MobileNetV1, MobileNetV2, VGG based SSD/SSD-lite implementation in Pytorch 1.0 / Pytorch 0.4. Out-of-box support for retraining on Open Images dataset. ONNX and Caffe2 support. Experiment Ideas like CoordConv.
I used your pipeline to train a custom dataset with 2 classes (one as background and another class for the object type as the dataset is only for a single class). I first modified you VOC dataloader to load my custom dataset. I trained upto 30 epochs and got an mAP of about 85% and the results seemed good for baseline. The output from softmax are good as for the background class its giving high probabilities and the object class its giving low probabilities for most boxes. Eg: while debugging I did F.softmax(confidences[3], dim=2). The output was :
In the output of the first layer it detects the object class in most test images and the distance between the scores is very high as well. Here it got the output with .91, .90 where the object is and scores in rest of the bounding boxes being very low (in range of e-03 or e-04) where the object is not there.
This output is reasonable as its able to detect background in most anchor locations. Similar was the case in other outputs of confidence heads. It was getting high score for the a few anchor boxes for the object class and the output made sense.
To train and experiment more, I've integrated the your repository into my custom training pipeline by modularizing the code in such a way that the class has functions to build_net, train and eval the model.I have not made any change to the files in the vision folder for building the model , except for a few functional changes. I'm able to propely load the models trained in your implementation into my pipeline without problem. I'm able to load the model properly and initialize with pretrained weights from mb2-ssd-lite-mp-0_686.pth. But now that I have trained mobilenet2-ssd-lite in my integrated code for 30 epochs, the softmax outputs from my integrated pipeline is giving out values that are high for all the anchor boxes in object class. I again did F.softmax(confidences[3], dim=2), this is the output:
Thus it means its able to learn where the object is with high confidence but not able to properly tell where its not in the layers ahead. In confidence[0], compared to the model trained in your original implementation above, the scores of the other anchors in the one trained in my integration are not that distant. In confidence[1] - confidence[5], the confidence outputs among the object class are very close in each layers separately but in higher range. It should ideally be able to output the confidence for the anchors to be in range of 0.0xxx as its doing from your implementation. Eg: in confidence[1] all the values for class score might be 0.29xx, in confidence[3] as given above, all the values are in the range 0.32xx and it is a pattern I was seeing. Sometime it can go in the range of 0.4xxx as well for all the anchor boxes. They only differ in 3rd and 4th decimal place.
I'm getting mAP of 71% on the integrated version as well. The consequence of this is when I run inference on the test images nms ends up outputting a lot of bounding boxes all over the image. If I increase the prebability threshold to eg 0.5, all the bounding boxes in close range go away if they are below 0.5 and do end up having the object class detected. But it does miss out cases it was able to detect with the model trained in the original implementation.
The loss output from your original seem to lower in your original code. But when I'm training from my integration the losses go down as well but not as much. I used cosine lr schelduler in both the runs. I trained mobilenet-1-ssd as well from my implementation but the results are the same.
Orignal implementation loss:
Losses from my pipeline:
To me it looks like the the layers for confidence headers from confidence[1] - confidence[5] are not learning and even confidence[0] is not able to learn that well. I'm not able to clearly tell where I'm going wrong. Any suggestions will be welcome to debug such a problem. A pytorch forum thread is the closest I've found to the current problem.
Thanks for this awesome implementation!
I used your pipeline to train a custom dataset with 2 classes (one as background and another class for the object type as the dataset is only for a single class). I first modified you VOC dataloader to load my custom dataset. I trained upto 30 epochs and got an mAP of about 85% and the results seemed good for baseline. The output from softmax are good as for the background class its giving high probabilities and the object class its giving low probabilities for most boxes. Eg: while debugging I did
F.softmax(confidences[3], dim=2)
. The output was :The output for object class scores sorted
torch.sort(F.softmax(confidences[0], dim=2) [0][:,1])
is:In the output of the first layer it detects the object class in most test images and the distance between the scores is very high as well. Here it got the output with .91, .90 where the object is and scores in rest of the bounding boxes being very low (in range of e-03 or e-04) where the object is not there. This output is reasonable as its able to detect background in most anchor locations. Similar was the case in other outputs of confidence heads. It was getting high score for the a few anchor boxes for the object class and the output made sense.
To train and experiment more, I've integrated the your repository into my custom training pipeline by modularizing the code in such a way that the class has functions to build_net, train and eval the model.I have not made any change to the files in the vision folder for building the model , except for a few functional changes. I'm able to propely load the models trained in your implementation into my pipeline without problem. I'm able to load the model properly and initialize with pretrained weights from mb2-ssd-lite-mp-0_686.pth. But now that I have trained mobilenet2-ssd-lite in my integrated code for 30 epochs, the softmax outputs from my integrated pipeline is giving out values that are high for all the anchor boxes in object class. I again did
F.softmax(confidences[3], dim=2)
, this is the output:The output for
torch.sort(F.softmax(confidences[0], dim=2) [0][:,1])
isThus it means its able to learn where the object is with high confidence but not able to properly tell where its not in the layers ahead. In confidence[0], compared to the model trained in your original implementation above, the scores of the other anchors in the one trained in my integration are not that distant. In confidence[1] - confidence[5], the confidence outputs among the object class are very close in each layers separately but in higher range. It should ideally be able to output the confidence for the anchors to be in range of 0.0xxx as its doing from your implementation. Eg: in confidence[1] all the values for class score might be 0.29xx, in confidence[3] as given above, all the values are in the range 0.32xx and it is a pattern I was seeing. Sometime it can go in the range of 0.4xxx as well for all the anchor boxes. They only differ in 3rd and 4th decimal place.
I'm getting mAP of 71% on the integrated version as well. The consequence of this is when I run inference on the test images nms ends up outputting a lot of bounding boxes all over the image. If I increase the prebability threshold to eg 0.5, all the bounding boxes in close range go away if they are below 0.5 and do end up having the object class detected. But it does miss out cases it was able to detect with the model trained in the original implementation.
The loss output from your original seem to lower in your original code. But when I'm training from my integration the losses go down as well but not as much. I used cosine lr schelduler in both the runs. I trained mobilenet-1-ssd as well from my implementation but the results are the same.
Orignal implementation loss: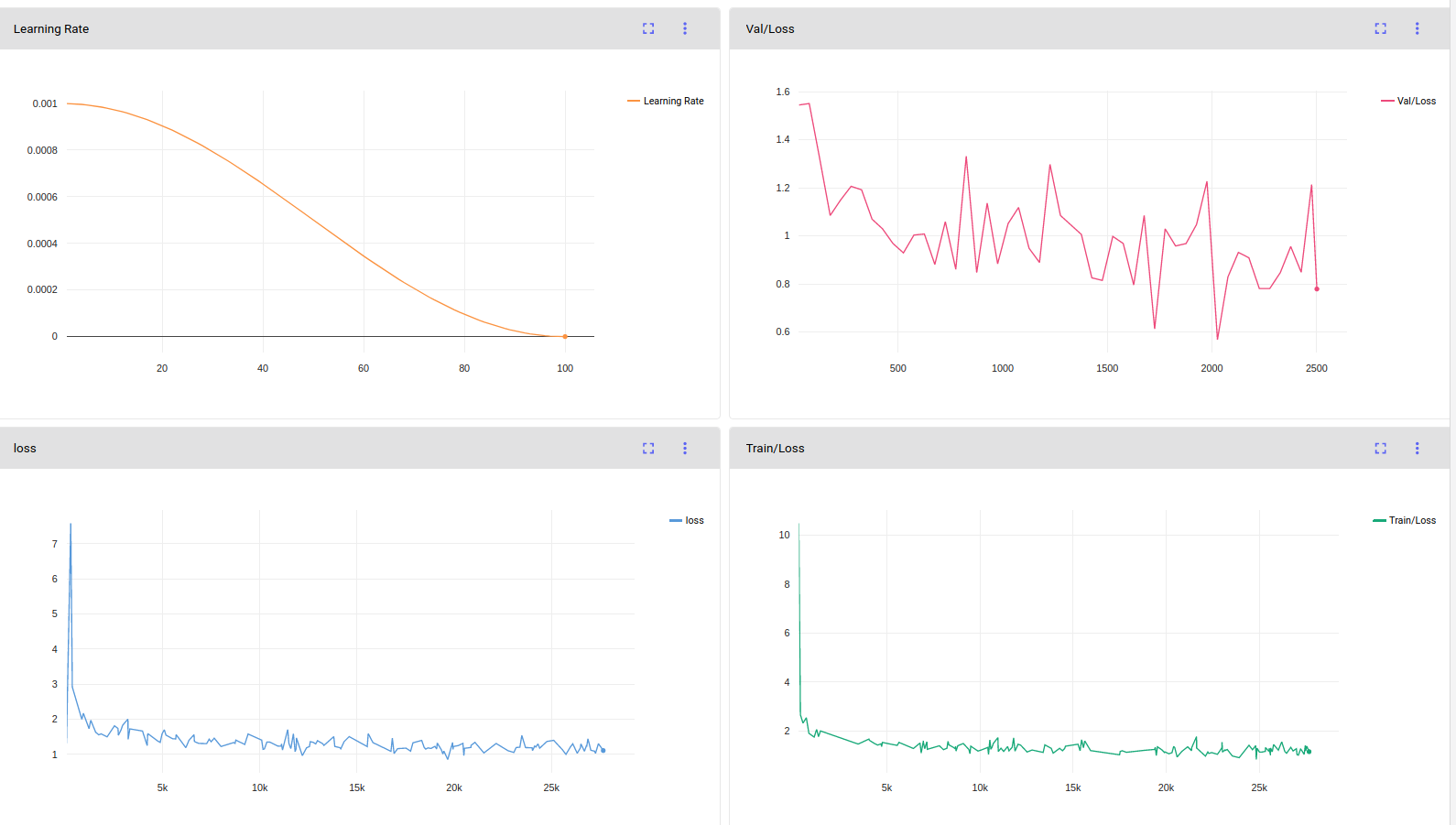
Losses from my pipeline: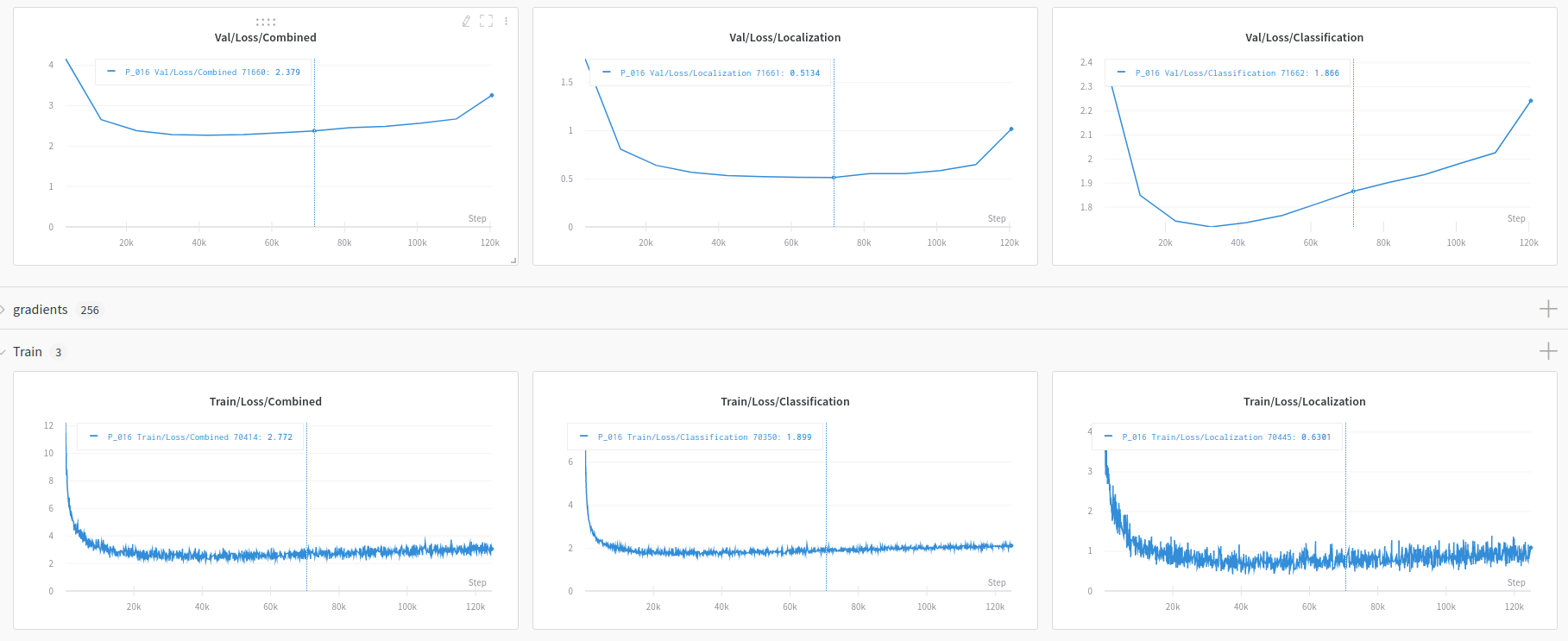
To me it looks like the the layers for confidence headers from confidence[1] - confidence[5] are not learning and even confidence[0] is not able to learn that well. I'm not able to clearly tell where I'm going wrong. Any suggestions will be welcome to debug such a problem. A pytorch forum thread is the closest I've found to the current problem.