Closed hoangnhien2411 closed 1 year ago
👋 Hello @hoangnhien2411, thank you for your interest in YOLOv5 🚀! Please visit our ⭐️ Tutorials to get started, where you can find quickstart guides for simple tasks like Custom Data Training all the way to advanced concepts like Hyperparameter Evolution.
If this is a 🐛 Bug Report, please provide a minimum reproducible example to help us debug it.
If this is a custom training ❓ Question, please provide as much information as possible, including dataset image examples and training logs, and verify you are following our Tips for Best Training Results.
Python>=3.7.0 with all requirements.txt installed including PyTorch>=1.7. To get started:
git clone https://github.com/ultralytics/yolov5 # clone
cd yolov5
pip install -r requirements.txt # install
YOLOv5 may be run in any of the following up-to-date verified environments (with all dependencies including CUDA/CUDNN, Python and PyTorch preinstalled):
If this badge is green, all YOLOv5 GitHub Actions Continuous Integration (CI) tests are currently passing. CI tests verify correct operation of YOLOv5 training, validation, inference, export and benchmarks on MacOS, Windows, and Ubuntu every 24 hours and on every commit.
Ultralytics YOLOv8 🚀 is our new cutting-edge, state-of-the-art (SOTA) model released at https://github.com/ultralytics/ultralytics. YOLOv8 is designed to be fast, accurate, and easy to use, making it an excellent choice for a wide range of object detection, image segmentation and image classification tasks. See the [YOLOv8 Docs] for details and get started with:
pip install ultralytics
👋 Hello, this issue has been automatically marked as stale because it has not had recent activity. Please note it will be closed if no further activity occurs.
Access additional YOLOv5 🚀 resources:
Access additional Ultralytics ⚡ resources:
Feel free to inform us of any other issues you discover or feature requests that come to mind in the future. Pull Requests (PRs) are also always welcomed!
Thank you for your contributions to YOLOv5 🚀 and Vision AI ⭐!
how could you solve this problem?
@whatseob, the error you are experiencing may be due to the dimensions of your image being incompatible with the mosaic operation. Please ensure that your images are all the same size and try downsampling your image, either by using a smaller training resolution or by downsampling your images outside of YOLOv5.
Also, make sure to check that the path to your mosaic9 images is correct, and try modifying the batch size or the number of mosaic images, to potentially reduce memory usage.
If the above suggestions do not help, please provide more information, including your dataset, training logs, code snippets, and any other relevant information.
I met the same error, how could you fix it?
@ckf48 hello there,
I'm sorry to hear that you are also experiencing the error. I would be happy to help you solve this problem.
The error could be caused by several factors, including an incorrect path to the mosaic9 images, incompatible image dimensions, or memory issues related to the batch size or number of mosaic images.
To resolve this error, you could try the following:
If the error persists, please provide more information, including your dataset, training logs, and code snippets, so we can better understand the issue and provide more specific guidance.
Please let me know if you have any additional questions or concerns.
@glenn-jocher Thanks for reply, but why the mosaic9 function need images in same size,while the origin mosaic4 function doesn't?
@ckf48 , you are welcome.
The mosaic9 function in YOLOv5 requires images to be of the same size because this function combines four images into a single mosaic image, which requires each input image to fit perfectly alongside the others. In contrast, the original mosaic4 function only merged two images, thus allowing for images of varying sizes.
Since the mosaic9 function combines more images, having images that are not the same size may result in image distortion and other issues that can interfere with the object detection process. Therefore, it is important to ensure that all the input images are of the same size for the mosaic9 function to work correctly.
I hope this explanation helps. Please let me know if you have any further questions or concerns.
@glenn-jocher Thanks again for reply, I think the image size is the reason, for I was training with a dataset made by myself, the problem is that nearly every shape of image in my dataset is different from each other, is there any way to resize them into the same size while training?
@ckf48 You are welcome! Yes, you could resize your images to the same size during training using OpenCV or Pillow libraries. Here's a simple example:
import cv2
# resize all images to the same size
def resize_images(images, size):
return [cv2.resize(image, size) for image in images]
# example usage
img_size = (640, 640) # size you want your images to be after resizing
train_images_resized = resize_images(train_images, img_size) # train_images is a list of your original images
In this example, we are using the cv2.resize function to resize all images to img_size
. You can adjust img_size
according to your needs.
Alternatively, you could also resize your images using the PIL (Pillow) library:
from PIL import Image
# resize all images to the same size
def resize_images(images, size):
return [image.resize(size) for image in images]
# example usage
img_size = (640, 640) # size you want your images to be after resizing
train_images_resized = resize_images(train_images, img_size) # train_images is a list of your original images
Here we use the resize function from the PIL
library to resize all images to the specified size.
I hope this helps you to resize your images to the same size while training! Let me know if you have any further questions.
@glenn-jocher Thanks a lot!I choose the cv2 version and applyed it into theload_image()
function and finally it works, I have been trapped in this problem for a week, you saved my life.
@ckf48 you are welcome! I'm glad that my suggestion worked for you and that you were able to resolve the issue you were facing. If you have any further questions or concerns, feel free to ask. We are always here to help.
Search before asking
Question
I want to try mosaic 9 for my dataset and compare to mosaic 4 . However when I use mosaic9 I got this error. How to address it?
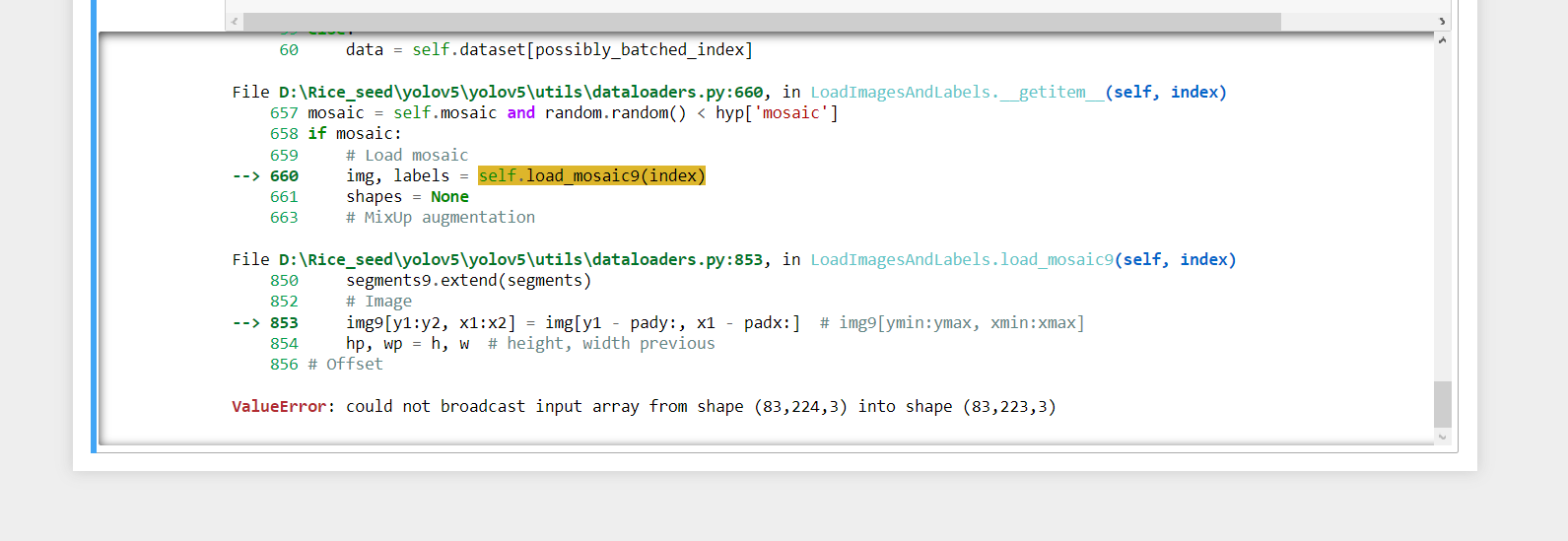
Additional
No response