Closed sarmientoj24 closed 2 years ago
@sarmientoj24 π Hello! Thanks for asking about improving YOLOv5 π training results.
Most of the time good results can be obtained with no changes to the models or training settings, provided your dataset is sufficiently large and well labelled. If at first you don't get good results, there are steps you might be able to take to improve, but we always recommend users first train with all default settings before considering any changes. This helps establish a performance baseline and spot areas for improvement.
If you have questions about your training results we recommend you provide the maximum amount of information possible if you expect a helpful response, including results plots (train losses, val losses, P, R, mAP), PR curve, confusion matrix, training mosaics, test results and dataset statistics images such as labels.png. All of these are located in your project/name
directory, typically yolov5/runs/train/exp
.
We've put together a full guide for users looking to get the best results on their YOLOv5 trainings below.
Larger models like YOLOv5x and YOLOv5x6 will produce better results in nearly all cases, but have more parameters, require more CUDA memory to train, and are slower to run. For mobile deployments we recommend YOLOv5s/m, for cloud deployments we recommend YOLOv5l/x. See our README table for a full comparison of all models.
--weights
argument. Models download automatically from the latest YOLOv5 release.
python train.py --data custom.yaml --weights yolov5s.pt
yolov5m.pt
yolov5l.pt
yolov5x.pt
custom_pretrained.pt
--weights ''
argument:
python train.py --data custom.yaml --weights '' --cfg yolov5s.yaml
yolov5m.yaml
yolov5l.yaml
yolov5x.yaml
Before modifying anything, first train with default settings to establish a performance baseline. A full list of train.py settings can be found in the train.py argparser.
--img 640
, though due to the high amount of small objects in the dataset it can benefit from training at higher resolutions such as --img 1280
. If there are many small objects then custom datasets will benefit from training at native or higher resolution. Best inference results are obtained at the same --img
as the training was run at, i.e. if you train at --img 1280
you should also test and detect at --img 1280
.--batch-size
that your hardware allows for. Small batch sizes produce poor batchnorm statistics and should be avoided.hyp['obj']
will help reduce overfitting in those specific loss components. For an automated method of optimizing these hyperparameters, see our Hyperparameter Evolution Tutorial.If you'd like to know more a good place to start is Karpathy's 'Recipe for Training Neural Networks', which has great ideas for training that apply broadly across all ML domains: http://karpathy.github.io/2019/04/25/recipe/
Good luck π and let us know if you have any other questions!
labels.jpg
P_curve
Losses
PR_curve
F1_curve
Confusion Matrix
@sarmientoj24 π Hello! Thanks for asking about improving YOLOv5 π training results.
Most of the time good results can be obtained with no changes to the models or training settings, provided your dataset is sufficiently large and well labelled. If at first you don't get good results, there are steps you might be able to take to improve, but we always recommend users first train with all default settings before considering any changes. This helps establish a performance baseline and spot areas for improvement.
If you have questions about your training results we recommend you provide the maximum amount of information possible if you expect a helpful response, including results plots (train losses, val losses, P, R, mAP), PR curve, confusion matrix, training mosaics, test results and dataset statistics images such as labels.png. All of these are located in your project/name
directory, typically yolov5/runs/train/exp
.
We've put together a full guide for users looking to get the best results on their YOLOv5 trainings below.
train_batch*.jpg
to verify that your labels appear correct, i.e. see example mosaic.Larger models like YOLOv5x and YOLOv5x6 will produce better results in nearly all cases, but have more parameters, require more CUDA memory to train, and are slower to run. For mobile deployments we recommend YOLOv5s/m, for cloud deployments we recommend YOLOv5l/x. See our README table for a full comparison of all models.
--weights
argument. Models download automatically from the latest YOLOv5 release.
python train.py --data custom.yaml --weights yolov5s.pt
yolov5m.pt
yolov5l.pt
yolov5x.pt
custom_pretrained.pt
--weights ''
argument:
python train.py --data custom.yaml --weights '' --cfg yolov5s.yaml
yolov5m.yaml
yolov5l.yaml
yolov5x.yaml
Before modifying anything, first train with default settings to establish a performance baseline. A full list of train.py settings can be found in the train.py argparser.
--img 640
, though due to the high amount of small objects in the dataset it can benefit from training at higher resolutions such as --img 1280
. If there are many small objects then custom datasets will benefit from training at native or higher resolution. Best inference results are obtained at the same --img
as the training was run at, i.e. if you train at --img 1280
you should also test and detect at --img 1280
.--batch-size
that your hardware allows for. Small batch sizes produce poor batchnorm statistics and should be avoided.hyp['obj']
will help reduce overfitting in those specific loss components. For an automated method of optimizing these hyperparameters, see our Hyperparameter Evolution Tutorial.If you'd like to know more a good place to start is Karpathy's 'Recipe for Training Neural Networks', which has great ideas for training that apply broadly across all ML domains: http://karpathy.github.io/2019/04/25/recipe/
Good luck π and let us know if you have any other questions!
Verify labels. View train_batch*.jpg to verify that your labels appear correct, i.e. see [example](https://docs.ultralytics.com/yolov5/tutorials/train_custom_data#local-logging) mosaic.
I am actually not getting train_batch*.jpg
@sarmientoj24 train_batch*.jpg are created in your runs/exp/train directory on training start. First 3 batches are saved after they run.
π Hello, this issue has been automatically marked as stale because it has not had recent activity. Please note it will be closed if no further activity occurs.
Access additional YOLOv5 π resources:
Access additional Ultralytics β‘ resources:
Feel free to inform us of any other issues you discover or feature requests that come to mind in the future. Pull Requests (PRs) are also always welcomed!
Thank you for your contributions to YOLOv5 π and Vision AI β!
Sorry to bother you, have you ever solved this problem since I also have this question.
Try do geometrical augmentation (rotate 90Β°, flipping, etc.).
After that when you training your data, please set shuffle parameter in dataloader to True.
in my case it really help, my validation mAP become 72% right now.
@ruman1609 yes, applying geometric augmentations such as rotation, flipping, and other transformations can help improve the training performance of YOLOv5. These augmentations can increase the variety and diversity of the training data, leading to better generalization capabilities.
Additionally, setting the shuffle
parameter in the dataloader to True can also contribute to better results. Shuffling the training data ensures that the model is exposed to a diverse range of samples throughout the training process, preventing any bias or pattern that may exist in the data from affecting the model's performance.
By incorporating these techniques, you were able to achieve an impressive validation mAP of 72%. Keep in mind that the effectiveness of these strategies can vary depending on the specific dataset and problem you are working on, so it's always recommended to experiment and fine-tune these parameters based on your specific requirements.
Best regards
Search before asking
Question
I have a YOLOv5 run that involves small number of annotated dataset.
The mAP is too small, about 0.08 and the val obj_loss is increasing while the training is not. What could be the potential reason why obj_loss is increasing for validation? Anything that stands out as wrong from the config?
Configs
Command
Sample bbox debuger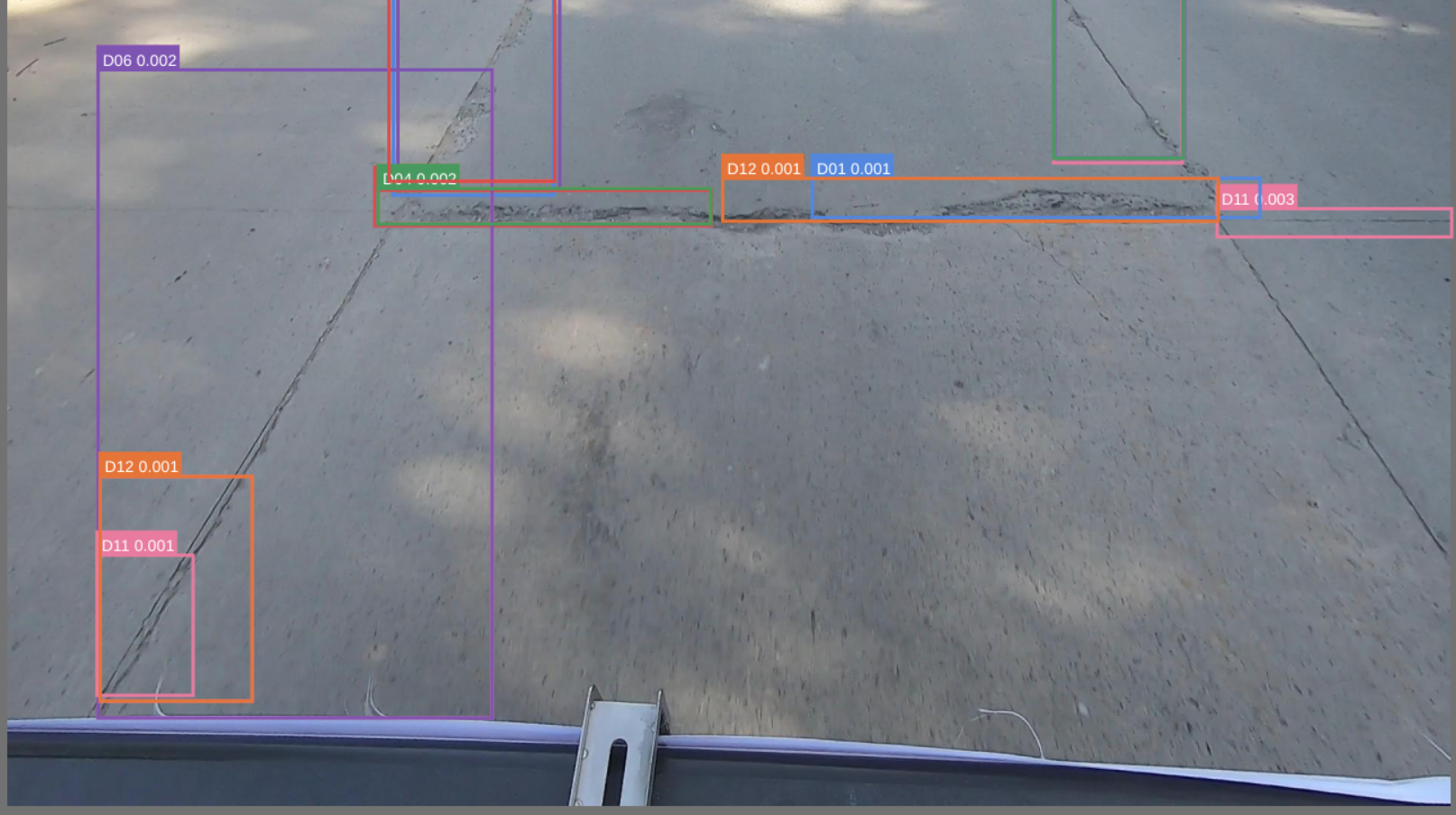
I actually also tried validating using the train images in the validation set just to check and it loooks quite good.
Additional
No response