Open Enlistedman opened 5 years ago
Hi @Enlistedman , The model in models/lstm5/WM-1.0-test.pth is just an untrained model saved to verify my code. Sorry for the confusion, I will remove this files. Also please wait for few days as training is still going on, I will update README once it is done.
Hi @Enlistedman , The model in models/lstm5/WM-1.0-test.pth is just an untrained model saved to verify my code. Sorry for the confusion, I will remove this files. Also please wait for few days as training is still going on, I will update README once it is done.
Thank you for your reply, I am looking forward to your training results.Best wishes for you.
Hi @Enlistedman , Can you please share the evaluation script used by you?
@Enlistedman
args : dict Has all the options for changing various variables of the model as well as parameters for evaluation dataset : ImagenetDataset (torch.utils.data.Dataset, For more info see datasets/vid_dataset.py)
""" import torch from network import mvod_basenet, mvod_bottleneck_lstm1, mvod_bottleneck_lstm2, mvod_bottleneck_lstm3, mvod_lstm4, mvod_lstm5 from network.predictor import Predictor from datasets.vid_dataset import ImagenetDataset,VIDDataset from config import mobilenetv1_ssd_config from utils import box_utils, measurements from utils.misc import str2bool, Timer import argparse import pathlib import numpy as np import logging import sys import cv2
parser = argparse.ArgumentParser(description="MVOD Evaluation on VID dataset") parser.add_argument('--net', default="lstm5", help="The network architecture, it should be of basenet, lstm1, lstm2, lstm3, lstm4 or lstm5.") parser.add_argument("--trained_model", type=str) parser.add_argument("--dataset", type=str, help="The root directory of the VOC dataset or Open Images dataset.") parser.add_argument("--label_file", type=str, help="The label file path.") parser.add_argument("--use_cuda", type=str2bool, default=True) parser.add_argument("--nms_method", type=str, default="hard") parser.add_argument("--iou_threshold", type=float, default=0.5, help="The threshold of Intersection over Union.") parser.add_argument("--eval_dir", default="eval_results_lstm5", type=str, help="The directory to store evaluation results.") parser.add_argument('--width_mult', default=1.0, type=float, help='Width Multiplifier for network') args = parser.parse_args() DEVICE = torch.device("cuda:0" if torch.cuda.is_available() and args.use_cuda else "cpu")
def group_annotation_by_class(dataset): """ Groups annotations of dataset by class """ true_case_stat = {} all_gt_boxes = {} all_difficult_cases = {} for i in range(len(dataset)): image_id, annotation = dataset.get_annotation(i)
gt_boxes, classes = annotation
is_difficult = [0]
gt_boxes = torch.from_numpy(gt_boxes)
for i, difficult in enumerate(is_difficult):
class_index = int(classes[i])
gt_box = gt_boxes[i]
if not difficult:
true_case_stat[class_index] = true_case_stat.get(class_index, 0) + 1
if class_index not in all_gt_boxes:
all_gt_boxes[class_index] = {}
if image_id not in all_gt_boxes[class_index]:
all_gt_boxes[class_index][image_id] = []
all_gt_boxes[class_index][image_id].append(gt_box)
if class_index not in all_difficult_cases:
all_difficult_cases[class_index]={}
if image_id not in all_difficult_cases[class_index]:
all_difficult_cases[class_index][image_id] = []
all_difficult_cases[class_index][image_id].append(difficult)
for class_index in all_gt_boxes:
for image_id in all_gt_boxes[class_index]:
all_gt_boxes[class_index][image_id] = torch.stack(all_gt_boxes[class_index][image_id])
for class_index in all_difficult_cases:
for image_id in all_difficult_cases[class_index]:
all_gt_boxes[class_index][image_id] = torch.tensor(all_gt_boxes[class_index][image_id])
return true_case_stat, all_gt_boxes, all_difficult_cases
def compute_average_precision_per_class(num_true_cases, gt_boxes, difficult_cases, prediction_file, iou_threshold, use_2007_metric): """ Computes average precision per class """ with open(prediction_file) as f: image_ids = [] boxes = [] scores = [] for line in f: t = line.rstrip().split(" ") image_ids.append(t[0]) scores.append(float(t[1])) box = torch.tensor([float(v) for v in t[2:]]).unsqueeze(0) box -= 1.0 # convert to python format where indexes start from 0 boxes.append(box) scores = np.array(scores) sorted_indexes = np.argsort(-scores) boxes = [boxes[i] for i in sorted_indexes] image_ids = [image_ids[i] for i in sorted_indexes] true_positive = np.zeros(len(image_ids)) false_positive = np.zeros(len(image_ids)) matched = set() for i, image_id in enumerate(image_ids): box = boxes[i] if image_id not in gt_boxes: false_positive[i] = 1 continue
gt_box = gt_boxes[image_id]
ious = box_utils.iou_of(box, gt_box)
max_iou = torch.max(ious).item()
max_arg = torch.argmax(ious).item()
if max_iou > iou_threshold:
if difficult_cases[image_id][max_arg] == 0:
if (image_id, max_arg) not in matched:
true_positive[i] = 1
matched.add((image_id, max_arg))
else:
false_positive[i] = 1
else:
false_positive[i] = 1
true_positive = true_positive.cumsum()
false_positive = false_positive.cumsum()
precision = true_positive / (true_positive + false_positive)
recall = true_positive / num_true_cases
if use_2007_metric:
return measurements.compute_voc2007_average_precision(precision, recall)
else:
return measurements.compute_average_precision(precision, recall)
if name == 'main': eval_path = pathlib.Path(args.eval_dir) eval_path.mkdir(exist_ok=True) timer = Timer() class_names = [name.strip() for name in open(args.label_file).readlines()] num_classes = len(class_names) dataset = ImagenetDataset(args.dataset, is_val=True) config = mobilenetv1_ssd_config true_case_stat, all_gb_boxes, all_difficult_cases = group_annotation_by_class(dataset) if args.net == 'basenet': pred_enc = mvod_basenet.MobileNetV1(num_classes=num_classes, alpha = args.width_mult) pred_dec = mvod_basenet.SSD(num_classes=num_classes, alpha = args.width_mult, is_test=True, config= config, batch_size=1) net = mvod_basenet.MobileVOD(pred_enc, pred_dec) elif args.net == 'lstm1': pred_enc = mvod_bottleneck_lstm1.MobileNetV1(num_classes=num_classes, alpha = args.width_mult) pred_dec = mvod_bottleneck_lstm1.SSD(num_classes=num_classes, alpha = args.width_mult, is_test=True, config= config, batch_size=1) net = mvod_bottleneck_lstm1.MobileVOD(pred_enc, pred_dec) elif args.net == 'lstm2': pred_enc = mvod_bottlneck_lstm2.MobileNetV1(num_classes=num_classes, alpha = args.width_mult) pred_dec = mvod_bottlneck_lstm2.SSD(num_classes=num_classes, alpha = args.width_mult, is_test=True, config= config, batch_size=1) net = mvod_bottlneck_lstm2.MobileVOD(pred_enc, pred_dec) elif args.net == 'lstm3': pred_enc = mvod_bottlneck_lstm3.MobileNetV1(num_classes=num_classes, alpha = args.width_mult) pred_dec = mvod_bottlneck_lstm3.SSD(num_classes=num_classes, alpha = args.width_mult, is_test=True, config= config, batch_size=1) net = mvod_bottlneck_lstm3.MobileVOD(pred_enc, pred_dec) elif args.net == 'lstm4': pred_enc = mvod_lstm4.MobileNetV1(num_classes=num_classes, alpha = args.width_mult) pred_dec = mvod_lstm4.SSD(num_classes=num_classes, alpha = args.width_mult, is_test=True, config= config, batch_size=1) net = mvod_lstm4.MobileVOD(pred_enc, pred_dec) elif args.net == 'lstm5': pred_enc = mvod_lstm5.MobileNetV1(num_classes=num_classes, alpha = args.width_mult) pred_dec = mvod_lstm5.SSD(num_classes=num_classes, alpha = args.width_mult, is_test=True, config= config, batch_size=1) net = mvod_lstm5.MobileVOD(pred_enc, pred_dec) else: logging.fatal("The net type is wrong. It should be one of basenet, lstm{1,2,3,4,5}.") parser.print_help(sys.stderr) sys.exit(1)
timer.start("Load Model")
net.load_state_dict(
torch.load(args.trained_model,
map_location=lambda storage, loc: storage))
net = net.to(DEVICE)
print(f"It took {timer.end('Load Model')} seconds to load the model.")
predictor = Predictor(net, config.image_size, config.image_mean,
config.image_std,
nms_method=args.nms_method,
iou_threshold=config.iou_threshold,
candidate_size=200,
sigma=0.5,
device=DEVICE)
results = []
for i in range(len(dataset)):
print("process image", i)
timer.start("Load Image")
image = dataset.get_image(i)
print("Load Image: {:4f} seconds.".format(timer.end("Load Image")))
timer.start("Predict")
boxes, labels, probs = predictor.predict(image)
if args.net == 'basenet':
continue
else:
net.detach_hidden()
print("Prediction: {:4f} seconds.".format(timer.end("Predict")))
indexes = torch.ones(labels.size(0), 1, dtype=torch.float32) * i
results.append(torch.cat([
indexes.reshape(-1, 1),
labels.reshape(-1, 1).float(),
probs.reshape(-1, 1),
boxes + 1.0 # matlab's indexes start from 1
], dim=1))
results = torch.cat(results)
for class_index, class_name in enumerate(class_names):
if class_index == 0: continue # ignore background
prediction_path = eval_path / f"det_test_{class_name}.txt"
with open(prediction_path, "w") as f:
sub = results[results[:, 1] == class_index, :]
for i in range(sub.size(0)):
print(sub[i,:].numpy())
prob_box = sub[i, 2:].numpy()
image_id = dataset.ids[int(sub[i, 0])]
print(
image_id + " " + " ".join([str(v) for v in prob_box]),
file=f
)
aps = []
print("\n\nAverage Precision Per-class:")
for class_index, class_name in enumerate(class_names):
if class_index == 0:
continue
prediction_path = eval_path / f"det_test_{class_name}.txt"
ap = compute_average_precision_per_class(
true_case_stat[class_index],
all_gb_boxes[class_index],
all_difficult_cases[class_index],
prediction_path,
args.iou_threshold,
use_2007_metric=False
)
aps.append(ap)
print(f"{class_name}: {ap}")
print(f"\nAverage Precision Across All Classes:{sum(aps)/len(aps)}")
@vikrant7 Hello,is all the code done?
Hello,I tested the model on ILSVRC2015 using the models/lstm5/WM-1.0-test.pth you provided, but each time the scores and boxes are the same value, the final result is very poor, can you provide the corresponding help? thank you very much.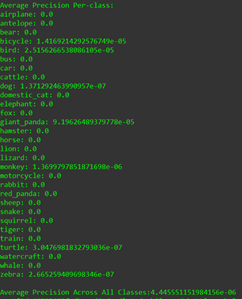