Open Carrotor116 opened 3 years ago
here is my complete test code, cus_test.py
file
import argparse
import glob
import os
import time
import cv2
import numpy as np
from HDR import *
def get_test_data_real(images_path):
# imgs_np = np.zeros([1, 3, 768, 1024, 6])
file1 = open(os.path.join(images_path, 'exposure.txt'), 'r')
Lines = file1.readlines()
t = [float(i) for i in Lines]
fs = [_ for _ in sorted(glob.glob(os.path.join(images_path, '*.tif')))]
assert len(fs) == 3
Xs = []
for j, f in enumerate(fs):
# ldr = (cv2.imread(f, -1) / 65535.0).astype(np.float32)
ldr = (cv2.imread(f, 1) / 256).astype(np.float32)
# ldr = cv2.resize(ldr, (1024, 768))
ldr = cv2.cvtColor(ldr, cv2.COLOR_BGR2RGB)
hdr = ldr ** 2.2 / (2 ** t[j])
X = np.concatenate([ldr, hdr], axis=-1)
Xs.append(X)
# imgs_np[0, j, :, :, :] = X
hdr = cv2.imread(os.path.join(images_path, 'HDRImg.hdr'), -1)[..., ::-1]
return Xs, hdr
class SimulateKalantariDataset(object):
def __init__(self, root):
self.root = root
self.scenes = [_ for _ in os.listdir(root) if os.path.isdir(os.path.join(root, _))]
assert len(self.scenes) > 0, 'invalid root: {}'.format(root)
def __len__(self):
return len(self.scenes)
@staticmethod
def zoom_image_np(image, height_max, width_max, interpolation=cv2.INTER_CUBIC):
_min, _max = np.min(image), np.max(image)
assert len(image.shape) in (2, 3), 'invalid image with shape: {}'.format(image.shape)
height_ori, width_ori = image.shape[:2]
hr = 1. * height_max / height_ori
wr = 1. * width_max / width_ori
if np.min([hr, wr]) < 1.:
r = np.min([hr, wr])
h = np.ceil(r * height_ori)
w = np.ceil(r * width_ori)
image = cv2.resize(image, dsize=(int(w), int(h)), interpolation=interpolation)
if len(image.shape) == 2:
image = image[..., np.newaxis]
image = np.clip(image, _min, _max)
return image
def _center_crop(self, x, rate=8 * 32):
x = self.zoom_image_np(x, 1400, 1400)
h, w, c = x.shape
crop_h = h // rate * rate
crop_w = w // rate * rate
j = int(round((h - crop_h) / 2.))
i = int(round((w - crop_w) / 2.))
x = x[max(0, j):min(h, j + crop_h), max(0, i):min(w, i + crop_w), :]
if x.shape[:2] != (crop_h, crop_w):
x = cv2.resize(x, (crop_w, crop_h))
return x
def __getitem__(self, item):
Xs, hdr = get_test_data_real(os.path.join(self.root, self.scenes[item]))
Xs = [self._center_crop(_) for _ in Xs] # resize image
hdr = self._center_crop(hdr) # resize reference hdr
sdr = np.stack(Xs, axis=0)
return np.expand_dims(sdr, 0), hdr, self.scenes[item]
class TimeTic(object):
def __init__(self) -> None:
self._pre = {None: time.time()}
def tic(self, tid=None, unit='s') -> float:
now = time.time()
delta = (now - self._pre[tid]) if tid in self._pre.keys() else 0.
self._pre[tid] = now
if unit == 's':
return delta
elif unit == 'ms':
return delta * 1e3
else:
raise RuntimeError('ERROR: do not support unit: {}'.format(unit))
def main(config, model):
dataset = SimulateKalantariDataset(config.test_data)
tonemap = cv2.createTonemapReinhard()
tic = TimeTic()
times = []
for idx, (SDR, hdr, name) in enumerate(dataset):
tic.tic()
rs = model.predict(SDR)
times.append(tic.tic())
out = rs[0]
print(' process {}/{}: {}, time: {:.6f}'.format(idx, len(dataset), name, times[-1]))
cv2.imwrite(os.path.join(config.result_dir, 'nhdr_{}.hdr'.format(name)), rs[0][..., ::-1])
cv2.imwrite(os.path.join(config.result_dir, 'nhdr_{}_ref.hdr'.format(name)), hdr[..., ::-1])
out = tonemap.process(out.copy())
cv2.imwrite(os.path.join(config.result_dir, 'nhdr_{}.png'.format(name)), np.uint8(out * 255)[..., ::-1])
print('avg time: {:.9f}'.format(np.mean(times)))
if __name__ == "__main__":
parser = argparse.ArgumentParser()
# Input Parameters
parser.add_argument('--test_path', type=str, default="Test/EXTRA/001/")
parser.add_argument('--gpu', type=int, default=1)
parser.add_argument('--weight_test_path', type=str, default="weights/best.h5")
parser.add_argument('--filter', type=int, default=32)
parser.add_argument('--kernel', type=int, default=3)
parser.add_argument('--encoder_kernel', type=int, default=3)
parser.add_argument('--decoder_kernel', type=int, default=4)
parser.add_argument('--triple_pass_filter', type=int, default=256)
parser.add_argument('--test_data', type=str, required=True)
parser.add_argument('--result_dir', type=str, required=True)
config = parser.parse_args()
if not os.path.exists(config.result_dir):
os.mkdir(config.result_dir)
os.environ['CUDA_VISIBLE_DEVICES'] = str(config.gpu)
model_x = NHDRRNet(config)
# x = Input(shape=(3, 256, 256, 6))
x = Input(shape=(3, 768, 1280, 6))
out = model_x.main_model(x)
model = Model(inputs=x, outputs=out)
model.load_weights(config.weight_test_path)
model.summary()
main(config, model)
test command
$ tree Test/PAPER/
Test/PAPER/
├── BarbequeDay
│ ├── 262A2943.tif
│ ├── 262A2944.tif
│ ├── 262A2945.tif
│ ├── exposure.txt
│ └── HDRImg.hdr
├── LadySitting
│ ├── 262A2705.CR2
│ ├── 262A2705.pgm
│ ├── 262A2705.tif
│ ├── 262A2706.CR2
│ ├── 262A2706.pgm
│ ├── 262A2706.tif
│ ├── 262A2707.CR2
│ ├── 262A2707.pgm
│ ├── 262A2707.tif
│ ├── exposure.txt
│ └── HDRImg.hdr
├── ManStanding
│ ├── 262A2629.tif
│ ├── 262A2630.tif
│ ├── 262A2631.tif
│ ├── exposure.txt
│ └── HDRImg.hdr
├── PeopleStanding
│ ├── 262A2866.tif
│ ├── 262A2867.tif
│ ├── 262A2868.tif
│ ├── exposure.txt
│ ├── HDRImg.hdr
└── PeopleTalking
├── 262A2810.tif
├── 262A2811.tif
├── 262A2812.tif
├── exposure.txt
└── HDRImg.hdr
5 directories, 31 files
$ python cus_test.py --weight_test_path NNHDRNet.h5 --test_data Test/PAPER/ --result_dir results --gpu -1
Hi, I clone the source code and download model weight (
NNHDRNet.h5
, size 121 MB) and test dateset provided inREADME.md
, then I tested and found result are not consistent with the picturedemo/peopleStanding.png
.Such as, for test the scene
Test/PAPER/PeopleStanding
, I modified the model input size (in line) from (3, 256, 256, 6) to (3, 768, 1280, 6) and input resized images.The result is follow:
The result provided in this repository is follow :
My test results have severe distortion.
Is this test result normal? or is the weight file the optimal weight?
can you share the weight me?
Hi, I clone the source code and download model weight (
NNHDRNet.h5
, size 121 MB) and test dateset provided inREADME.md
, then I tested and found result are not consistent with the picturedemo/peopleStanding.png
.Such as, for test the scene
Test/PAPER/PeopleStanding
, I modified the model input size (in line) from (3, 256, 256, 6) to (3, 768, 1280, 6) and input resized images.The result is follow:
The result provided in this repository is follow :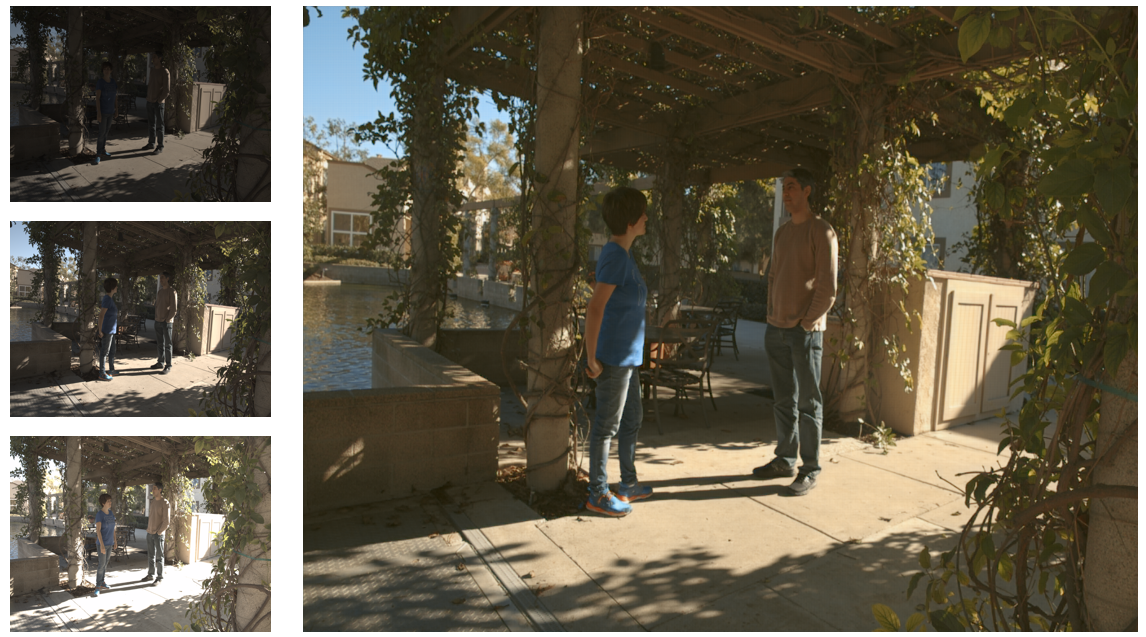
My test results have severe distortion.
Is this test result normal? or is the weight file the optimal weight?