Open vincenzorusso3 opened 2 years ago
#
#
#
"""Main script to run the object detection routine.""" import argparse import sys import time
import numpy as np
import cv2 from object_detector import ObjectDetector from object_detector import ObjectDetectorOptions import utils
from motpy import Detection, MultiObjectTracker, NpImage from motpy.core import setup_logger from motpy.detector import BaseObjectDetector from motpy.testing_viz import draw_detection, draw_track from motpy.utils import ensure_packages_installed
def run(model: str, camera_id: int, width: int, height: int, num_threads: int, enable_edgetpu: bool) -> None: """Continuously run inference on images acquired from the camera.
Args: model: Name of the TFLite object detection model. camera_id: The camera id to be passed to OpenCV. width: The width of the frame captured from the camera. height: The height of the frame captured from the camera. num_threads: The number of CPU threads to run the model. enable_edgetpu: True/False whether the model is a EdgeTPU model. """
counter, fps = 0, 0 start_time = time.time()
logger = setup_logger(name, 'DEBUG', is_main=True)
model_spec = {'order_pos': 1, 'dim_pos': 2, 'order_size': 0, 'dim_size': 2, 'q_var_pos': 5000., 'r_var_pos': 0.1} dt = 1 / 5 # assume 6 fps tracker = MultiObjectTracker(dt=dt, model_spec=model_spec)
cap = cv2.VideoCapture(camera_id) cap.set(cv2.CAP_PROP_FRAME_WIDTH, width) cap.set(cv2.CAP_PROP_FRAME_HEIGHT, height) cap.set(cv2.CAP_PROP_FPS, 5)
row_size = 20 # pixels left_margin = 24 # pixels text_color = (0, 0, 255) # red font_size = 1 font_thickness = 1 fps_avg_frame_count = 10
options = ObjectDetectorOptions( num_threads=num_threads, score_threshold=0.3, max_results=3, label_allow_list=['car','truck','motorcycle'], enable_edgetpu=enable_edgetpu) detector = ObjectDetector(model_path=model, options=options)
print(model) print(num_threads) print(enable_edgetpu)
out_detections=[]
while cap.isOpened(): success, image = cap.read() if not success: sys.exit( 'ERROR: Unable to read from webcam. Please verify your webcam settings.' )
counter += 1
image = cv2.flip(image, 1)
# Run object detection estimation using the model.
detections = detector.detect(image)
out_detections=[]
for detection in detections:
#print(type(detection.bounding_box))
score= round(detection.categories[0].score, 2)
# la bbox gliela devo passare come [[x1,y1,x2,y2,score]]
new_bbox=np.array([detection.bounding_box.left,detection.bounding_box.bottom,detection.bounding_box.right,detection.bounding_box.top])
print(new_bbox)#singola bbox devo aggregare per fotogramma
#arr.append(new_bbox)
#out_detections.append(Detection(box=new_bbox, score=score))
#print(type(new_bbox))
#rint(new_bbox.shape)
det=Detection(new_bbox,score)
#print(det)
out_detections.append(det)
#track_bbs_ids=mot_tracker.update(np.array(arr))
#print(track_bbs_ids)
#print(out_detections)
tracker.step(out_detections)
#print(detections)
tracks = tracker.active_tracks(min_steps_alive=2)
#logger.debug(f'tracks: {len(tracks)}')
print(tracks)
# Draw keypoints and edges on input image
#image = utils.visualize(image, detections)
for det in out_detections:
draw_detection(image, det)
for track in tracks:
draw_track(image, track)
# Calculate the FPS
if counter % fps_avg_frame_count == 0:
end_time = time.time()
fps = fps_avg_frame_count / (end_time - start_time)
start_time = time.time()
# Show the FPS
fps_text = 'FPS = {:.1f}'.format(fps)
text_location = (left_margin, row_size)
cv2.putText(image, fps_text, text_location, cv2.FONT_HERSHEY_PLAIN,
font_size, text_color, font_thickness)
# Stop the program if the ESC key is pressed.
if cv2.waitKey(1) == 27:
break
cv2.imshow('object_detector', image)
cap.release() cv2.destroyAllWindows()
def main(): parser = argparse.ArgumentParser( formatter_class=argparse.ArgumentDefaultsHelpFormatter) parser.add_argument( '--model', help='Path of the object detection model.', required=False, default='efficientdet_lite0.tflite') parser.add_argument( '--cameraId', help='Id of camera.', required=False, type=int, default=0) parser.add_argument( '--frameWidth', help='Width of frame to capture from camera.', required=False, type=int, default=640) parser.add_argument( '--frameHeight', help='Height of frame to capture from camera.', required=False, type=int, default=480) parser.add_argument( '--numThreads', help='Number of CPU threads to run the model.', required=False, type=int, default=4) parser.add_argument( '--enableEdgeTPU', help='Whether to run the model on EdgeTPU.', action='store_true', required=False, default=False) args = parser.parse_args()
run(args.model, int(args.cameraId), args.frameWidth, args.frameHeight, int(args.numThreads), bool(args.enableEdgeTPU))
if name == 'main': main()
Dear Sir, What might be the best parameters, or acceleration model, for a setting like this: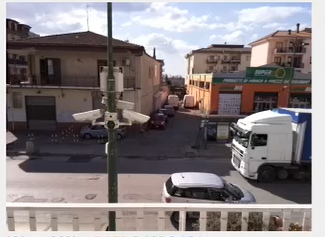
I'have tried with some attempts but I always get track-id switching, generating always new tracks
Yours Sincerely.
code.TXT