Open pangxueyu233 opened 2 years ago
Hi Tan,
I found the chromosome name of my data had 'chr', so I modified the source data of reg3.py
to make it possible to remove the CN regions as following:
......
# presets
presets = {
"hm":[Reg("chr" + str(i + 1)) for i in range(22)] + [Reg("X"), Reg("Y")],
"hf":[Reg("chr" + str(i + 1)) for i in range(22)] + [Reg("X")],
"mm":[Reg("chr" + str(i + 1)) for i in range(19)] + [Reg("chrX"), Reg("chrY")],
"mf":[Reg("chr" + str(i + 1)) for i in range(19)] + [Reg("chrX")]}
preset_descriptions = {
"hm":"human male",
"hf":"human female",
"mm":"mouse male",
"mf":"mouse female"}
......
But when I used the dip-c reg3
to exclude the CN regions in .3dg
files, it would return errors. The codes I used are as following:
DIP_C=./programme/dip-c-master/dip-c
sample=MLL3_RS2_1
rep=1
$DIP_C reg3 -e $sample.filter.haplotype.bed -p hf $sample.20k.${rep}.3dg > $sample.20k.${rep}.filter.3dg
And it returns:
Traceback (most recent call last):
File "/mnt/data/user_data/xiangyu/programme/dip-c-master/dip-c", line 130, in <module>
main()
File "/mnt/data/user_data/xiangyu/programme/dip-c-master/dip-c", line 69, in main
return_value = reg3.reg3(sys.argv[1:])
File "/mnt/data/user_data/xiangyu/programme/dip-c-master/reg3.py", line 89, in reg3
g3d_data = file_to_g3d_data(open(args[0], "rb"))
File "/mnt/data/user_data/xiangyu/programme/dip-c-master/classes.py", line 1427, in file_to_g3d_data
g3d_data.add_g3d_particle(string_to_g3d_particle(g3d_file_line.strip()))
File "/mnt/data/user_data/xiangyu/programme/dip-c-master/classes.py", line 1214, in string_to_g3d_particle
hom_name, ref_locus, x, y, z = g3d_particle_string.split("\t")
ValueError: need more than 1 value to unpack
And I also checked the source data of reg3.py
, the error happed in this function file_to_g3d_data
. And when I execute the following codes, it would reported same errors:
import sys
import getopt
import gzip
from classes import Reg, file_to_reg_list, file_to_g3d_data, G3dData
g3d_data = file_to_g3d_data(open("./MLL3_RS2_1.20k.1.3dg", "rb"))
it returns:
Traceback (most recent call last):
File "<stdin>", line 1, in <module>
File "classes.py", line 1427, in file_to_g3d_data
g3d_data.add_g3d_particle(string_to_g3d_particle(g3d_file_line.strip()))
File "classes.py", line 1214, in string_to_g3d_particle
hom_name, ref_locus, x, y, z = g3d_particle_string.split("\t")
ValueError: need more than 1 value to unpack
Can you help me work it out?
And I had submitted my .3dg
file and abnomal CN region data.
mydata.zip
Thanks Xiangyu Pan
I have figrued it out, I found the wrong
.3dg
files were used as input. When I used the.20k.${rep}.dip-c.3dg
files, produced byhickit_3dg_to_3dg_rescale_unit.sh
, to run$DIP_C reg3
, the right output had been generated.
Thanks Xiangyu Pan
Hi Xiangyu,
Thank you for your interest in our work, and sorry about the delay in my response. Because 3D modeling is challenging for abnormal CNs (e.g. CN gain or LOH; CN loss is actually fine), I recommend removing these regions at a much earlier stage: using dip-c reg
or hickit to remove contacts from these regions before any subsequent analysis.
Best, Tan
Hi Tan,
Recently, I use dip-c
for subsequent analysis on the GM12878 data. I found the structure reconstruction were different by aligning with different version of reference (hg38 and/or hg19). The RMSD values are also changed a lot. Here is my results you could check:
1) I thought the SNP_file
would be important file for imputaion, my SNP_file
were downloaded and processed by following codes:
mwget -n 50 -c 50 ftp://platgene_ro:''@ussd-ftp.illumina.com/2017-1.0/hg38/small_variants/NA12878/NA12878.vcf.gz
mv NA12878.vcf.gz NA12878_hg38.vcf.gz
hickit.js vcf2tsv NA12878_hg38.vcf.gz > phased_SNP_hg38.tsv
mwget -n 50 -c 50 ftp://platgene_ro:''@ussd-ftp.illumina.com/2017-1.0/hg19/small_variants/NA12878/NA12878.vcf.gz
mv NA12878.vcf.gz NA12878_hg19.vcf.gz
hickit.js vcf2tsv NA12878_hg19.vcf.gz > phased_SNP_hg19.tsv
2) And I also found that l thought the structure generated from hickit_3dg_to_3dg_rescale_backbone.sh
and hickit_3dg_to_3dg_rescale_unit.sh
are similar, the RMSD values are different as following:
Because I didn't familiar the internal formula and codes in dip-c
, did you think whether it has something would affect the RMSD values and structure files .clean.3dg
by aligning with different version of reference (especially, the hg19 and hg38)
If you need the raw data, I could provide them for you.
And here is my codes for runing the sample. If you find somewhere wrong, please tell me.
cd /mnt/data/sequencedata/3D_Genome/tmp
# GENOME=/mnt/data/user_data/xiangyu/programme/genome_index/bwa_index/bwa_hg19_index/hg19.tmp
GENOME=/mnt/data/user_data/xiangyu/programme/genome_index/bwa_index/bwa_hg38_index/hg38.fa
SNP_file=/mnt/data/user_data/xiangyu/programme/dip-c-master/snps/phased_SNP_hg38.tsv
# SNP_file=/mnt/data/user_data/xiangyu/programme/dip-c-master/snps/phased_SNP_hg19.tsv
scripts=/mnt/data/user_data/xiangyu/programme/dip-c-master/scripts
output=/mnt/data/sequencedata/3D_Genome/tmp/
DIP_C=/mnt/data/user_data/xiangyu/programme/dip-c-master/dip-c
COLOR=/mnt/data/user_data/xiangyu/programme/dip-c-master/color
sample=PD10.hg38
bwa mem -5SP -t 25 -R "@RG\tID:PD10.hg38\tSM:PD10.hg38\tLB:Genome3D\tPL:Illumina" $GENOME \
/mnt/data/sequencedata/3D_Genome/tmp/PD10_XXL_pa-2_S101_L002_R1_001.fastq.gz \
/mnt/data/sequencedata/3D_Genome/tmp/PD10_XXL_pa-2_S101_L002_R2_001.fastq.gz | gzip > PD10.hg38.aln.sam.gz
mkdir /mnt/data/sequencedata/3D_Genome/tmp/sam_all
mv *.sam.gz ./sam_all
hickit.js sam2seg -v $SNP_file $output/sam_all/$sample.aln.sam.gz 2> $sample.raw.contacts.seg.log | hickit.js chronly -y - | gzip > $sample.raw.contacts.seg.gz
hickit -i $sample.raw.contacts.seg.gz -o - 2> $sample.raw.contacts.pairs.log | bgzip > $sample.raw.contacts.pairs.gz
hickit -i $sample.raw.contacts.pairs.gz -u -o - 2> $sample.raw.impute.pairs.log | bgzip > $sample.raw.impute.pairs.gz
$scripts/hickit_pairs_to_con.sh $sample.raw.contacts.pairs.gz
$scripts/hickit_impute_pairs_to_con.sh $sample.raw.impute.pairs.gz
for rep in `seq 1 3`
do
hickit -s${rep} -M -i $sample.raw.impute.pairs.gz -Sr1m -c1 -r10m -c2 -b4m \
-b1m -O $sample.raw.1m.${rep}.3dg \
-b200k -O $sample.raw.200k.${rep}.3dg \
-D5 -b50k -O $sample.raw.50k.${rep}.3dg \
-D5 -b20k -O $sample.raw.20k.${rep}.3dg
done
# hickit_3dg_to_3dg_rescale_backbone.sh
for rep in `seq 1 3`
do
$scripts/hickit_3dg_to_3dg_rescale_unit.sh $sample.raw.20k.${rep}.3dg
$DIP_C clean3 -c $sample.raw.impute.con.gz $sample.raw.20k.${rep}.dip-c.3dg > $sample.raw.20k.${rep}.clean.3dg 2> $sample.20k_clean.log # remove repetitive (contact-less) regions
$scripts/hickit_3dg_to_3dg_rescale_unit.sh $sample.raw.50k.${rep}.3dg
$DIP_C clean3 -c $sample.raw.impute.con.gz $sample.raw.50k.${rep}.dip-c.3dg > $sample.raw.50k.${rep}.clean.3dg 2> $sample.50k_clean.log # remove repetitive (contact-less) regions
$scripts/hickit_3dg_to_3dg_rescale_unit.sh $sample.raw.200k.${rep}.3dg
$DIP_C clean3 -c $sample.raw.impute.con.gz $sample.raw.200k.${rep}.dip-c.3dg > $sample.raw.200k.${rep}.clean.3dg 2> $sample.200k_clean.log # remove repetitive (contact-less) regions
$scripts/hickit_3dg_to_3dg_rescale_unit.sh $sample.raw.1m.${rep}.3dg
$DIP_C clean3 -c $sample.raw.impute.con.gz $sample.raw.1m.${rep}.dip-c.3dg > $sample.raw.1m.${rep}.clean.3dg 2> $sample.1m_clean.log # remove repetitive (contact-less) regions
done
$DIP_C align -o $sample.raw.aligned.20k. $sample.raw.20k.[1-3].clean.3dg 2> $sample.raw.20k.align.log > $sample.raw.20k.align.color
$DIP_C align -o $sample.raw.aligned.50k. $sample.raw.50k.[1-3].clean.3dg 2> $sample.raw.50k.align.log > $sample.raw.50k.align.color
$DIP_C align -o $sample.raw.aligned.200k. $sample.raw.200k.[1-3].clean.3dg 2> $sample.raw.200k.align.log > $sample.raw.200k.align.color
$DIP_C align -o $sample.raw.aligned.1m. $sample.raw.1m.[1-3].clean.3dg 2> $sample.raw.1m.align.log > $sample.raw.1m.align.color
$DIP_C color -n $COLOR/hg19.chr_new.txt $sample.raw.20k.1.clean.3dg | $DIP_C vis -c /dev/stdin $sample.raw.20k.1.clean.3dg > $sample.raw.20k.clean.n.cif
$DIP_C color -n $COLOR/hg19.chr_new.txt $sample.raw.50k.1.clean.3dg | $DIP_C vis -c /dev/stdin $sample.raw.50k.1.clean.3dg > $sample.raw.50k.clean.n.cif
$DIP_C color -n $COLOR/hg19.chr_new.txt $sample.raw.200k.1.clean.3dg | $DIP_C vis -c /dev/stdin $sample.raw.200k.1.clean.3dg > $sample.raw.200k.clean.n.cif
$DIP_C color -n $COLOR/hg19.chr_new.txt $sample.raw.1m.1.clean.3dg | $DIP_C vis -c /dev/stdin $sample.raw.1m.1.clean.3dg > $sample.raw.1m.clean.n.cif
Thanks a lot Xiangyu Pan
Hi Tan,
it's really a interesting tool to analyse the 3D genome on single-cell level. Recently, we also implemented this platform in our projects. But we met some problems. As you can see in some cells of our data, there were obvious copy-number (CN) gains or losses regions. Although you mentioned
.reg
files inREADME.md
file, I'm not sure where and how should we do to remove them.Now, I have two plans to achive it: 1) remove the CN regions by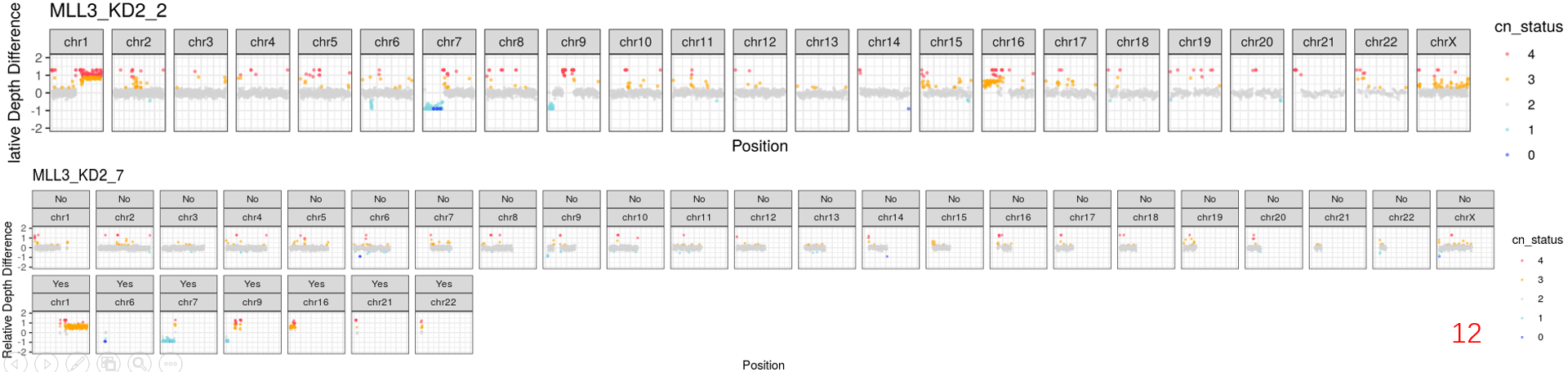
samtools
andbedtools
from*.aln.sam.gz
files; 2) remove them bydip-c reg3
. But I'm not sure which way is better, because theRMSD
is the important standard for the structure. And theRMSD
scores would be calculated based on*3dg
files. So, I prefer to remove these region by the first way to before genome structure reconstruction. Do you think whether it's resonbale to do?Sincerely Xiangyu Pan West China hospital Sichuan University Chengdu, China