Open tinazliu opened 6 years ago
啊,三個輸出是因為我之前在嘗試age estimation時有(classification loss, regression loss, center loss)的組合才有這樣的。另外我這個project是按照 https://kexue.fm/archives/4493 做出來的完整範例,要感謝蘇大。
根據你的error你的l2_loss是(?,?,?,?)還蠻奇怪的。四個維度都變成batchsize的感覺,先檢查一下吧。 至於fit_generator我記得要定義輸入的X和Y是多少
類似這樣
hist = model.fit_generator(generator=data_generator_centerloss(X=[x_train, y_train_a_class_value], Y=[y_train_a_class, random_y_train_a], batch_size=batch_size),
steps_per_epoch=train_num // batch_size,
validation_data=([x_test,y_test_a_class_value], [y_test_a_class, random_y_test_a]),
epochs=nb_epochs, verbose=1,
callbacks=callbacks)
data generator例子:
def data_generator_centerloss(X,Y,batch_size):
X1 = X[0]
X2 = X[1]
Y1 = Y[0]
Y2 = Y[1]
while True:
idxs = np.random.permutation(len(X1))
X1 = X1[idxs] #images
X2 = X2[idxs] #labels for center loss
Y1 = Y1[idxs]
Y2 = Y2[idxs]
p1,p2,q1,q2 = [],[],[],[]
for i in range(len(X1)):
p1.append(X1[i])
p2.append(X2[i])
q1.append(Y1[i])
q2.append(Y2[i])
if len(p1) == batch_size:
yield [np.array(p1),np.array(p2)],[np.array(q1),np.array(q2)]
p1,p2,q1,q2 = [],[],[],[]
if p1:
yield [np.array(p1),np.array(p2)],[np.array(q1),np.array(q2)]
p1,p2,q1,q2 = [],[],[],[]
大大您好: 謝謝您的回覆,我在實作時也有拜讀到蘇大的文章,真的很感謝您們兩位!! :)
這邊我還在查找,如果大大有遇過類似的問題,還望您給我一點方向!謝謝大大 ~ 如果沒有遇過也沒關係,哈哈一直打擾您真不好意思! :balloon:[更新]:balloon:
最後謝謝大大在百忙中回覆我的問題!
@shamangary hi, I wanna know if I use the function flow_flow_directory to get the data generator, How I define the Embedding's input. that means, I don't get the labels(the target_input). Can you help me to solve it? I am not sure about the embedding's input. Can I give it a one-hot vector?
@tinazliu @shamangary @lily0101 I have some problem when I use centerloss in image classification with keras. 1、in custom_vgg_model.fit(y = {'fc2':y_train,'predictions':y_train}),'fc2':y_train have error that
ValueError: Error when checking target: expected fc2 to have shape (None, 4096) but got array with shape (6300, 45)
y_train is the labels. If I do like this custom_vgg_model.fit(y = {'fc2':dummy1,'predictions':y_train}),the model will train successful. The dummy1 have same shape with 'fc2' output(feature).
dummy1 = np.zeros((y_train.shape[0],4096))
But can't improve the accuracy of the model.So it is wrong coding.
2、It is wrong to use ImageDataGenerator.flow(x = X_train, y = {'fc2':dummy1,'predictions':y_train}, batch_size=batch_Sizes) .So I can't expand my data.
image_input = Input(shape=(224, 224, 3))
model = VGG16(input_tensor=image_input, include_top=True,weights='imagenet')
model.summary()
last_layer = model.get_layer('fc2').output
feature = last_layer
out = Dense(num_classes,activation = 'softmax',name='predictions')(last_layer)
custom_vgg_model = Model(inputs = image_input, outputs = [out,feature])
custom_vgg_model.summary()
for layer in custom_vgg_model.layers[:-3]:
layer.trainable = False
custom_vgg_model.layers[3].trainable
sgd = optimizers.SGD(lr=learn_Rate,decay=decay_Rate,momentum=0.9,nesterov=True)
center_loss = lossclass.get_center_loss(alpha=0.5, num_classes=45,feature_dim = 4096)
custom_vgg_model.compile(loss={'predictions': "categorical_crossentropy", 'fc2': center_loss},
loss_weights={'fc2': 1, 'predictions': 1},optimizer= sgd,
metrics={'predictions': 'accuracy'})
t=time.time()
dummy1 = np.zeros((y_train.shape[0],4096))
dummy2 = np.zeros((y_test.shape[0],4096))
if not data_Augmentation:
hist = custom_vgg_model.fit(x = X_train,y = {'fc2':y_train,'predictions':y_train},batch_size=batch_Sizes,
epochs=epoch_Times, verbose=1,validation_data=(X_test, {'fc2':y_test,'predictions':y_test}))
else:
datagen = ImageDataGenerator(
featurewise_center=False,
samplewise_center=False,
featurewise_std_normalization=False,
samplewise_std_normalization=False,
zca_whitening=False,
rotation_range=20,
width_shift_range=0.2,
height_shift_range=0.2,
horizontal_flip=True,
vertical_flip=True,
rescale=None,
preprocessing_function=None,
data_format=None)
print('x_train.shape[0]:{:d}'.format(X_train.shape[0]))
hist = custom_vgg_model.fit_generator(datagen.flow(x = X_train, y = {'fc2':dummy1,'predictions':y_train}, batch_size=batch_Sizes),
steps_per_epoch=X_train.shape[0]/batch_Sizes,epochs=epoch_Times,
verbose=1, validation_data=(X_test, {'fc2':y_test,'predictions':y_test}))
# lossclass.py
def _center_loss_func(labels,features, alpha, num_classes, centers, feature_dim):
assert feature_dim == features.get_shape()[1]
labels = K.argmax(labels, axis=1)
labels = tf.to_int32(labels)
centers_batch = K.gather(centers, labels)
diff = (1 - alpha) * (centers_batch - features)
centers = tf.scatter_sub(centers, labels, diff)
centers_batch = K.gather(centers, labels)
loss = K.mean(K.square(features - centers_batch))
return loss
def get_center_loss(alpha, num_classes, feature_dim):
"""Center loss based on the paper "A Discriminative
Feature Learning Approach for Deep Face Recognition"
(http://ydwen.github.io/papers/WenECCV16.pdf)
"""
# Each output layer use one independed center: scope/centers
centers = K.zeros([num_classes, feature_dim], dtype='float32')
@functools.wraps(_center_loss_func)
def center_loss(y_true, y_pred):
return _center_loss_func(y_true, y_pred, alpha, num_classes, centers, feature_dim)
return center_loss
大大您好,謝謝你提供這麼棒的source code,讓我受益良多 :) 以下我有一些關於fit generator的問題,還望大大能夠解惑~
問題1:
以上是大大提供fit_generator的範本,我好奇的是為何
Y=[y_train_a_class,y_train_a, random_y_train_a]
有3個輸出 ?y_train_a
代表什麼意思? 會有這個好奇點是因為我看在TTY.mnist.py
是用.fit實踐的 -->model_centerloss.fit([x_train,y_train_value], [y_train, random_y_train], batch_size=batch_size, epochs=epochs, verbose=1, validation_data=([x_test,y_test_value], [y_test,random_y_test]), callbacks=[histories])
,照我的理解,其為雙輸入雙輸出的格式。故我覺得.fit_generator也要為雙輸入雙輸出~問題2
我依照雙輸入和雙輸出的想法建構自己的fit_generator,出現一個很奇怪的問題
以下是我輸入和輸出的 .shape,感覺大小是正確的
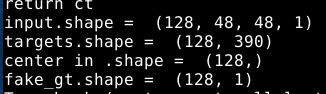
所以有點摸不著頭緒,是不是我的generator和l2_loss的格是不相符,不過我看l2_loss的型態都是?,感覺怪怪的QQ
補充
以下是我實作generator的方式:,我的generator會return這些東西
然後我的fit_generator是這樣實踐的
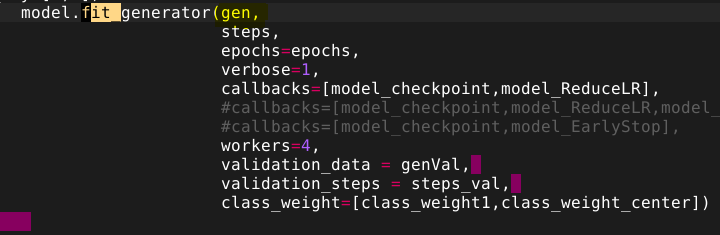
不好意思打擾您,真的很謝謝你提供那麼棒的程式,讓我在實踐center loss時有一個很棒的參考對象~謝謝
,Tina