Closed jindongwang closed 4 years ago
Hi, Jindong
You need to change the parameter from 0.0 (SHOT-IM) to 0.3 (SHOT), namely, --cls_par 0.3 in the command line.
Any idea why SHOT does not achieve a high performance (says, larger than 81.5 (Drop To Adapt) or 84.3 (Self-Ensemble)) on VisDA-2017?
Any idea why SHOT does not achieve a high performance (says, larger than 81.5 (Drop To Adapt) or 84.3 (Self-Ensemble)) on VisDA-2017?
Actually, since we only use the trained model from source, it is reasonable to see our results fall behind some state-of-the-art UDA methods. Based on some insights from this paper, recently we develop another new method that scores 86.2% for VisDA-C, the corresponding paper would appear on arxiv a few days later.
Thanks for your interests.
@Openning07 @tim-learn The accuracy of Drop to Adapt on VisDA using Resnet-50 is 76.2% (81.5% using Resnet-101); and the accuracy of Self-Ensemble is 84.3% using Resnet-152. We need to pay attention to the backbone networks for different methods when comparing them.
@tim-learn Thanks for your quick response! Now it works:
@Openning07 @tim-learn The accuracy of Drop to Adapt on VisDA using Resnet-50 is 76.2% (81.5% using Resnet-101); and the accuracy of Self-Ensemble is 84.3% using Resnet-152. We need to pay attention to the backbone networks for different methods when comparing them.
Thank Jindong for your reminder. As far as I know, most UDA methods adopt the ResNet101 backbone, and the best mean accuracy is reported in CAN [Contrastive Adaptation Network, CVPR2019] (87.2%, ResNet101).
The result for Art->Clipart is 55.26%... Still some gap to the number reported in the paper. I can live with that. Thanks!
So far, the results I've reproduced are listed below: Ar -> Cl: 55.4 (-1.5); Ar -> Pr: 77.9 (-0.2); Ar -> Re: 80.9 (-0.2); Cl -> Ar: 68.0 (+0.1); Cl -> Pr: 79.1 (+0.7); Cl -> Re: 78.9 (+0.8); Pr -> Ar: 67.2 (+0.2); Pr -> Cl: 55.0 (+0.4); Pr -> Re: 81.5 (-0.3); Re -> Ar: 73.8 (+0.4).
@Openning07 @jindongwang The results in this paper are averaged based on three different seeds {2019, 2020, 2021}, you can try these seeds. Besides, results in different environments may still vary.
Good!
The result for Art->Clipart is 55.26%... Still some gap to the number reported in the paper. I can live with that. Thanks!
Hello, my results are similar to those you previously obtained. Could you please share how you improved them?
Hi, thanks for the great work! I run your code on Office-Home dataset for task Art->Clipart, which should produce an accuracy of 56%+ shown in the paper. But I get the following results, which is basically ~52%. I just run your demo code and didn't change anything. Could you please tell me where I'm wrong? Thanks.
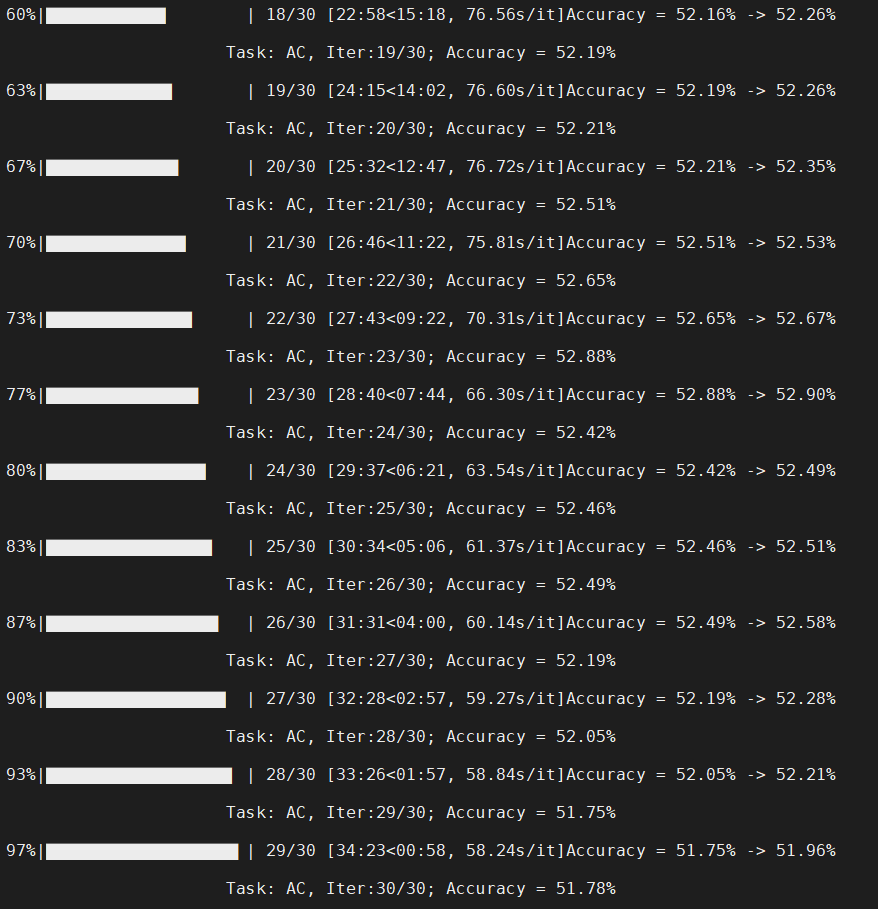