Closed antopost closed 1 year ago
👋 Hello @antopost, thank you for your interest in YOLOv5 🚀! Please visit our ⭐️ Tutorials to get started, where you can find quickstart guides for simple tasks like Custom Data Training all the way to advanced concepts like Hyperparameter Evolution.
If this is a 🐛 Bug Report, please provide screenshots and minimum viable code to reproduce your issue, otherwise we can not help you.
If this is a custom training ❓ Question, please provide as much information as possible, including dataset images, training logs, screenshots, and a public link to online W&B logging if available.
For business inquiries or professional support requests please visit https://ultralytics.com or email support@ultralytics.com.
Python>=3.7.0 with all requirements.txt installed including PyTorch>=1.7. To get started:
git clone https://github.com/ultralytics/yolov5 # clone
cd yolov5
pip install -r requirements.txt # install
YOLOv5 may be run in any of the following up-to-date verified environments (with all dependencies including CUDA/CUDNN, Python and PyTorch preinstalled):
If this badge is green, all YOLOv5 GitHub Actions Continuous Integration (CI) tests are currently passing. CI tests verify correct operation of YOLOv5 training (train.py), validation (val.py), inference (detect.py) and export (export.py) on macOS, Windows, and Ubuntu every 24 hours and on every commit.
@antopost naturally I can't assist debugging your custom code, but I can provide a bit of advice: don't reinvent the wheel. If the official model works well, then I'd use that.
👋 Hello, this issue has been automatically marked as stale because it has not had recent activity. Please note it will be closed if no further activity occurs.
Access additional YOLOv5 🚀 resources:
Access additional Ultralytics ⚡ resources:
Feel free to inform us of any other issues you discover or feature requests that come to mind in the future. Pull Requests (PRs) are also always welcomed!
Thank you for your contributions to YOLOv5 🚀 and Vision AI ⭐!
Search before asking
Question
I want to use YOLOv5 pre-trained layer weights as part of a multi-task network. For this I saved the state_dict, loaded my custom model and overwrote the object detection layers (see below). Simply calling
model.load_state_dict
does not work, since I named my model layers differently. For inference I'm using a custom detect module.Problem: Although I use exactly the same weights and input, the results are very different (left are mine):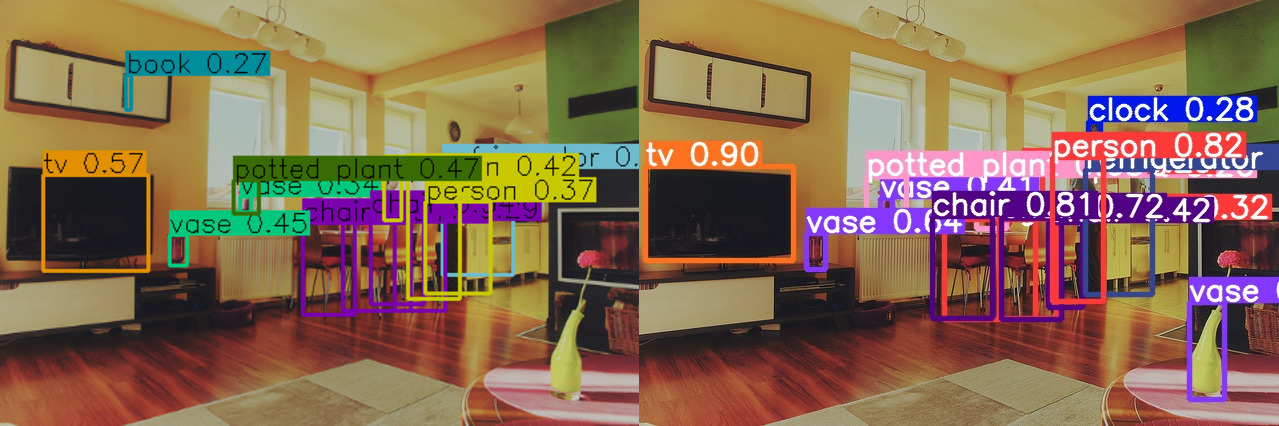
I compared some of the intermediate results from different layers and noticed that they drift apart the further the data move through the network. It seems like the error is due to rounding but both models and input are 32bit.
In my own implementation I defined my model like so (the other task heads have been omitted):
With this method I overwrote the weights of the network to work around the fact that both state_dicts have differently named keys:
The output seems to only begin to diverge after the first C3 module (see YOLOv5 architecture) or to be more exact, after (both of) the Conv
BNSiLUlayers of the C3 module. The mean absolute difference of the tensors when they first diverge is just 9.7185235e-05 which is small enough to be a rounding error. To double-check, I confirmed that the weights of the Conv layers of either implementation are exactly the same.Additional
Here are some things that I have already checked / additional info: