the scale of Open Images, there is no easy way of incorporating this type of feedback, as we would need to verify any of the flagged content, with probably diminishing returns. I understand where you're coming from but I encourage you to think at the scale of 15 million boxes.
In any case, thank you for your feedback and for sharing the list of problematic images and I hope that Open Images is useful for your work despite its imperfections.
I'm by no means an expert, but I wonder how useful a dataset is for training models for object detection if it's as dubious as Open Images appears to be? My assumption is that this sort of quality consideration should matter more than it appears to, as there seems to be an unexpectedly/surprisingly high percentage of low-quality images/boxes in this dataset. It may be that the adage "garbage in / garbage out" counterintuitively doesn't apply so much to the areas of endeavor where this dataset might typically be used? My assumption has been that removing questionable images such as the ones shown above will result in better training outcomes when using the dataset as training input for object detection models. Perhaps I should have run some experiments to verify this assumption before pestering you guys about it.
In any event, thanks for the work you guys do to provide this dataset to the community -- while not perfect it's nevertheless quite useful. Very appreciated!
In the course of my work with images downloaded from OpenImages, I have come across a number of problematic images/bounding box annotations that I think should be removed or rectified. Is there a mechanism in place for this sort of QA?
A few examples of images from the "Person" class with wonky bounding boxes are attached below for illustration.
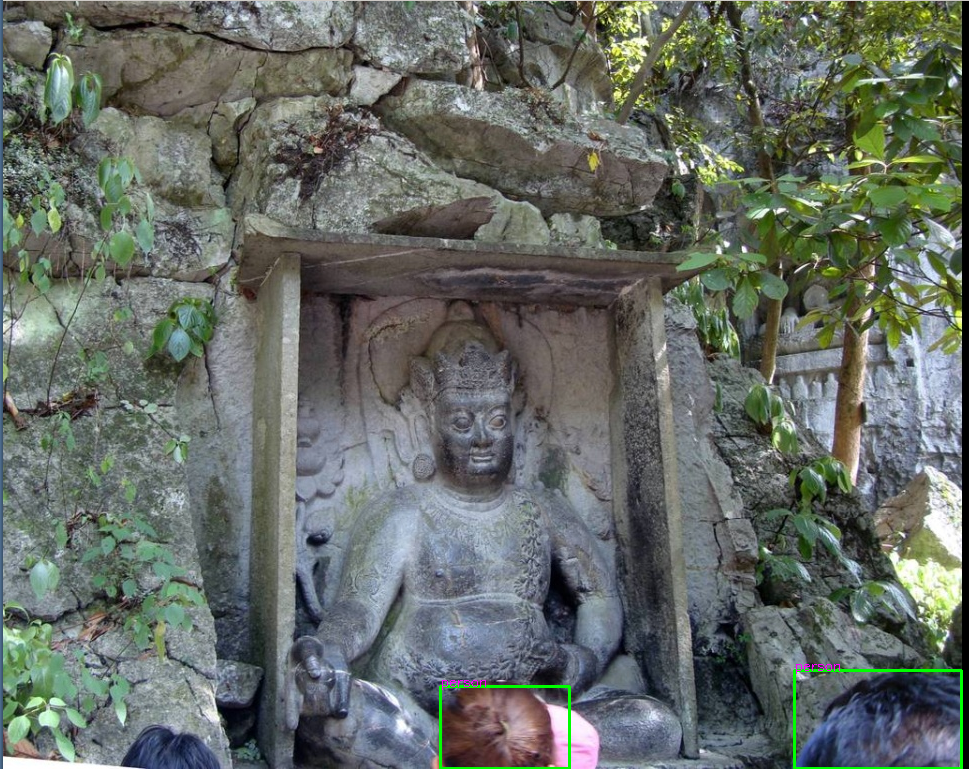