Closed aiTrainee closed 2 years ago
👋 Hello @aiTrainee, thank you for your interest in YOLOv5 🚀! Please visit our ⭐️ Tutorials to get started, where you can find quickstart guides for simple tasks like Custom Data Training all the way to advanced concepts like Hyperparameter Evolution.
If this is a 🐛 Bug Report, please provide screenshots and minimum viable code to reproduce your issue, otherwise we can not help you.
If this is a custom training ❓ Question, please provide as much information as possible, including dataset images, training logs, screenshots, and a public link to online W&B logging if available.
For business inquiries or professional support requests please visit https://ultralytics.com or email support@ultralytics.com.
Python>=3.7.0 with all requirements.txt installed including PyTorch>=1.7. To get started:
git clone https://github.com/ultralytics/yolov5 # clone
cd yolov5
pip install -r requirements.txt # install
YOLOv5 may be run in any of the following up-to-date verified environments (with all dependencies including CUDA/CUDNN, Python and PyTorch preinstalled):
If this badge is green, all YOLOv5 GitHub Actions Continuous Integration (CI) tests are currently passing. CI tests verify correct operation of YOLOv5 training (train.py), validation (val.py), inference (detect.py) and export (export.py) on MacOS, Windows, and Ubuntu every 24 hours and on every commit.
@aiTrainee this is just an ONNX module terminology for a constant. You should probably start with the YOLOv3 paper by Redmon: https://arxiv.org/abs/1804.02767
Thanks for your quick response. I have already looked at YOLOv3 paper and I understand the architecture quite good.
But this part (the constant):
Isn't included in the original architecture of a tiny yolo v3. I'm not used to onnx. So merging this constant to the model doesn't impact it?
@aiTrainee oh, yes our YOLOv3-tiny architecture should be exactly identical to the original YOLOve paper.
As I said ONNX and other providers create their own translations from the PyTorch modules we create, but the end result is mathematically identical. We have benchmarking scripts that compare speed and mAP of all exported models against their PyTorch originals and you can observe identical mAP to numerical precision, i.e.: PR https://github.com/ultralytics/yolov5/pull/6613
benchmarks: weights=/content/yolov5/yolov5s.pt, imgsz=640, batch_size=1, data=/content/yolov5/data/coco128.yaml
Checking setup...
YOLOv5 🚀 v6.0-282-g8310a17 torch 1.10.0+cu111 CPU
Setup complete ✅ (8 CPUs, 51.0 GB RAM, 44.7/166.8 GB disk)
Benchmarks complete (637.94s)
Format mAP@0.5:0.95 Inference time (ms)
0 PyTorch 0.402908 111.652056
1 TorchScript 0.402908 142.402692
2 ONNX 0.402908 64.537143
3 OpenVINO 0.402908 69.528472
4 TensorRT NaN NaN
5 CoreML NaN NaN
6 TensorFlow SavedModel 0.402908 150.990861
7 TensorFlow GraphDef 0.402908 123.970838
8 TensorFlow Lite 0.402851 229.984051
9 TensorFlow Edge TPU NaN NaN
10 TensorFlow.js NaN NaN
👋 Hello, this issue has been automatically marked as stale because it has not had recent activity. Please note it will be closed if no further activity occurs.
Access additional YOLOv5 🚀 resources:
Access additional Ultralytics ⚡ resources:
Feel free to inform us of any other issues you discover or feature requests that come to mind in the future. Pull Requests (PRs) are also always welcomed!
Thank you for your contributions to YOLOv5 🚀 and Vision AI ⭐!
Search before asking
Question
Hi, I converted my trained tiny_yolo_v3.pt into onnx file. And I'm trying to understand the model.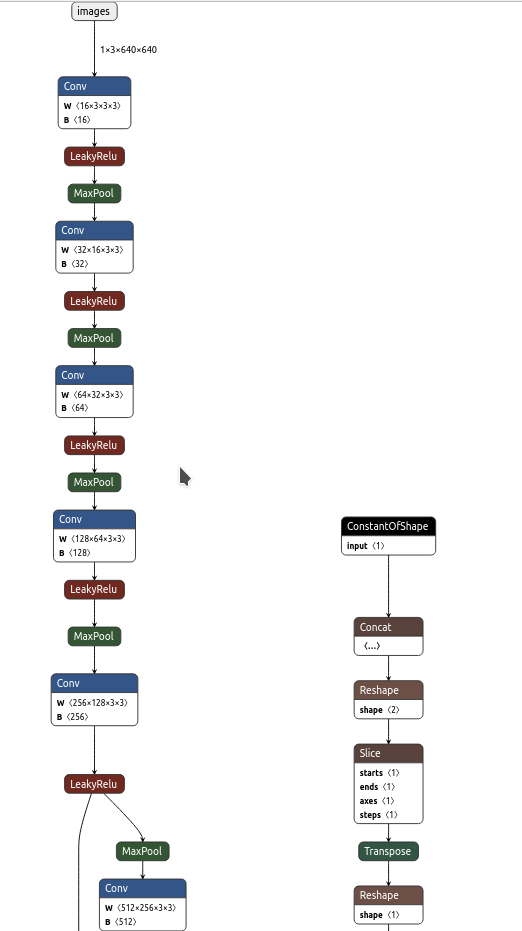
I have a problem understand the constantOfShape part. Can you please explain it's source and impact on the model. Thanks!
Additional
No response