Open r3krut opened 2 years ago
@r3krut did you find a working way to do it? So far I was dealing with this by not calculating loss at those positions where I know the objects to be ignored are. But I am not sure about correctness of this solution.
@janaoravcova I have found a similar way to ignore this type of bboxes as you described. I checked this approach for single class detection problem,. Maybe, it's will not work for multi-class detection problem.
def _cn_gen_targets(annots, img_h, img_w, r=cfg.r, num_classes=cfg.num_classes):
"""
Generate targets from annots for CenterNet model(Heatmpas+Center offsets + Object sizes)
Args:
annots : ndarray of annotations in form of [x1, y1, x2, y2, class_id, ignore]
img_h : image H
img_w : image W
r : stride
num_classes : number of classes
Return:
bboxes_heatmaps - heatmap with splat centers of bboxes
bboxes_offests -
bboxes_sizes -
"""
th = img_h // r # size of target for H
tw = img_w // r # size of target for W
bboxes_heatmaps = np.zeros((th, tw, num_classes), dtype=np.float32)
centers_mask = np.zeros((th, tw), dtype=np.uint8)
ignore_mask = np.zeros((th, tw), dtype=np.uint8)
bboxes_offsets = np.zeros((th, tw, 2), dtype=np.float32)
bboxes_sizes = np.zeros((th, tw, 2), dtype=np.float32)
for annot in annots:
bbox_x1 = annot[0] # x1
bbox_y1 = annot[1] # y1
bbox_x2 = annot[2] # x2
bbox_y2 = annot[3] # y2
cls_id = int(annot[4]) # cls_id
ignore = bool(annot[5]) # 1 if ignore, else 0
aw = bbox_x2 - bbox_x1 # width of bbox
ah = bbox_y2 - bbox_y1 # height of bbox
bbox_center_x = bbox_x1 + aw / 2.0
bbox_center_y = bbox_y1 + ah / 2.0
aw_hm = aw / r
ah_hm = ah / r
gr = gaussian_radius((ah_hm, aw_hm), min_overlap=0.7)
hm_pos = np.array([bbox_center_x // r, bbox_center_y // r], np.int32) # center position of bbox in HEATMAP
hm_pos[0] = np.clip(hm_pos[0], 0, tw-1)
hm_pos[1] = np.clip(hm_pos[1], 0, th-1)
if not ignore:
draw_gaussian(bboxes_heatmaps[:, :, cls_id-1], hm_pos, int(gr))
bboxes_offsets[hm_pos[1], hm_pos[0], :] = np.array([bbox_center_x / r, bbox_center_y / r]) - hm_pos
bboxes_sizes[hm_pos[1], hm_pos[0], :] = np.log(np.array([aw / r, ah / r])) # direct regression
rr = int(gr)
centers_mask = cv2.rectangle(centers_mask, (hm_pos[0]-rr, hm_pos[1]-rr), (hm_pos[0]+rr-1, hm_pos[1]+rr-1), 1, -1)
else:
ignore_mask = cv2.rectangle(ignore_mask, (int(bbox_x1//r), int(bbox_y1//r)), (int(bbox_x2//r), int(bbox_y2//r)), 1, -1)
training_mask = (~(ignore_mask - (centers_mask & ignore_mask)).astype(np.bool)).astype(np.uint8)
# training_mask = np.ones((th, tw), dtype=np.uint8)
return numpy_to_tensor(bboxes_heatmaps).float(), \
numpy_to_tensor(bboxes_offsets).float(), \
numpy_to_tensor(bboxes_sizes).float(), \
numpy_to_tensor(np.expand_dims(training_mask, axis=-1)).float()
In loss calculation:
def forward(self, gt_hms, gt_offsets, gt_sizes,
pred_hms, pred_offsets, pred_sizes,
training_mask):
gt_hms = gt_hms * training_mask
pred_hms = pred_hms * training_mask
gt_offsets = gt_offsets * training_mask
pred_offsets = pred_offsets * training_mask
gt_sizes = gt_sizes * training_mask
pred_sizes = pred_sizes * training_mask
.....
I think, maybe you should generate a mask which ignore image as well as label. Only ignore label will infuence network performance.
Hi! Thanks for your outstanding work! I have a some question about loss calculation. Suppose, I have in my dataset some target objects which should be ignored during training. There is a right way to do this during heatmap loss calculation based on Gaussians?
Should I generate a binary training mask for pixels which belongs to "ignore" object for whole gaussian(positive+negative) or only for center(positive) pixel?
This is original image with targets visualization(red - targets, cyan - ignoring objects.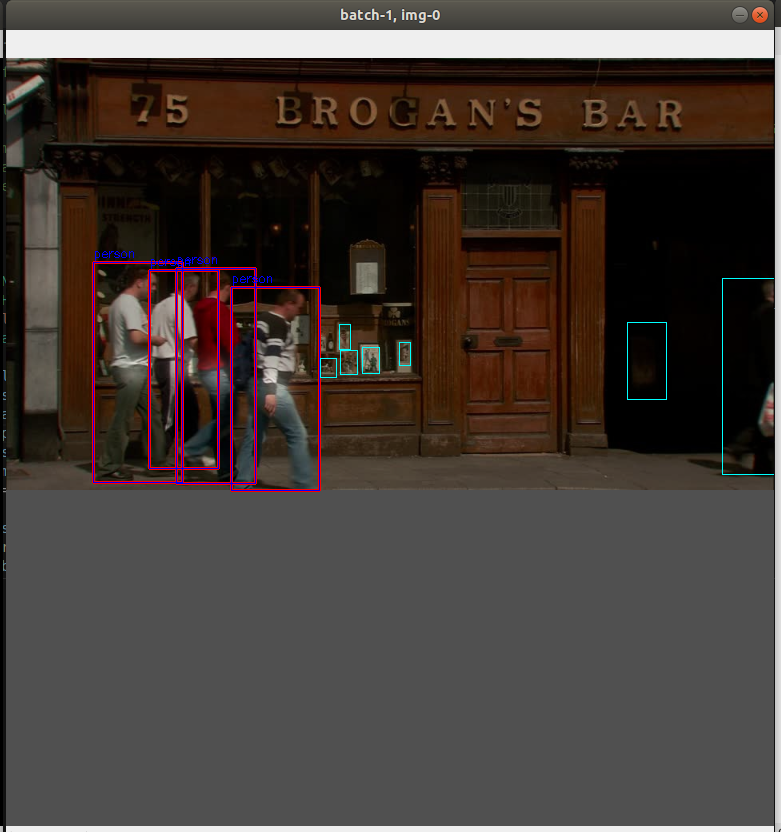
This is source heatmaps for all objects.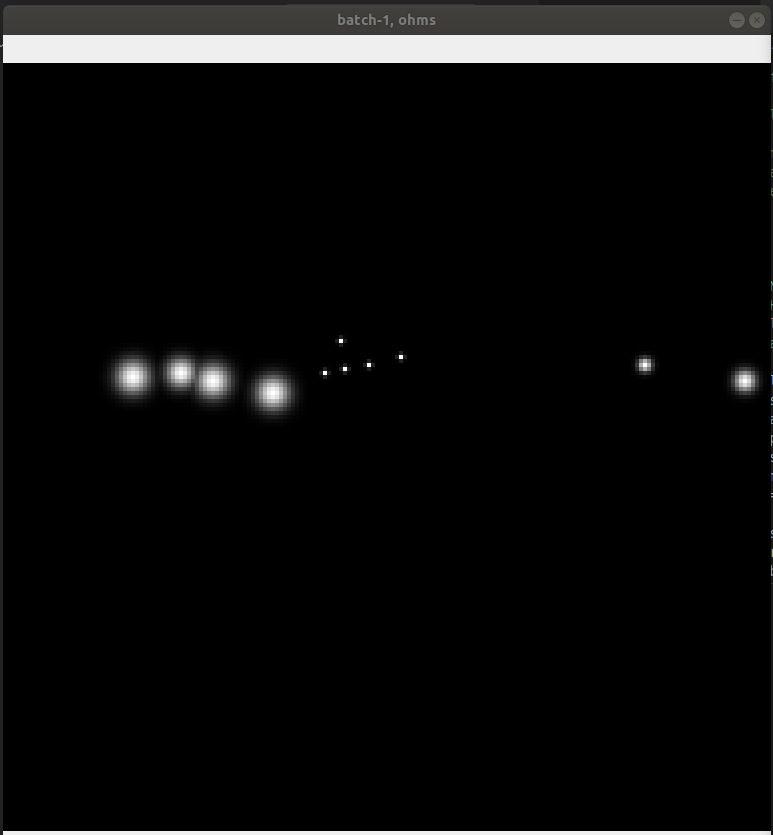
This mask for ignoring objects(drawing as circle with same radius as in heatmap generation)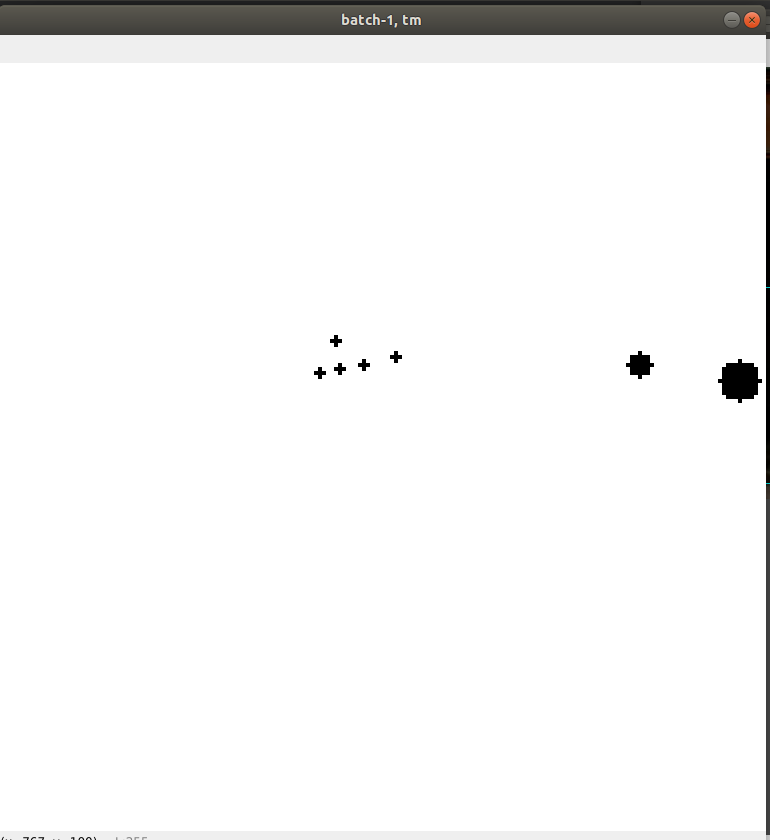
This is masked source heatmaps.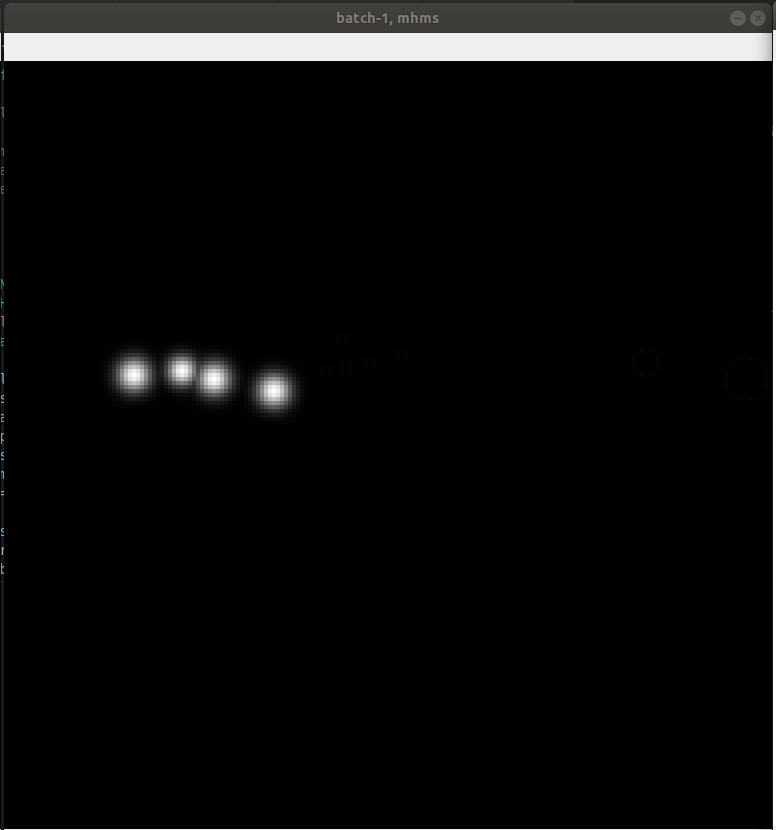
My question is: is there a proper way to generate training mask for ignoring objects?